Online Metric Algorithms with Untrusted Predictions
ICML(2023)
摘要
Machine-learned predictors, although achieving very good results for inputs resembling training data, cannot possibly provide perfect predictions in all situations. Still, decision-making systems that are based on such predictors need not only benefit from good predictions, but should also achieve a decent performance when the predictions are inadequate. In this article, we propose a prediction setup for arbitrary metrical task systems (MTS) (e.g., caching, k-server, and convex body chasing) and online matching on the line. We utilize results from the theory of online algorithms to show how to make the setup robust. Specifically, for caching, we present an algorithm whose performance, as a function of the prediction error, is exponentially better than what is achievable for generalMTS. Finally, we present an empirical evaluation of our methods on real-world datasets, which suggests practicality.
更多查看译文
关键词
Metrical task systems,caching,competitive analysis
AI 理解论文
溯源树
样例
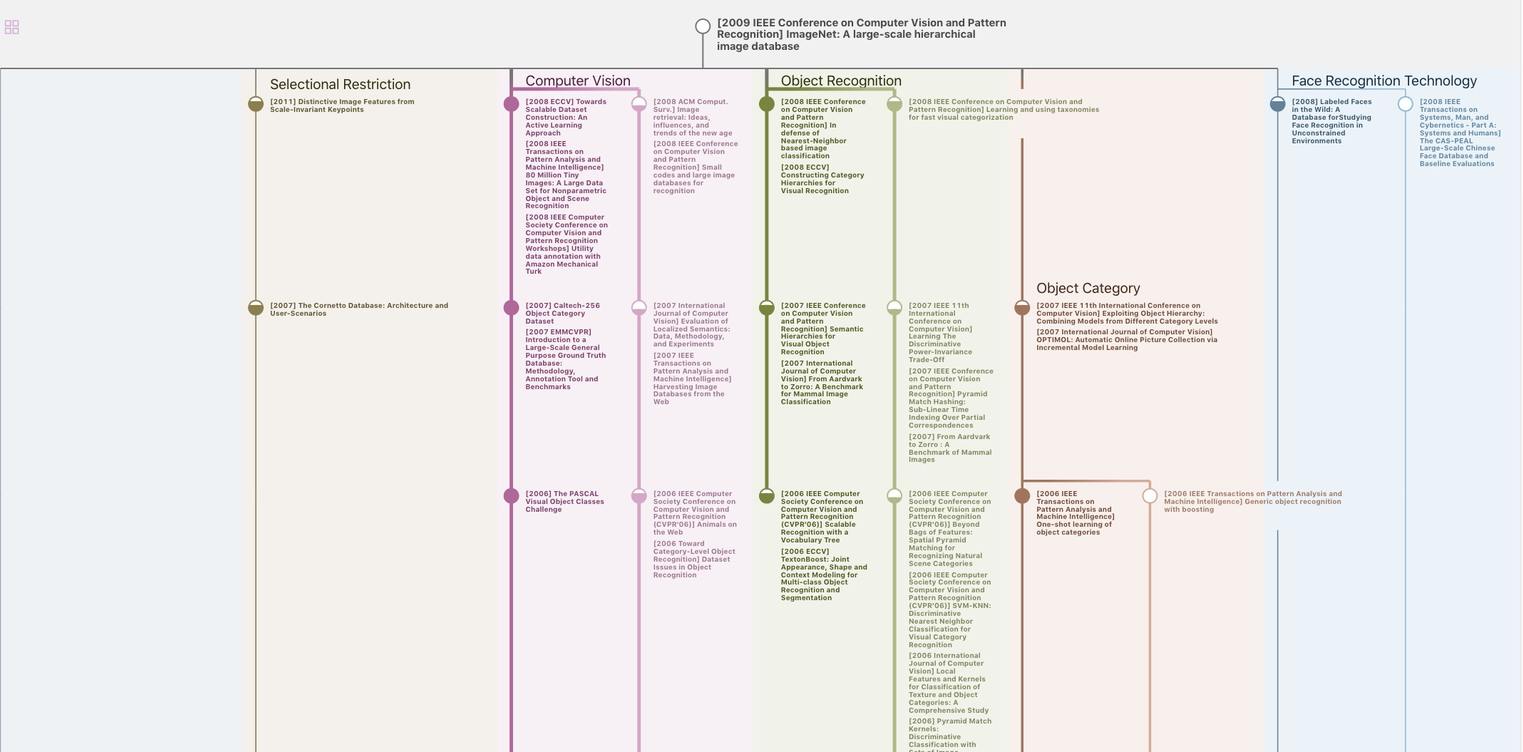
生成溯源树,研究论文发展脉络
Chat Paper
正在生成论文摘要