Accelerated deep reinforcement learning with efficient demonstration utilization techniques
WORLD WIDE WEB-INTERNET AND WEB INFORMATION SYSTEMS(2020)
摘要
The use of demonstrations for deep reinforcement learning (RL) agents usually accelerates their training process as well as guides the agents to learn complicated policies. Most of the current deep RL approaches with demonstrations assume that there is a sufficient amount of high-quality demonstrations. However, for most real-world learning cases, the available demonstrations are often limited in terms of amount and quality. In this paper, we present an accelerated deep RL approach with dual replay buffer management and dynamic frame skipping on demonstrations. The dual replay buffer manager manages a human replay buffer and an actor replay buffer with independent sampling policies. We also propose dynamic frame skipping on demonstrations called DFS-ER (Dynamic Frame Skipping-Experience Replay) that learns the action repetition factor of the demonstrations. By implementing DFS-ER, we can accelerate deep RL by improving the efficiency of demonstration utilization, thereby yielding a faster exploration of the environment. We verified the training acceleration in three dense reward environments and one sparse reward environment compared to the conventional approach. In our evaluation using the Atari game environments, the proposed approach showed 21.7%-39.1% reduction in training iterations in a sparse reward environment.
更多查看译文
关键词
Deep reinforcement learning, Dynamic frame skipping, Experience replay, Imitation learning
AI 理解论文
溯源树
样例
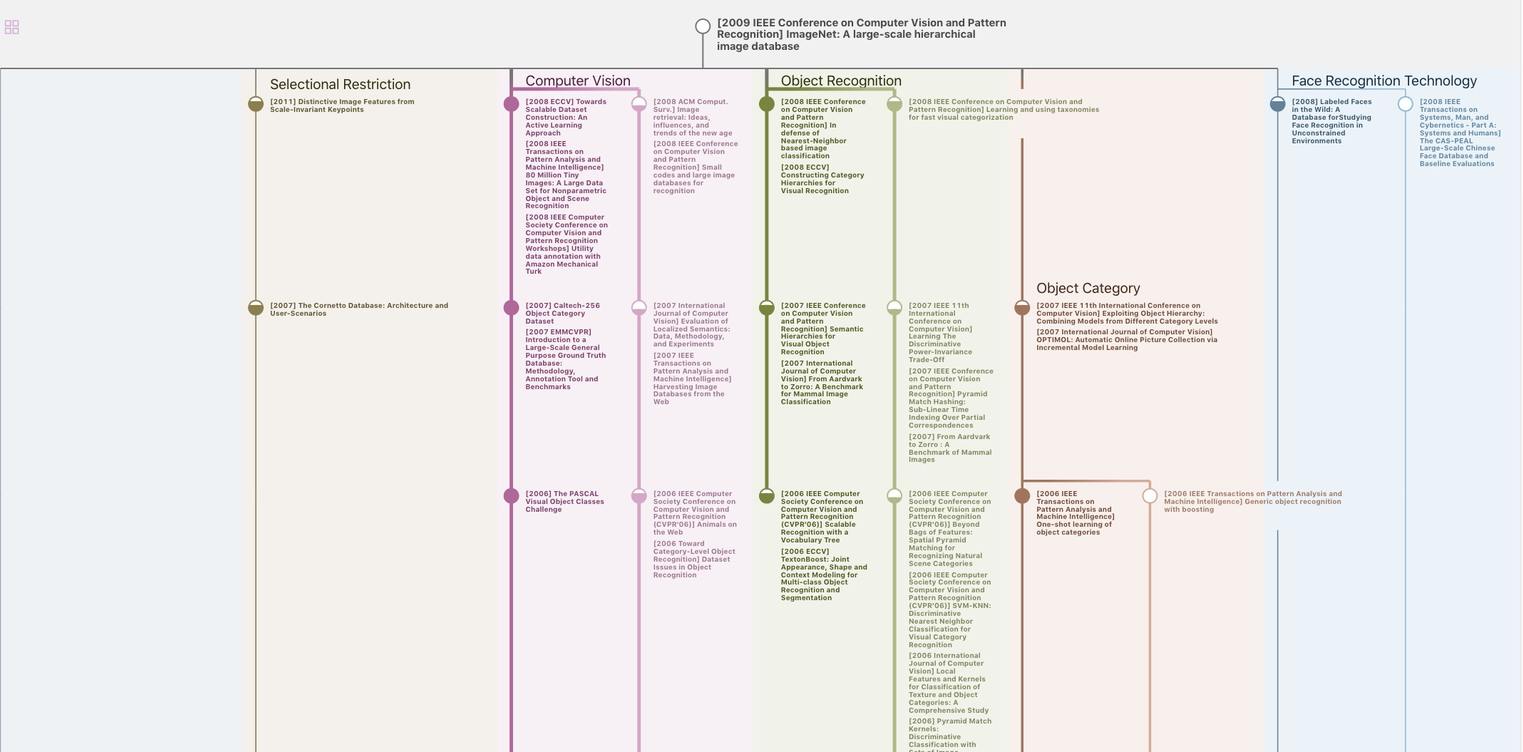
生成溯源树,研究论文发展脉络
Chat Paper
正在生成论文摘要