Defect Image Sample Generation With GAN for Improving Defect Recognition
IEEE Transactions on Automation Science and Engineering(2020)
摘要
This article aims to improve deep-learning-based surface defect recognition. Owing to the insufficiency of the defect images in practical production lines and the high cost of labeling, it is difficult to obtain a sufficient defect data set in terms of diversity and quantity. A new generation method called surface defect-generation adversarial network (SDGAN), which employs generative adversarial networks (GANs), is proposed to generate defect images using a large number of defect-free images from industrial sites. Experiments show that the defect images generated by the SDGAN have better image quality and diversity than those generated by the state-of-the-art methods. The SDGAN is applied to expand the commutator cylinder surface defect image data sets with and without labels (referred to as the CCSD-L and CCSD-NL data sets, respectively). Regarding anomaly recognition, a 1.77% error rate and a 49.43% relative improvement (IMP) for the CCSD-NL defect data set are obtained. Regarding defect classification, a 0.74% error rate and a 57.47% IMP for the CCSD-L defect data set are achieved. Moreover, defect classification trained on the images augmented by the SDGAN is robust to uneven and poor lighting conditions.
Note to Practitioners
—This article proposes a method of defect image generation to address the lack of industrial defect images. Traditional defect recognition methods have two disadvantages: different types of defects require different algorithms and handcrafted features are deficient. Defect recognition using deep learning can solve the above problems. However, deep learning requires a plethora of images, and the number of industrial defect images cannot meet this requirement. We propose a new defect image-generation method called SDGAN to generate a defect image data set that balances diversity and authenticity. In practice, we employ a large number of defect-free images to generate a large number of defect images using our method to expand the industry defect-free image data set. Then, the augmented defect data set is used to build a deep-learning defect recognition model. Experiments show that the accuracy of defect recognition can be significantly improved by building a deep-learning defect recognition model using the augmented data set. Therefore, deep learning can achieve excellent performance in defect recognition with a limited number of defect images.
更多查看译文
关键词
Gallium nitride,Machine learning,Image generation,Image recognition,Feature extraction,Data models,Surface treatment
AI 理解论文
溯源树
样例
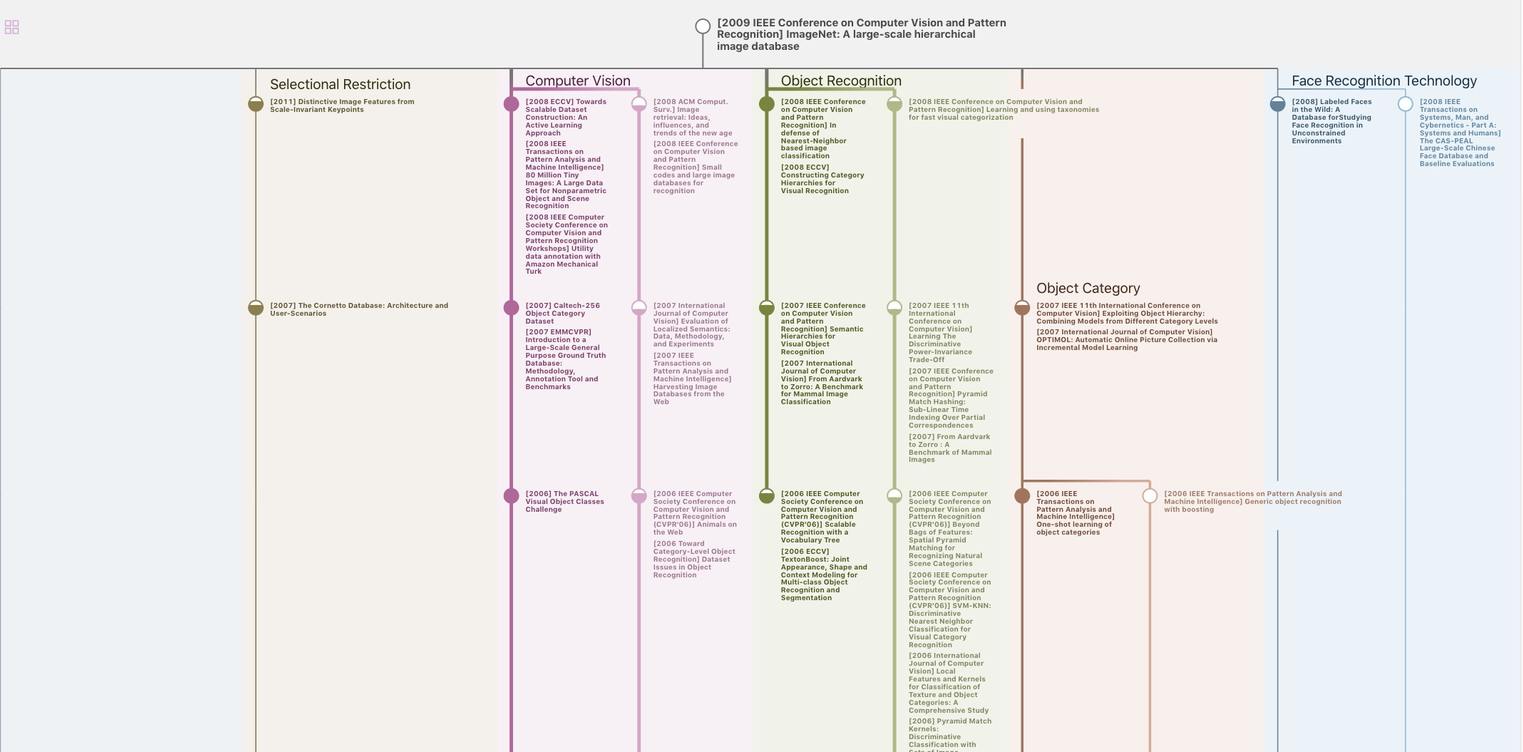
生成溯源树,研究论文发展脉络
Chat Paper
正在生成论文摘要