Structured Domain Adaptation With Online Relation Regularization for Unsupervised Person Re-ID
IEEE TRANSACTIONS ON NEURAL NETWORKS AND LEARNING SYSTEMS(2024)
摘要
Unsupervised domain adaptation (UDA) aims at adapting the model trained on a labeled source-domain dataset to an unlabeled target-domain dataset. The task of UDA on open-set person reidentification (re-ID) is even more challenging as the identities (classes) do not have overlap between the two domains. One major research direction was based on domain translation, which, however, has fallen out of favor in recent years due to inferior performance compared with pseudo-label-based methods. We argue that domain translation has great potential on exploiting valuable source-domain data but the existing methods did not provide proper regularization on the translation process. Specifically, previous methods only focus on maintaining the identities of the translated images while ignoring the intersample relations during translation. To tackle the challenges, we propose an end-to-end structured domain adaptation framework with an online relation-consistency regularization term. During training, the person feature encoder is optimized to model intersample relations on-the-fly for supervising relation-consistency domain translation, which in turn improves the encoder with informative translated images. The encoder can be further improved with pseudo labels, where the source-to-target translated images with ground-truth identities and target-domain images with pseudo identities are jointly used for training. In the experiments, our proposed framework is shown to achieve state-of-the-art performance on multiple UDA tasks of person re-ID. With the synthetic -> real translated images from our structured domain-translation network, we achieved second place in the Visual Domain Adaptation Challenge (VisDA) in 2020.
更多查看译文
关键词
Training,Image color analysis,Learning systems,Germanium,Adaptation models,Visualization,Task analysis,Domain translation,person reidentification (re-ID),relation consistency,unsupervised domain adaptation (UDA)
AI 理解论文
溯源树
样例
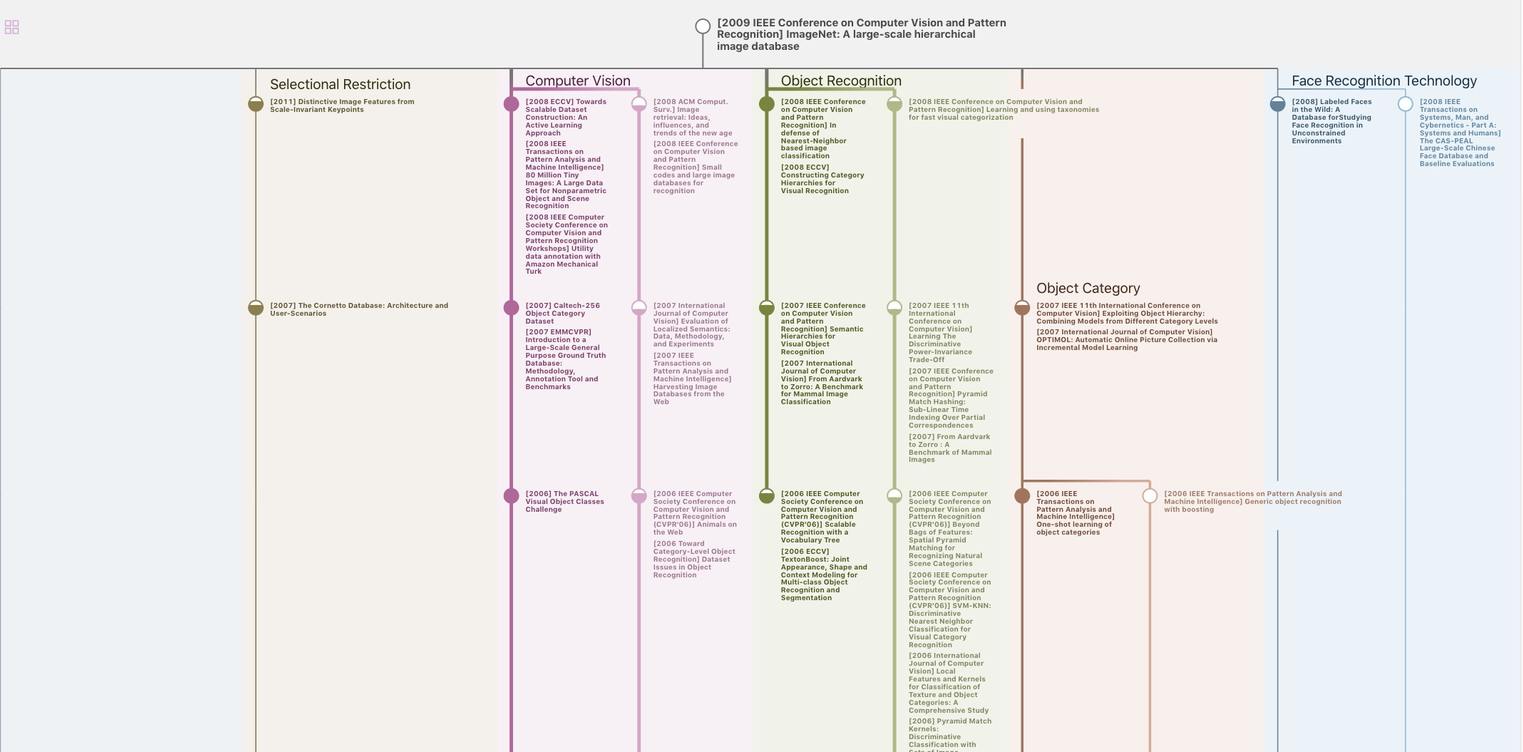
生成溯源树,研究论文发展脉络
Chat Paper
正在生成论文摘要