Preventing Clean Label Poisoning using Gaussian Mixture Loss
arxiv(2020)
摘要
Since 2014 when Szegedy et al. showed that carefully designed perturbations of the input can lead Deep Neural Networks (DNNs) to wrongly classify its label, there has been an ongoing research to make DNNs more robust to such malicious perturbations. In this work, we consider a poisoning attack called Clean Labeling poisoning attack (CLPA). The goal of CLPA is to inject seemingly benign instances which can drastically change decision boundary of the DNNs due to which subsequent queries at test time can be mis-classified. We argue that a strong defense against CLPA can be embedded into the model during the training by imposing features of the network to follow a Large Margin Gaussian Mixture distribution in the penultimate layer. By having such a prior knowledge, we can systematically evaluate how unusual the example is, given the label it is claiming to be. We demonstrate our builtin defense via experiments on MNIST and CIFAR datasets. We train two models on each dataset: one trained via softmax, another via LGM. We show that using LGM can substantially reduce the effectiveness of CLPA while having no additional overhead of data sanitization. The code to reproduce our results is available online.
更多查看译文
AI 理解论文
溯源树
样例
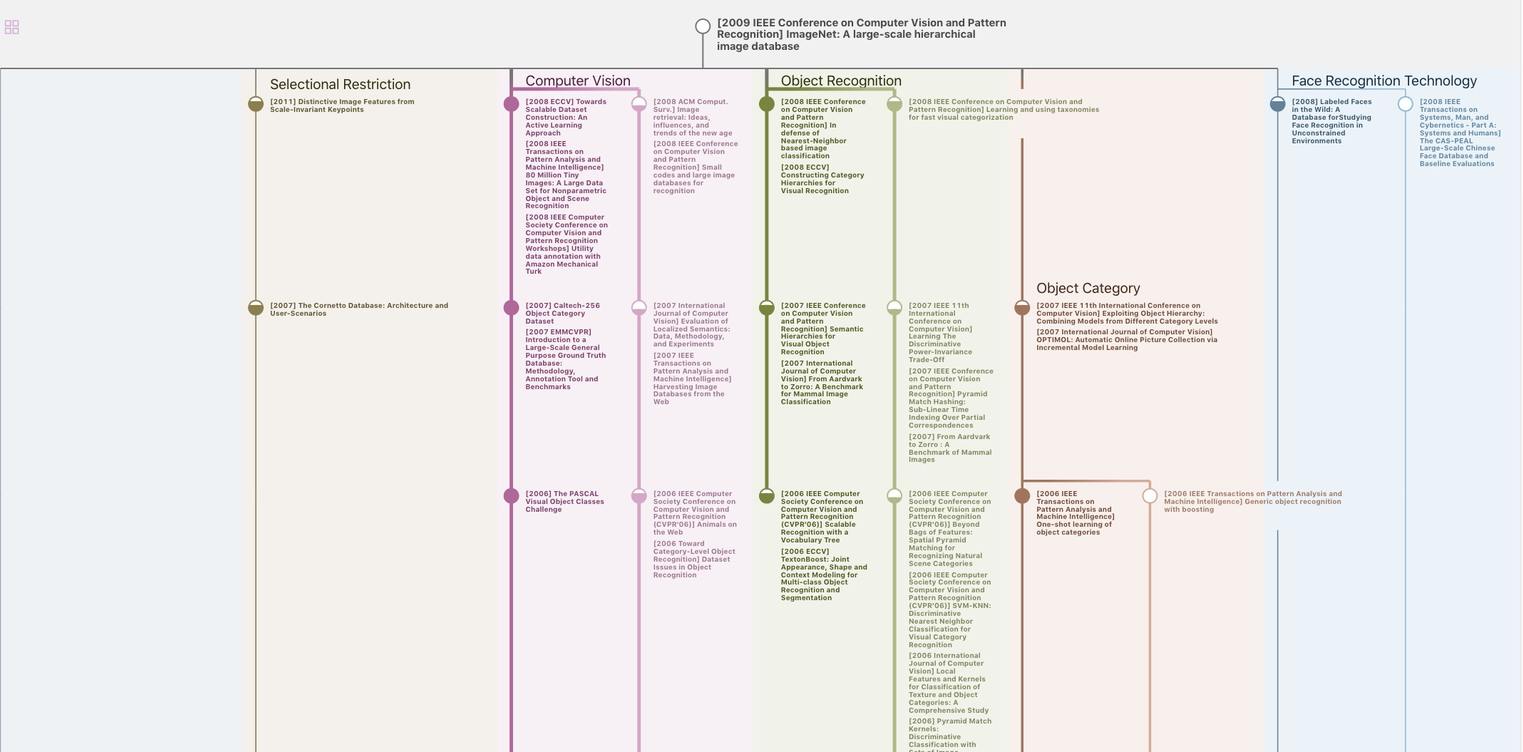
生成溯源树,研究论文发展脉络
Chat Paper
正在生成论文摘要