Soft sensor modeling for unobserved multimode nonlinear processes based on modified kernel partial least squares with latent factor clustering
IEEE ACCESS(2020)
摘要
To cope with the soft sensor modeling of unobserved multimode nonlinear processes, this paper proposes a modified kernel partial least squares (KPLS) by integrating latent factor clustering (LFC), called LFC-KPLS. In the proposed method, the process data are first divided into several batches orderly, and then projected onto the latent space by using the nonlinear functional expansion technology. In the latent space, partial least squares method is applied to compute the regression coefficients between the input variables and output variable of each batch. These regression coefficients, called the latent factors, can describe the functional relationships in the unobserved multimode data. Therefore, the latent factors are used for mode clustering so that the process data with similar functional relations can be clustered in one mode together. For each mode, the nonlinear soft sensor is established based on KPLS. To assign the mode of the online query sample, a mode identification strategy based on Bayesian inference is designed for the soft sensor online prediction. Finally, two cases studies are adopted to validate the proposed method.
更多查看译文
关键词
Soft sensor,nonlinearity,unobserved multimode,kernel partial least squares,latent factor clustering
AI 理解论文
溯源树
样例
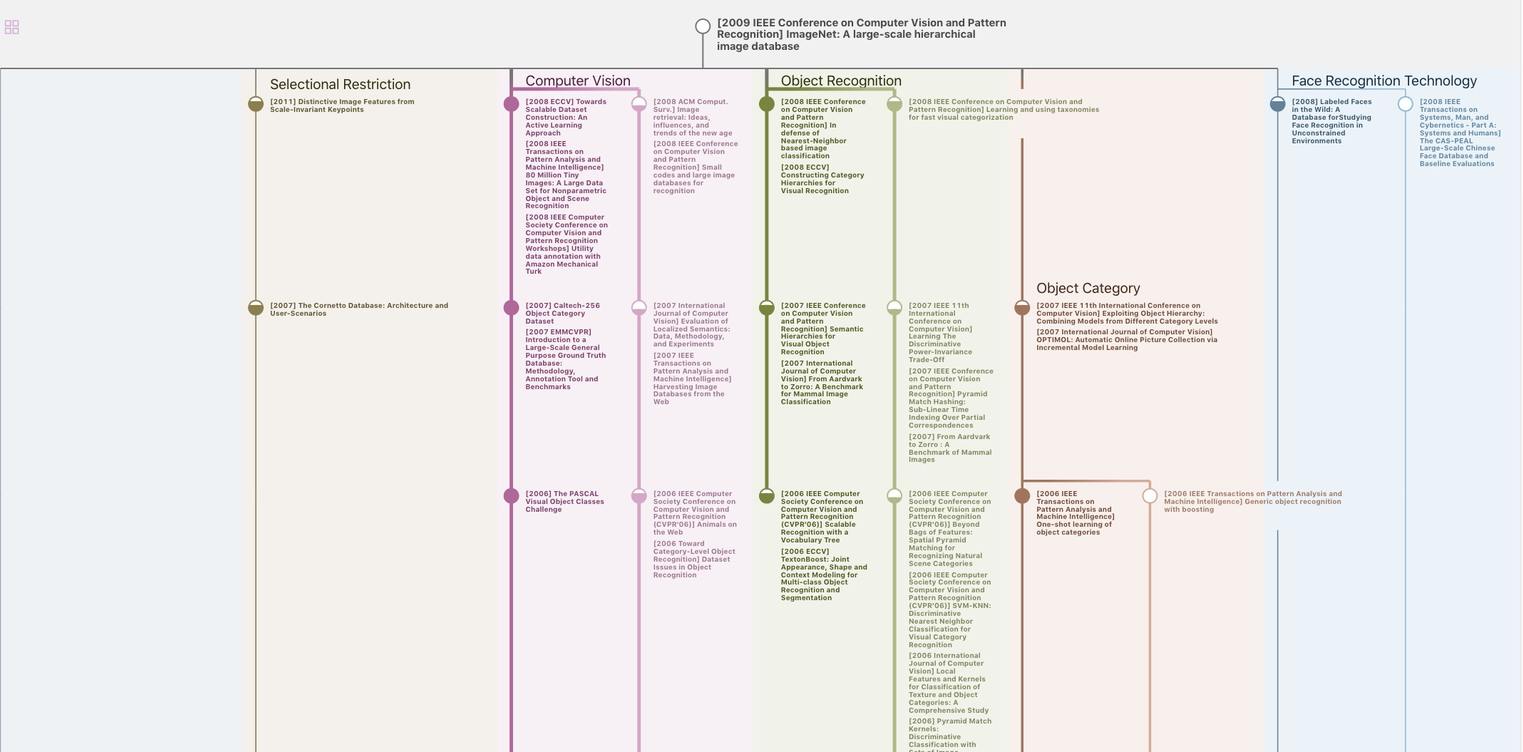
生成溯源树,研究论文发展脉络
Chat Paper
正在生成论文摘要