Robust Speech Recognition based on Multi-Objective Learning with GRU Network
2019 Asia-Pacific Signal and Information Processing Association Annual Summit and Conference (APSIPA ASC)(2019)
摘要
This paper proposes a new scheme to execute the task of speech enhancement (SE) for recognition based on multi-objective learning method which uses three objectives in the gated recurrent unit (GRU) network training procedure. The first objective is the main target for the expected SE task by directly mapping the noisy log-power spectrum (LPS) features to clean Mel-frequency cepstral coefficients (MFCC) features. The second one is an auxiliary target to help improving the main one by learning additional information from the backend acoustic model (AM). The third one is also an auxiliary target achieved by learning some information from mapping noisy LPS to clean LPS. The two auxiliary structures could help the original structure to optimize the network parameters by correcting the errors. This approach imposes more constraints on direct feature mapping and information passing from the acoustic model to the network, enabling the enhanced network to better serve the AM. The experimental results show that the new multi-objective scheme with joint feature mapping and the posterior probability learning method improves the performance of SE. And this scheme significantly lowers the Character Error Rate (CER) of the AM compared to the baseline deep neural network (DNN) network.
更多查看译文
关键词
gated recurrent unit network training procedure,expected SE task,noisy log-power spectrum,Mel-frequency cepstral coefficient features,auxiliary target,backend acoustic model,mapping noisy LPS,auxiliary structures,network parameter optimization,direct feature mapping,enhanced network,multiobjective scheme,joint feature mapping,baseline deep neural network network,robust speech recognition,GRU network,speech enhancement,multiobjective learning method,posterior probability learning method,character error rate,DNN network,MFCC features,error correction
AI 理解论文
溯源树
样例
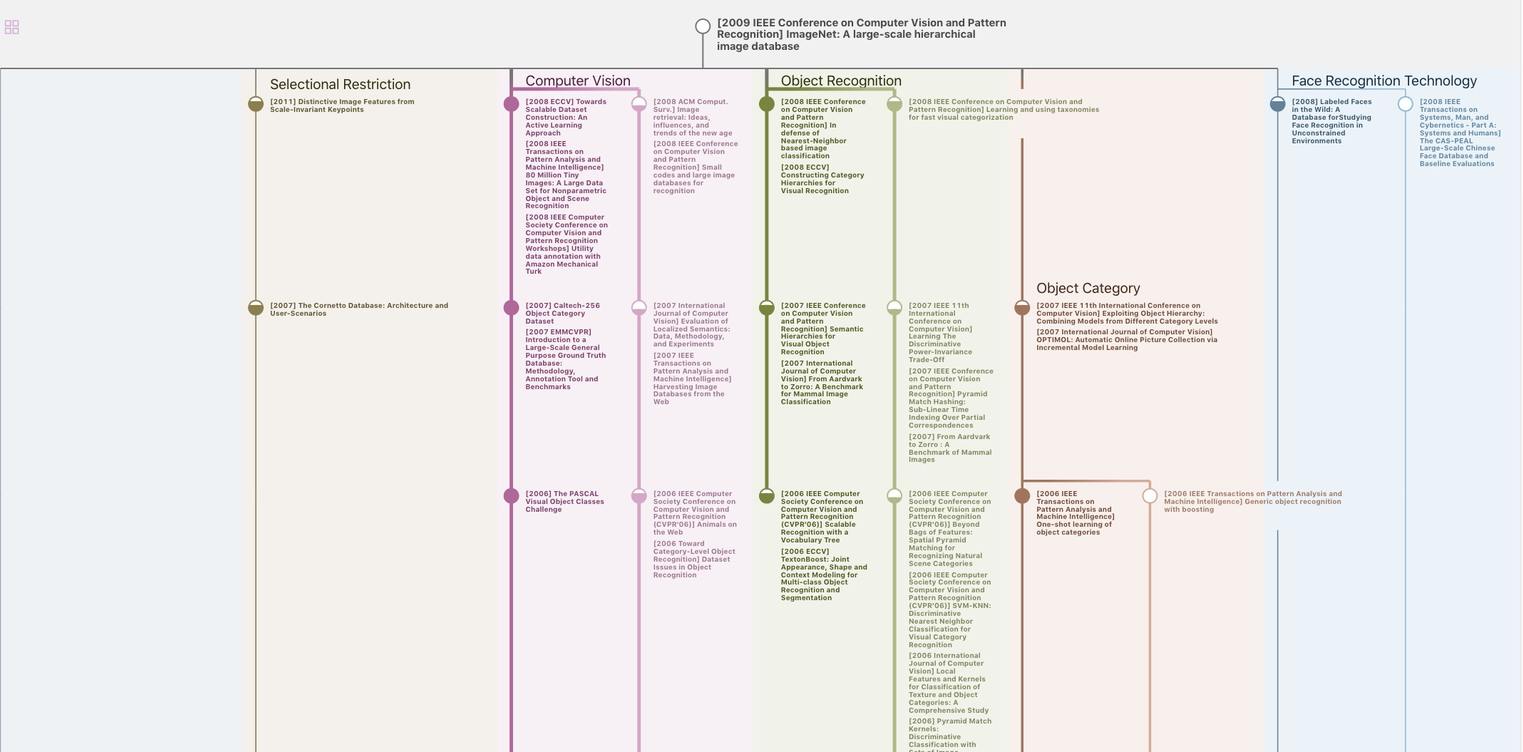
生成溯源树,研究论文发展脉络
Chat Paper
正在生成论文摘要