Multiobjective Particle Swarm Optimization Based on Network Embedding for Complex Network Community Detection
IEEE Transactions on Computational Social Systems(2020)
摘要
Community detection in complex networks is significant to social network analysis. Most of the algorithms take advantage of single-objective optimization methods, which may not be effective for complex networks. Compared with single-objective algorithms, multiobjective evolutionary algorithms can avoid local optimization. However, multiobjective evolutionary algorithms often encounter problems of excessive search space and low efficiency. To solve these issues, this study introduces network embedding into the multiobjective particle swarm algorithm and maps nodes into a low-latitude space, thereby effectively reducing the search space while increasing search efficiency via a consensus propagation strategy. Experimental results demonstrate that a novel effective algorithm based on multiobjective particle swarm optimization (NE-PSO) performs effectively and has competitive performance in comparison with state-of-the-art approaches on synthetic and real-world networks, especially the large-scale ones.
更多查看译文
关键词
Complex networks,Optimization,Particle swarm optimization,Genetic algorithms,Task analysis,Clustering algorithms
AI 理解论文
溯源树
样例
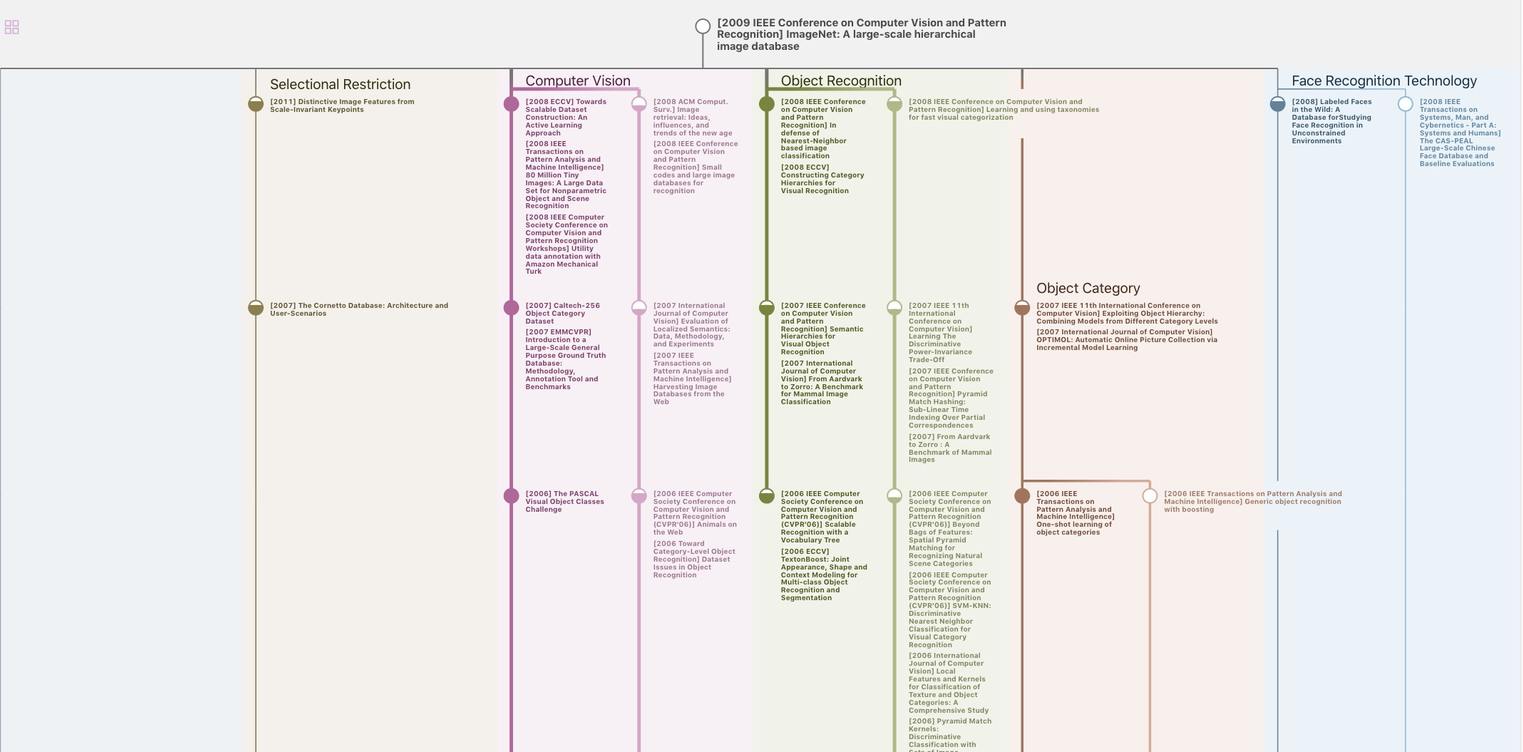
生成溯源树,研究论文发展脉络
Chat Paper
正在生成论文摘要