Vroom!: A Search Engine for Sounds by Vocal Imitation Queries
CHIIR '20: Conference on Human Information Interaction and Retrieval Vancouver BC Canada March, 2020(2020)
摘要
Traditional search through collections of audio recordings compares a text-based query to text metadata associated with each audio file and does not address the actual content of the audio. Text descriptions do not describe all aspects of the audio content in detail. Query by vocal imitation (QBV) is a kind of query by example that lets users imitate the content of the audio they seek, providing an alternative search method to traditional text search. Prior work proposed several neural networks, such as TL-IMINET, for QBV, however, previous systems have not been deployed in an actual search engine nor evaluated by real users. We have developed a state-of-the-art QBV system (Vroom!) and a baseline query-by-text search engine (TextSearch). We deployed both systems in an experimental framework to perform user experiments with Amazon Mechanical Turk (AMT) workers. Results showed that Vroom! received significantly higher search satisfaction ratings than TextSearch did for sound categories that were difficult for subjects to describe by text. Results also showed a better overall ease-of-use rating for Vroom! than TextSearch on the sound library used in our experiments. These findings suggest that QBV, as a complimentary search approach to existing text-based search, can improve both search results and user experience.
更多查看译文
关键词
vocal imitation, sound search, subjective evaluation, Siamese style convolutional recurrent neural networks, text description
AI 理解论文
溯源树
样例
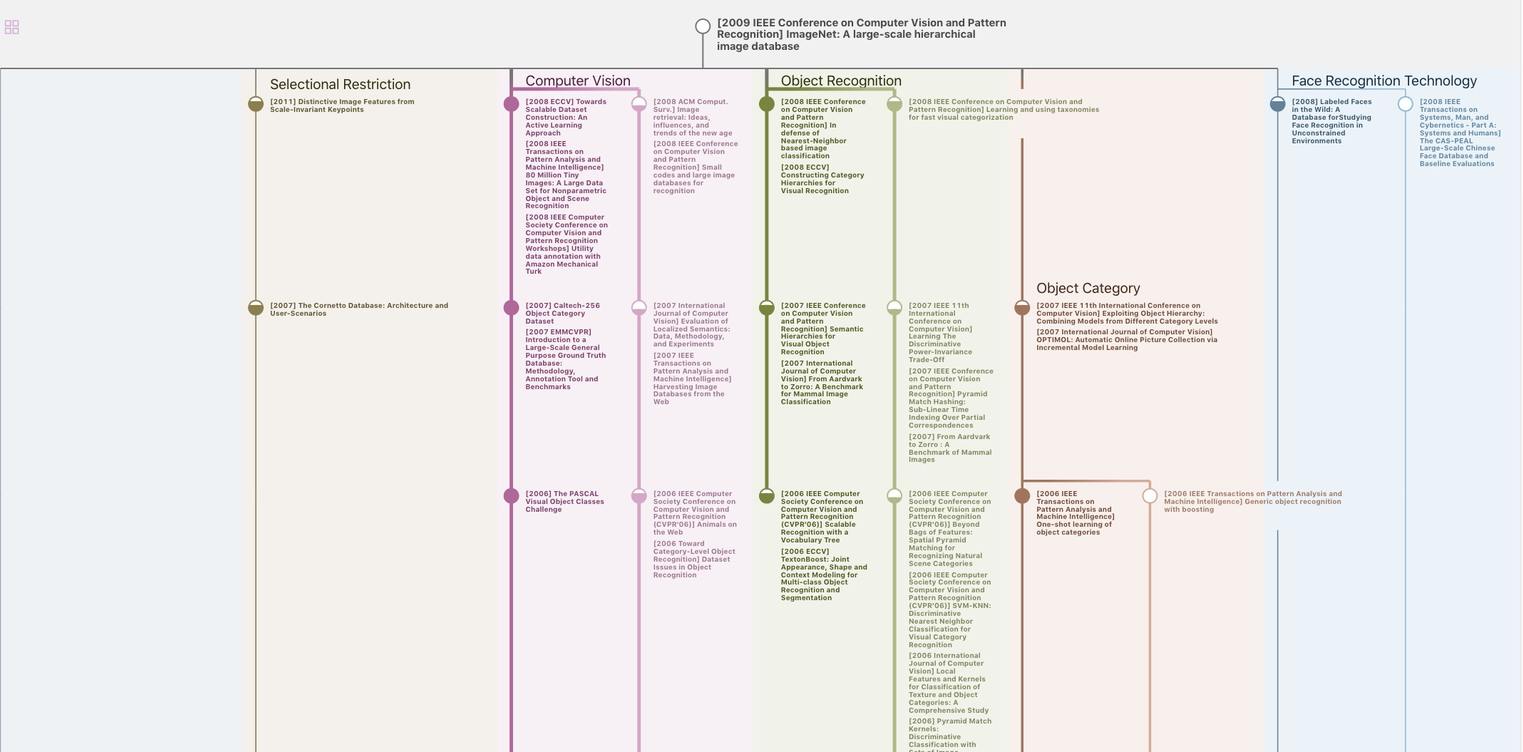
生成溯源树,研究论文发展脉络
Chat Paper
正在生成论文摘要