Predicting Indoor Crowd Density using Column-Structured Deep Neural Network.
PredictGIS@SIGSPATIAL(2017)
摘要
This work proposes a deep neural network approach known as the column-structured deep neural network (COL-DNN-R) for predicting crowd density in an indoor environment using historical Wi-Fi traces of individual visitors. With a structure designed to minimize feature engineering, COL-DNN accepts raw features such as crowd density, opening and closing hours and peak visitor counts for extracting features. The extracted features are used by a regression model R for predicting the crowd densities. Standard regression models such as MLP, RF and SVM can be used as R. Experiments are performed to investigate the effect of feature representation and model structure on the prediction accuracy. Experiment results show the best prediction accuracy is obtained using features extracted by COL-DNN and using MLP as the regression model, i.e., R = MLP.
更多查看译文
关键词
Indoor Crowd Prediction, Deep Neural Network, Feature Extraction
AI 理解论文
溯源树
样例
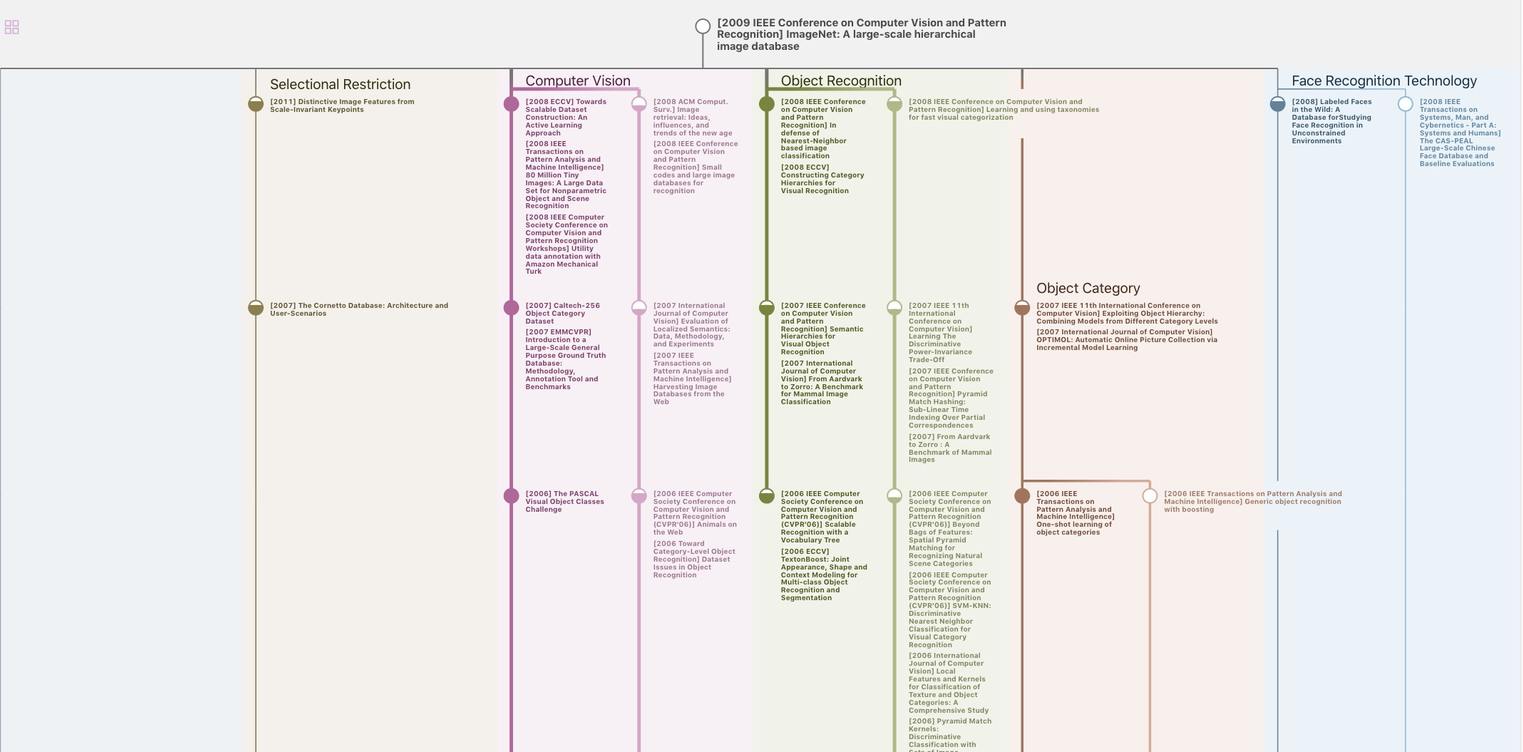
生成溯源树,研究论文发展脉络
Chat Paper
正在生成论文摘要