Deep Learning based Prediction Model for Adaptive Video Streaming
2020 International Conference on COMmunication Systems & NETworkS (COMSNETS)(2020)
摘要
Improving the overall Quality of Experience (QoE) of a client for a given video streaming session is a challenging task in a continuously varying network environment. A dynamic bit-rate adaptation strategy at the client side that can maximize the overall QoE for a session is required irrespective of the bandwidth allocation scheme adopted in-network. Even though there are a plethora of state-of-the-art strategies for adaptive bit-rate streaming, they suffer from a few key shortcomings which may significantly restrict the overall QoE potentially achievable by an end-user. For instance, QoE management using a fixed set of rules may not always guarantee optimal bandwidth utilization, video quality enhancement and accurate buffer estimation, especially in the face of severely varying and often unpredictable bandwidth fluctuations. To handle these issues across a wide range of varying network conditions and QoE parameters, machine learning strategies are being used in recent times. ML approaches train the QoE models based on diverse observations related to network bandwidth, actually received bitrate, segment size, etc, corresponding to video segments received in the past. In this work, we propose a Deep Neural Network based model that selects the appropriate video bit-rates in order to maximize the overall QoE of a user. The overall QoE is obtained as a linear combination of various input parameters such as perceived video quality, buffering time and smoothness of the video session. Experimental results reveal that the proposed architecture improves the average QoE achieved by a client by ≈8.84% when compared against Pensieve, a state-of-the-art ML based approach.
更多查看译文
关键词
Adaptive Video Streaming,DASH,LSTM,Actor-Critic Network,QoE
AI 理解论文
溯源树
样例
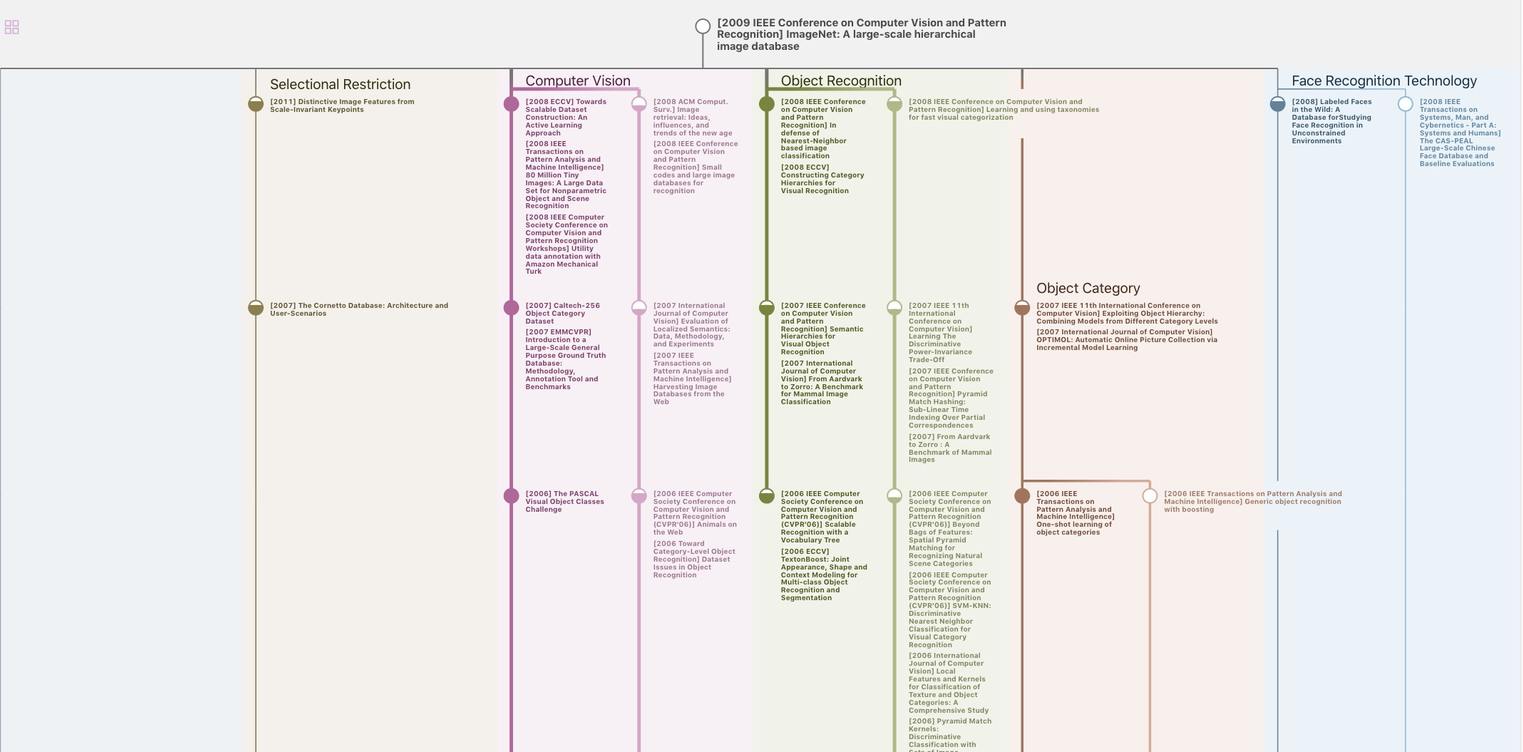
生成溯源树,研究论文发展脉络
Chat Paper
正在生成论文摘要