Discrepancy-Based Theory and Algorithms for Forecasting Non-Stationary Time Series
Annals of Mathematics and Artificial Intelligence(2020)
摘要
We present data-dependent learning bounds for the general scenario of non-stationary non-mixing stochastic processes. Our learning guarantees are expressed in terms of a data-dependent measure of sequential complexity and a discrepancy measure that can be estimated from data under some mild assumptions. Our learning bounds guide the design of new algorithms for non-stationary time series forecasting for which we report several favorable experimental results.
更多查看译文
关键词
Time series, Forecasting, Non-stationary, Non-mixing, Generalization bounds, Discrepancy, Expected sequential covering numbers, Sequential Rademacher complexity, 68T01
AI 理解论文
溯源树
样例
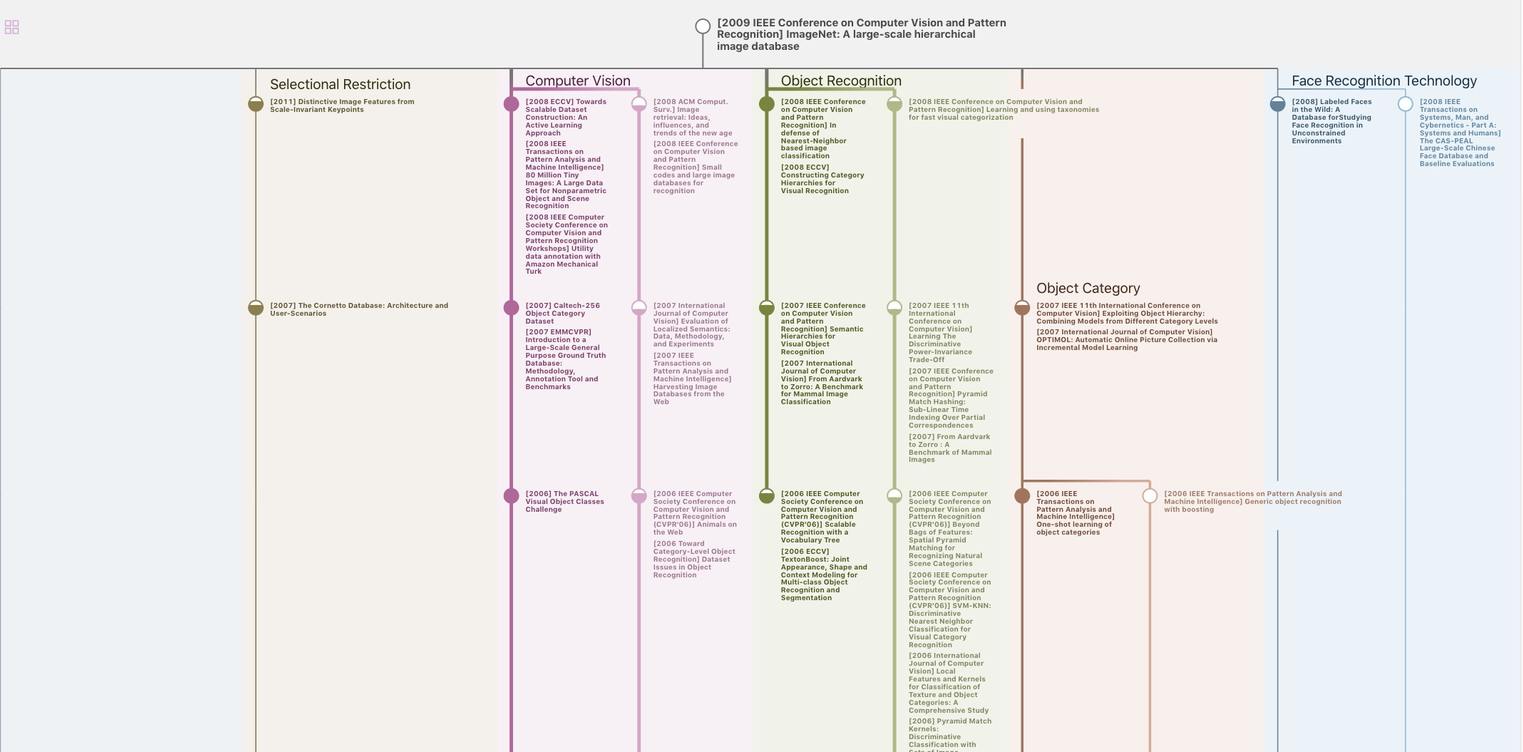
生成溯源树,研究论文发展脉络
Chat Paper
正在生成论文摘要