Finding Interesting Frames in Deep Video Analytics: a Top-K Approach
arxiv(2020)
摘要
Recently, the impressive accuracy of deep neural networks (DNNs) has created great demands on practical analytics over video data. Although efficient and accurate, the latest video analytics systems have not supported analytics beyond selection and aggregation yet. In data analytics, Top-K is a very important analytical operation that enables analysts to focus on the most important entities. In this paper, we present Everest, the first system that supports efficient and accurate Top-K video analytics. Everest can help rank and identify the most interesting frames/moments from videos with theoretical accuracy guarantees. Everest is built on a careful synthesis of machine learning, computer vision, and Top-K query processing. Evaluations on six real-world video streams and the latest Visual Road benchmark show that Everest can achieve between 10.8x to 17.9x higher efficiency than baseline approaches with high accuracy.
更多查看译文
关键词
deep video analytics,interesting frames
AI 理解论文
溯源树
样例
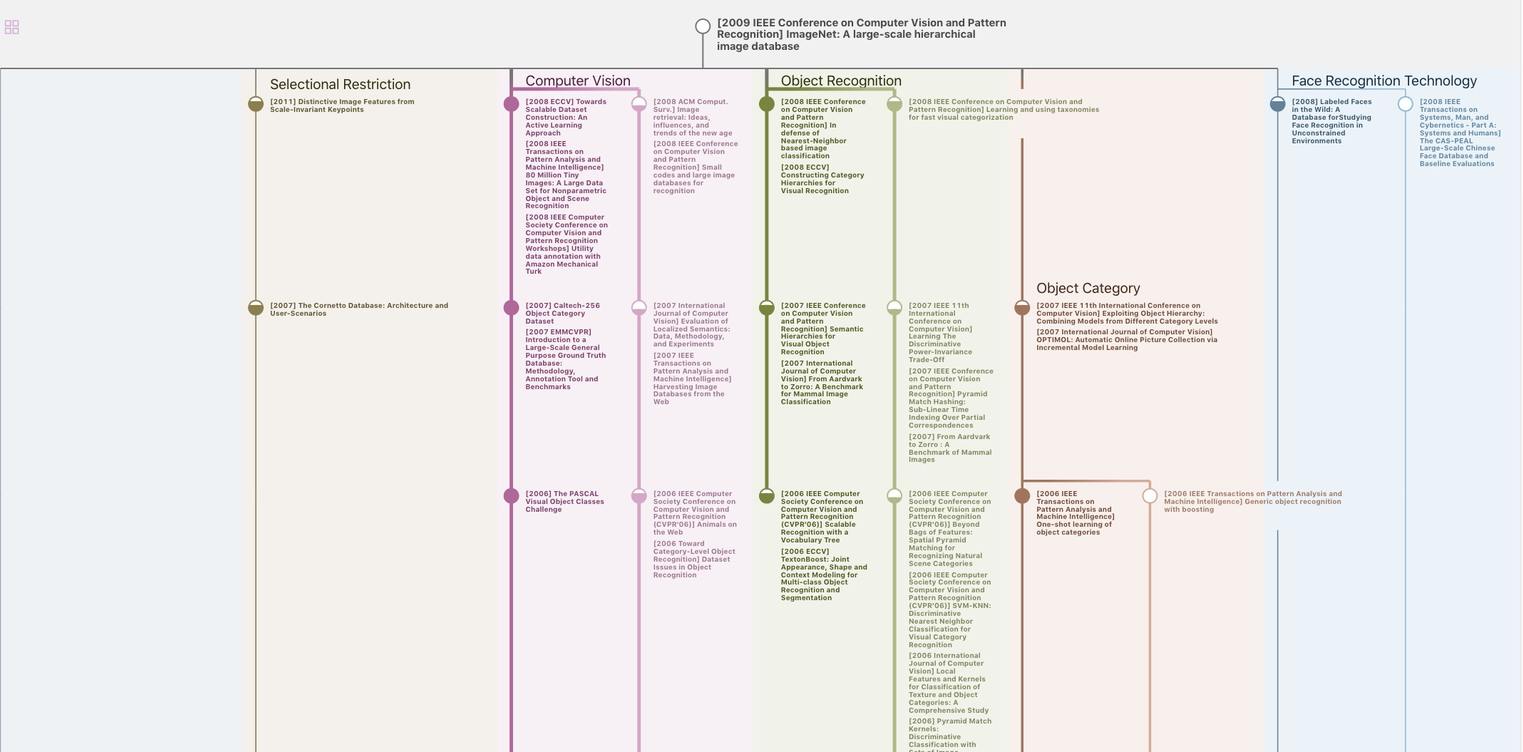
生成溯源树,研究论文发展脉络
Chat Paper
正在生成论文摘要