Deep Heterogeneous Social Network Alignment
2019 IEEE First International Conference on Cognitive Machine Intelligence (CogMI)(2019)
摘要
The online social network alignment problem aims at inferring the anchor links connecting the shared users across social networks, which are usually subject to the one-to-one cardinality constraint. Several existing social network alignment models have been proposed, many of which are based on the supervised learning setting. Given a set of labeled anchor links, a group of features can be extracted manually for the anchor links to build these models. Meanwhile, such methods may encounter great challenges in the application on real-world social network datasets, since manual feature extraction can be extremely expensive and tedious for the social networks involving heterogeneous information. In this paper, we propose to address the heterogeneous social network alignment problem with a deep learning model, namely DETA (Deep nETwork Alignment). Besides a small number of explicit features, DETA can automatically learn a set of latent features from the heterogeneous information. DETA models the anchor link one-to-one cardinality constraint as a mathematical constraint on the node degrees. Extensive experiments have been done on real-world aligned heterogeneous social network datasets, and the experimental results have demonstrated the effectiveness of the proposed model compared against the existing state-of-the-art baseline methods.
更多查看译文
关键词
Social-Network-Alignment-Information-Fusion-Deep-Learning-Data-Mining
AI 理解论文
溯源树
样例
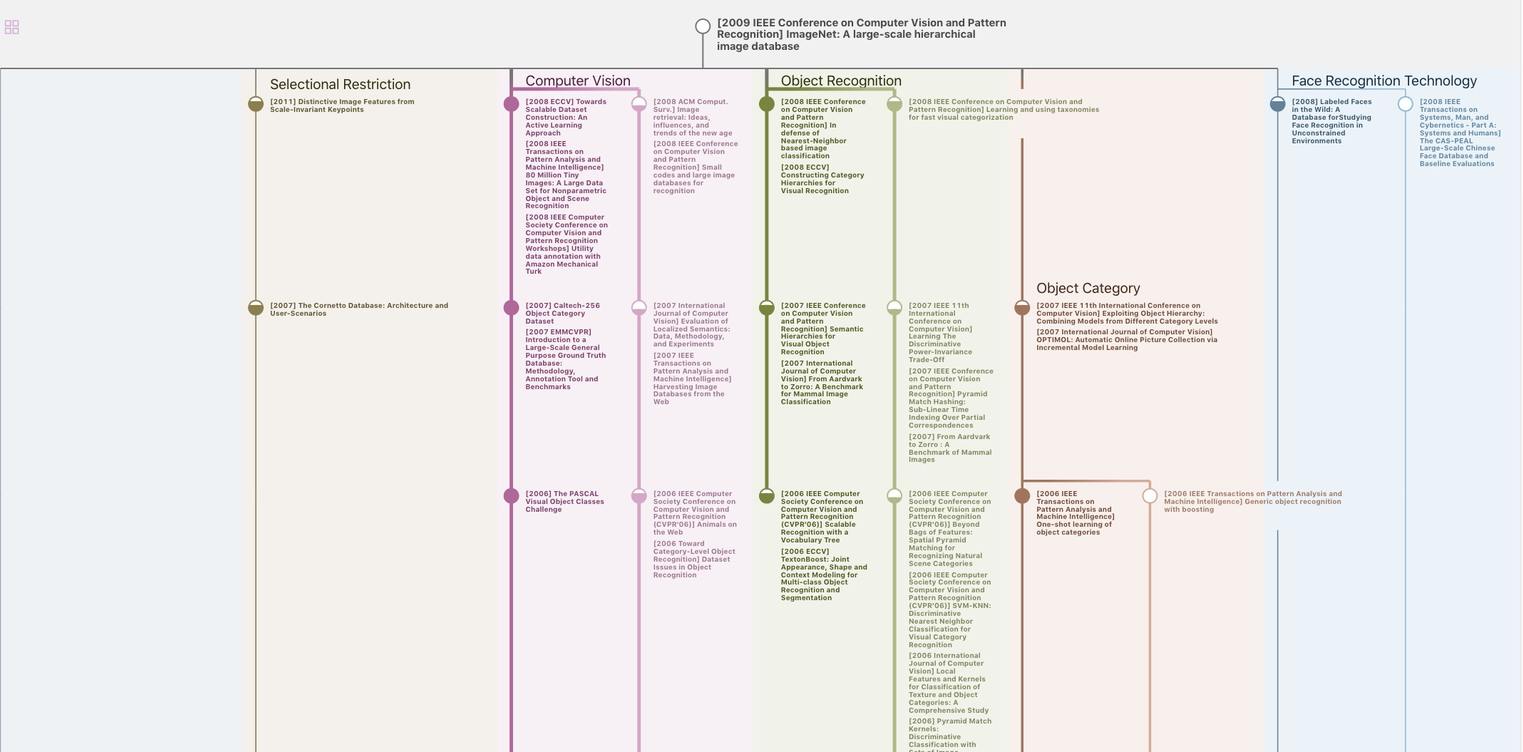
生成溯源树,研究论文发展脉络
Chat Paper
正在生成论文摘要