An Advanced Hybrid Deep Adversarial Autoencoder For Parameterized Nonlinear Fluid Flow Modelling
COMPUTER METHODS IN APPLIED MECHANICS AND ENGINEERING(2020)
摘要
Considering the high computation cost required in conventional computation fluid dynamic simulations, machine learning methods have been introduced to flow dynamic simulations in years, aiming on reducing CPU time. In this work, we propose a hybrid deep adversarial autoencoder (VAE-GAN) to integrate generative adversarial network (GAN) and variational autoencoder (VAE) for predicting parameterized nonlinear fluid flows in spatial and temporal dimensions. High-dimensional inputs are compressed into the low-dimensional representations by nonlinear functions in a convolutional encoder. In this way, the predictive fluid flows reconstructed in a convolutional decoder contain the dynamic fluid flow physics of high nonlinearity and chaotic nature. In addition, the low-dimensional representations are applied to the adversarial network for model training and parameter optimization, which enables fast computation process. The capability of the hybrid VAE-GAN is illustrated by varying inputs on a flow past a cylinder test case as well as a second case of water column collapse. Numerical results show that this hybrid VAE-GAN has successfully captured the spatio-temporal flow features with CPU speed-up of three orders of magnitude. These promising results suggest that the hybrid VAE-GAN can play a critical role in efficiently and accurately predicting complex flows in future research efforts. (c) 2020 Elsevier B.V. All rights reserved.
更多查看译文
关键词
Parameterized, Nonlinear fluid flows, Generative adversarial networks, Variational autoencoder, Model reduction
AI 理解论文
溯源树
样例
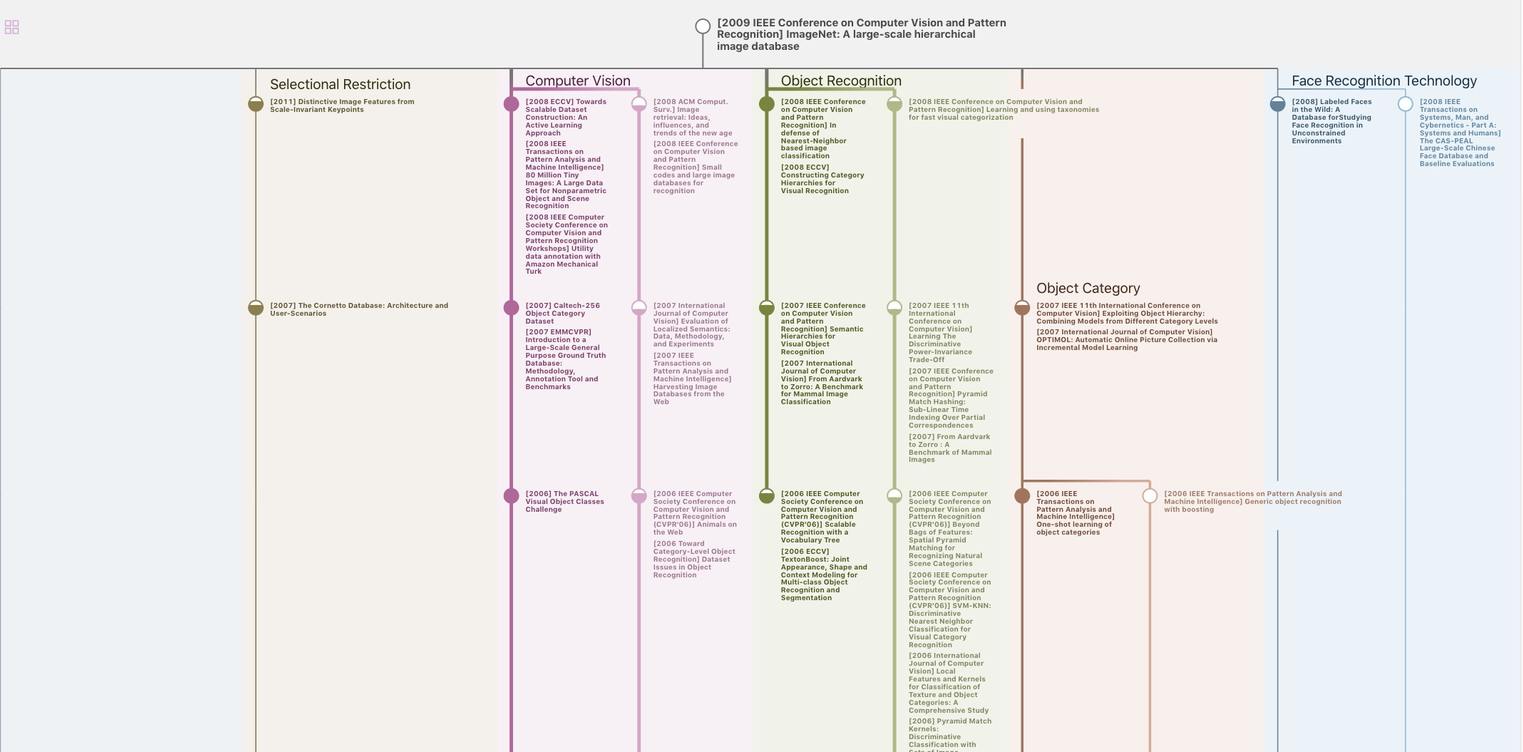
生成溯源树,研究论文发展脉络
Chat Paper
正在生成论文摘要