Online Estimation Of Mggd : The Riemannian Averaged Natural Gradient Method
CAMSAP(2019)
摘要
Multivariate Generalized Gaussian Distributions (MGGD) are a rich class of multivariate distributions, which have gained importance across many engineering applications (image processing, computer vision, radar and biomedical signal processing). Unfortunately, estimating the parameters of MGGD leads to non-linear matrix equations, whose solution becomes unpractical in high-dimensional problems, or when dealing with very large datasets. To overcome this difficulty, the present paper proposes a new method for online estimation of MGGD parameters, called the Riemannian Averaged Natural Gradient (RANG) method. The RANG method is suitable for application with high-dimensional and large datasets, since it requires modest memory and computational resources. The present paper formulates this new method, and presents some computer simulations, to showcase its performance. It is seen that, while the RANG method makes less exhaustive use of available data, it still achieves identical performance, to classical maximum-likelihood estimation, for sufficiently large datasets.
更多查看译文
关键词
nonlinear matrix equations,biomedical signal processing,computer vision,image processing,engineering applications,multivariate generalized Gaussian distributions,classical maximum-likelihood estimation,computer simulations,computational resources,RANG method,Riemannian Averaged Natural Gradient method,MGGD,online estimation,high-dimensional problems
AI 理解论文
溯源树
样例
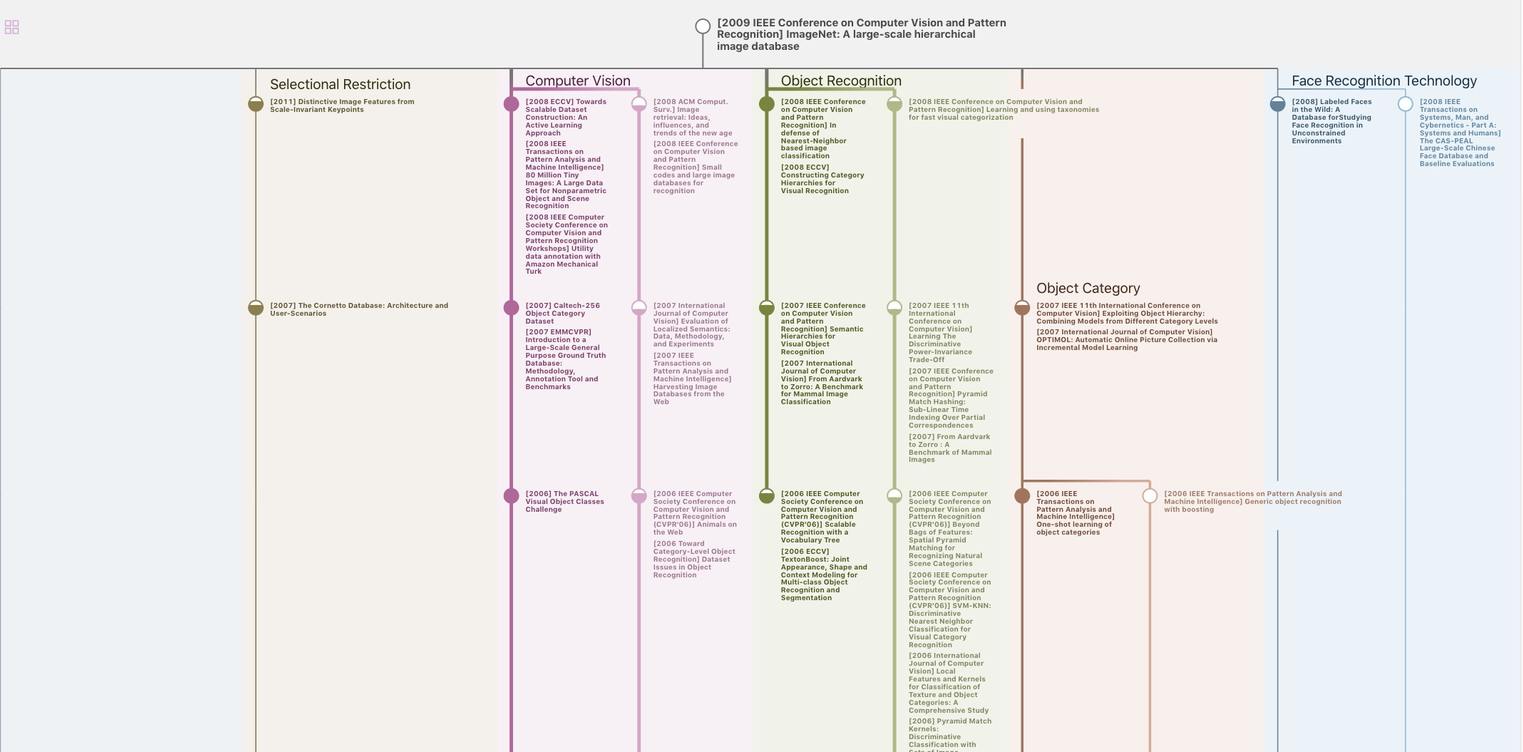
生成溯源树,研究论文发展脉络
Chat Paper
正在生成论文摘要