Intelligent Deep Learning Method for Forecasting the Health Evolution Trend of Aero-Engine With Dispersion Entropy-Based Multi-Scale Series Aggregation and LSTM Neural Network
IEEE ACCESS(2020)
摘要
Accurate health evolution trend forecasting of aero-engine is essential for operation reliability and maintenance costs of aeronautical equipment. In this study, an intelligent deep learning method, systematically blending the dispersion entropy-based multi-scale series aggregation scheme and long short term memory (LSTM) neural network, is proposed for forecasting the health evolution trend of aero-engine. Firstly, a comprehensive measurement of health levels, namely, integrated health state index (IHSI), is developed with high-dimensional dataset. Secondly, the complete ensemble empirical mode decomposition with adaptive noise (CEEMDAN) is exploited to decompose the IHSI sequence into several multi-scale series to further capture the internal characteristics of original sequence. Subsequently, multi-scale series aggregation assisted with dispersion entropy analysis theory is conducted for obtaining the aggregated sub-series (ASS). Finally, the ASS are served as the inputs of LSTM network to complete the health evolution trend forecasting of aero-engine. To demonstrate the effectiveness of the proposed method, six approaches are present for the comparisons of forecasting performance. The experimental results indicate that the proposed method can effectively measure the health evolution process of aero-engine and further obtain more accurate trend forecasting results.
更多查看译文
关键词
Aero-engine,health evolution trend forecasting,health state index,CEEMDAN,multiscale series aggregation,dispersion entropy,LSTM neural network
AI 理解论文
溯源树
样例
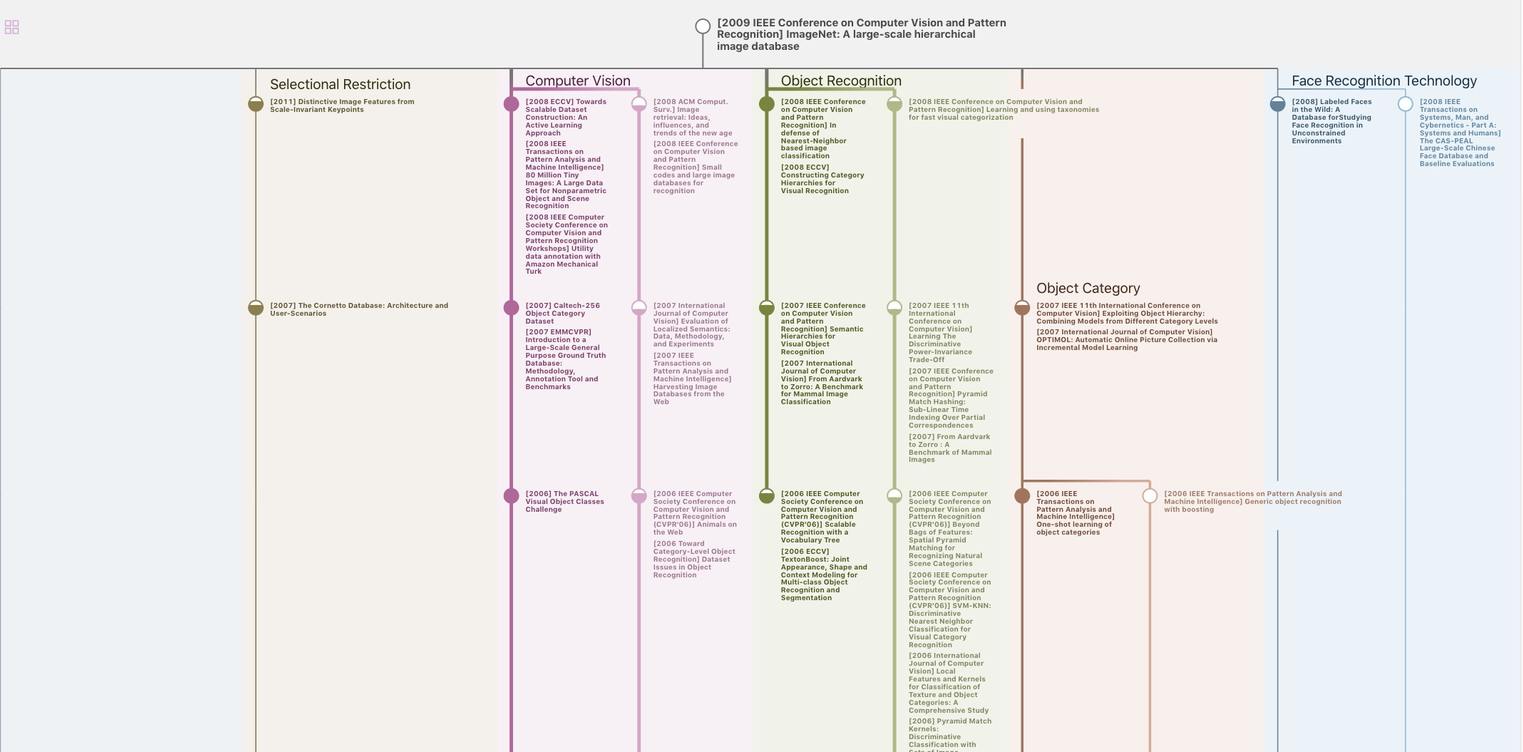
生成溯源树,研究论文发展脉络
Chat Paper
正在生成论文摘要