CPS: Class-level 6D Pose and Shape Estimation From Monocular Images
arxiv(2020)
摘要
Contemporary monocular 6D pose estimation methods can only cope with a handful of object instances. This naturally limits possible applications as, for instance, robots need to work with hundreds of different objects in a real environment. In this paper, we propose the first deep learning approach for class-wise monocular 6D pose estimation, coupled with metric shape retrieval. We propose a new loss formulation which directly optimizes over all parameters, i.e. 3D orientation, translation, scale and shape at the same time. Instead of decoupling each parameter, we transform the regressed shape, in the form of a point cloud, to 3D and directly measure its metric misalignment. We experimentally demonstrate that we can retrieve precise metric point clouds from a single image, which can also be further processed for e.g. subsequent rendering. Moreover, we show that our new 3D point cloud loss outperforms all baselines and gives overall good results despite the inherent ambiguity due to monocular data.
更多查看译文
关键词
monocular images,shape estimation,class-level
AI 理解论文
溯源树
样例
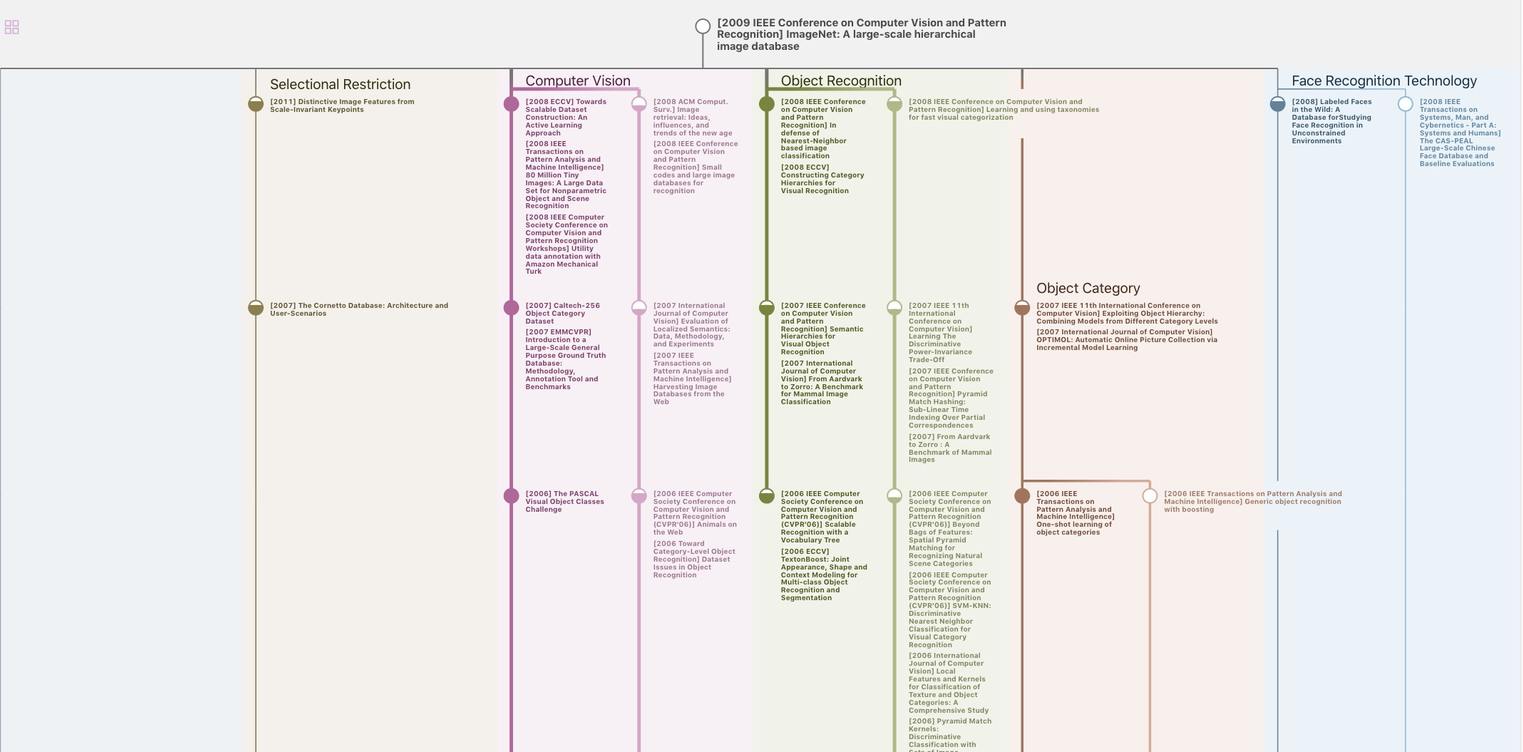
生成溯源树,研究论文发展脉络
Chat Paper
正在生成论文摘要