Time Series-Based GHG Emissions Prediction for Smart Homes
IEEE Transactions on Sustainable Computing(2020)
摘要
Smart homes play a crucial role in reducing the residential sector electricity consumption and Greenhouse Gases (GHG) emissions. In this work, we present a time series approach to predict GHG emissions to be integrated into smart home management systems. More specifically, we used Long Short-Term Memory (LSTM), a variant of Recurrent Neural Networks. The prediction results get mean absolute percentage error (MAPE) close to 2 percent when the region under study has an energy matrix mostly based on fossil fuels, less intermittent. For regions in which more renewable sources are present, the MAPE is around 12 percent. However, in either case, LSTM can predict the hours well with smaller emissions among the next 24 hours. Such day-ahead information brings awareness to the users and allows the scheduling of appliances to work in the hours in which the emissions are minimal, reducing them without significantly affecting the consumers’ behavior.
更多查看译文
关键词
Smart homes,Time series analysis,Pricing,Load modeling,Autoregressive processes,Energy management,Home appliances
AI 理解论文
溯源树
样例
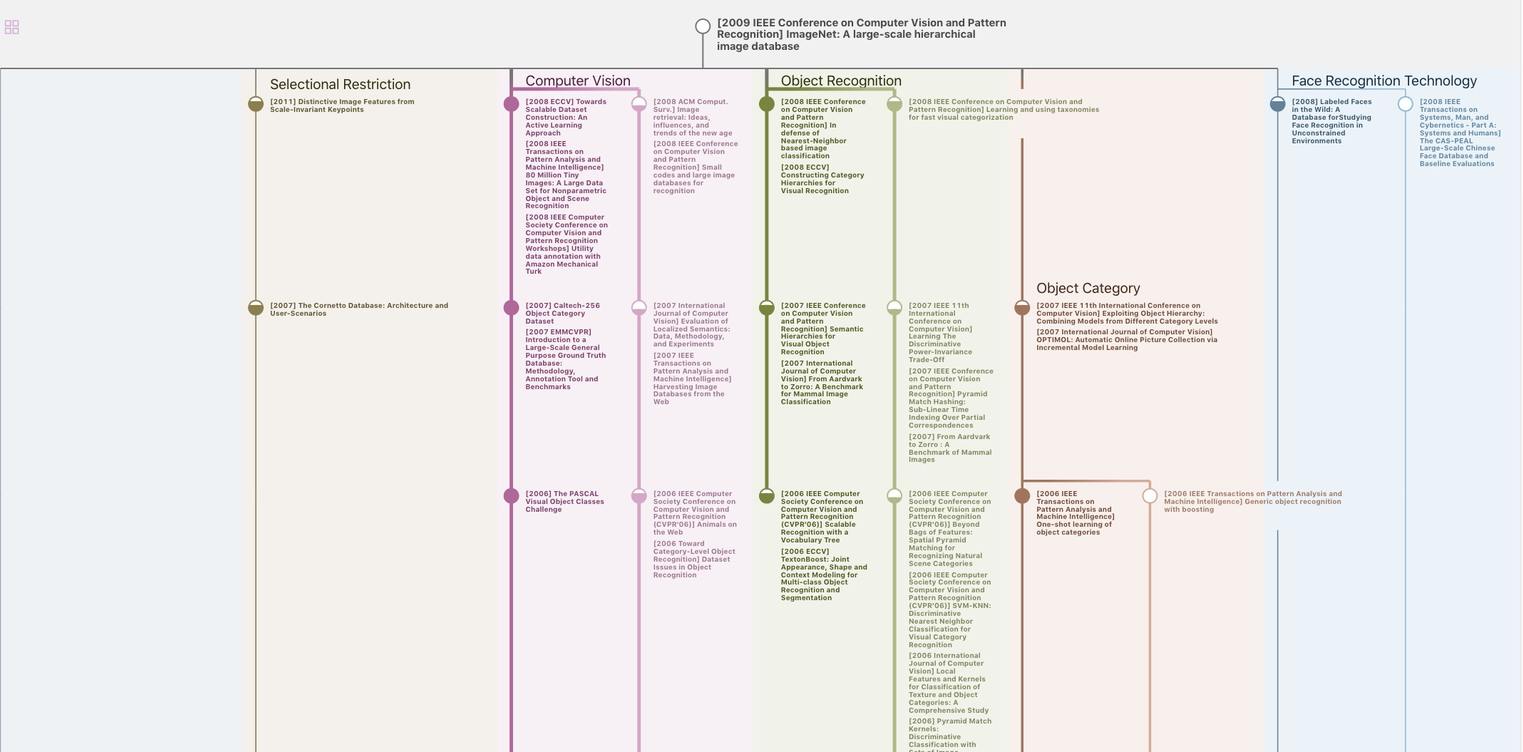
生成溯源树,研究论文发展脉络
Chat Paper
正在生成论文摘要