An integrated model for textual social media data with spatio-temporal dimensions
Information Processing & Management(2020)
摘要
GPS-enabled devices and social media popularity have created an unprecedented opportunity for researchers to collect, explore, and analyze text data with fine-grained spatial and temporal metadata. In this sense, text, time and space are different domains with their own representation scales and methods. This poses a challenge on how to detect relevant patterns that may only arise from the combination of text with spatio-temporal elements. In particular, spatio-temporal textual data representation has relied on feature embedding techniques. This can limit a model’s expressiveness for representing certain patterns extracted from the sequence structure of textual data. To deal with the aforementioned problems, we propose an Acceptor recurrent neural network model that jointly models spatio-temporal textual data. Our goal is to focus on representing the mutual influence and relationships that can exist between written language and the time-and-place where it was produced. We represent space, time, and text as tuples, and use pairs of elements to predict a third one. This results in three predictive tasks that are trained simultaneously. We conduct experiments on two social media datasets and on a crime dataset; we use Mean Reciprocal Rank as evaluation metric. Our experiments show that our model outperforms state-of-the-art methods ranging from a 5.5% to a 24.7% improvement for location and time prediction.
更多查看译文
关键词
Social media,Spatio-temporal data,Recurrent neural networks
AI 理解论文
溯源树
样例
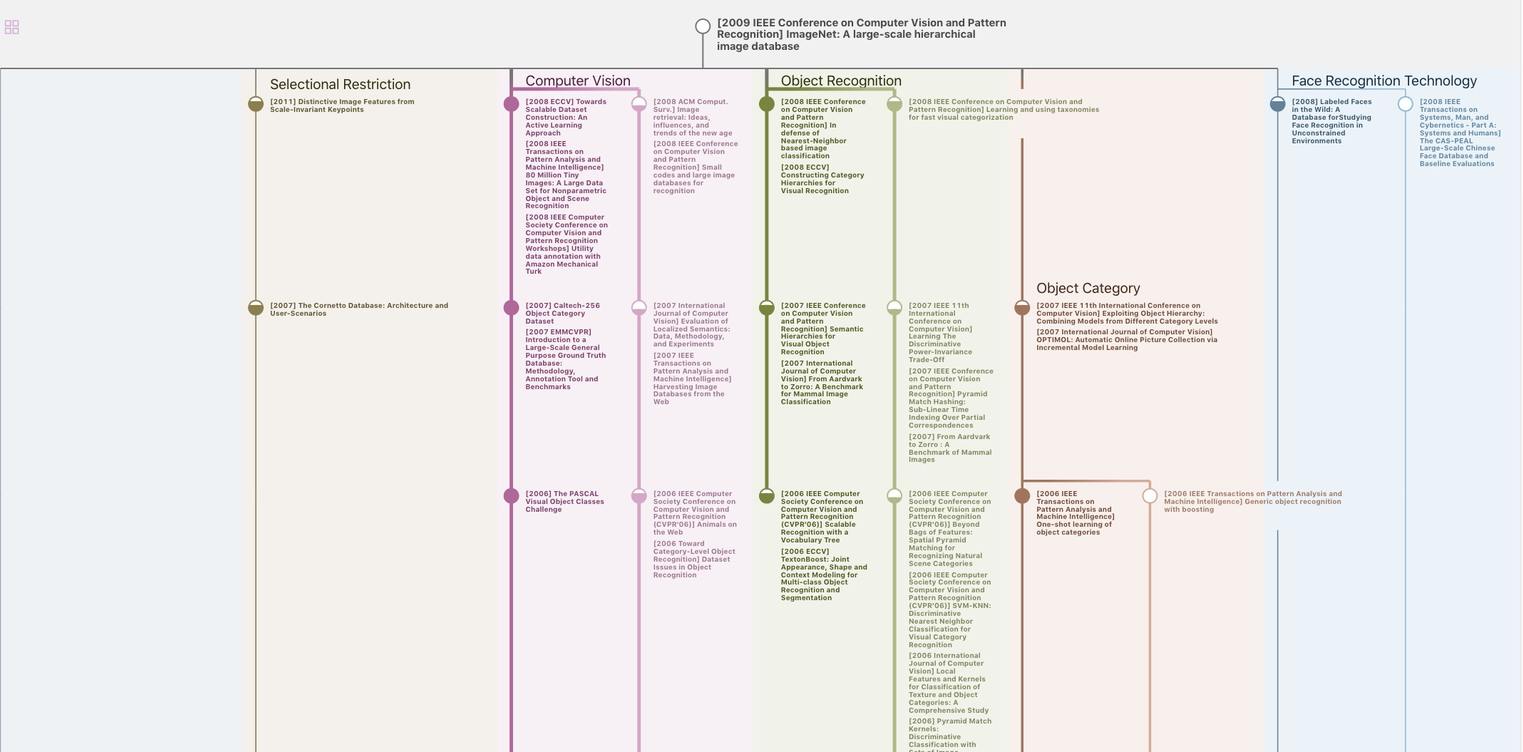
生成溯源树,研究论文发展脉络
Chat Paper
正在生成论文摘要