Two-level attention and score consistency network for plant segmentation
Computers and Electronics in Agriculture(2020)
摘要
Semantic segmentation of plant images can provide valuable information for plant phenotypic studies. However, challenges exist when facing complex background interference and uneven illumination. In this study, we propose a Two-level Attention and Score Consistency based Network (TASCN) for semantic segmentation of plant. Our TASCN includes a two-level attention sub-module (TAM) and a multi-scale feature fusion sub-module (FFM). The TAM combines a top-down semantic attention gate (Inter-layer attention, level 1) and a self-attention mechanism (Intra-layer attention, level 2) to highlight the salient features and suppress the irrelevant or interference information. The FFM fuses the multi-scale features from the TAM to obtain the final segmentation score-map. The score-map is further restricted with the score consistency loss to promote the semantic consistency and reduces the impact of uneven illumination. Experiments on rice dataset from the Chinese spacelab TG-2 demonstrate the impressing segmentation performance of our TASCN, especially for complex background interference and uneven illumination. In addition, both the quantitative evaluation (Mean IoU) and qualitative visual results show that our TASCN outperforms the state-of-the-art methods, including FCN, U-Net, SegNet, PSPNet, DeepLabv3+ and DANet. In conclusion, a novel TASCN is proposed to meet the increasing need for fine plant parsing in plant research.
更多查看译文
关键词
Attention mechanism,Score consistency,Plant image analysis,Semantic segmentation
AI 理解论文
溯源树
样例
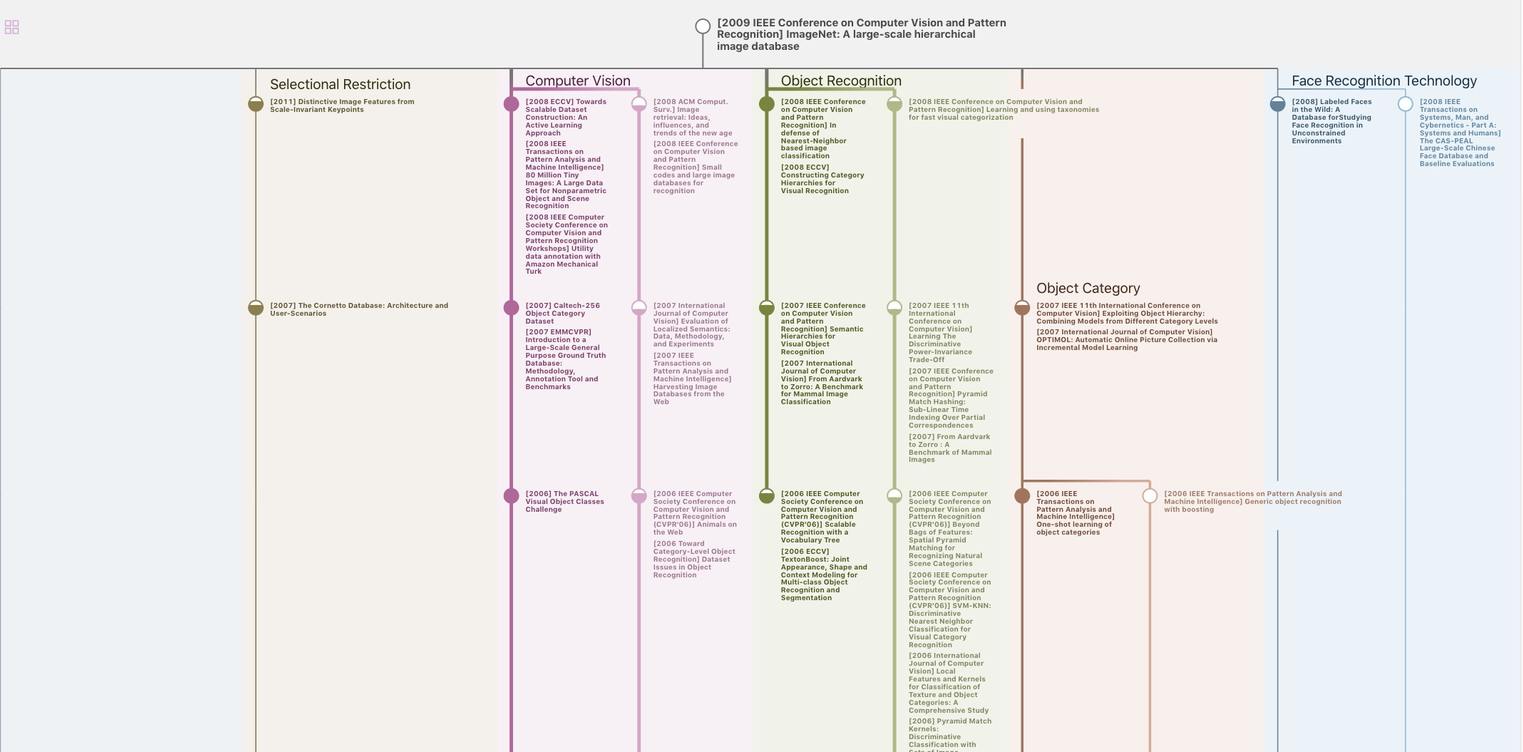
生成溯源树,研究论文发展脉络
Chat Paper
正在生成论文摘要