Deep Reinforcement Learning For Fresh Data Collection In Uav-Assisted Iot Networks
IEEE INFOCOM 2020 - IEEE CONFERENCE ON COMPUTER COMMUNICATIONS WORKSHOPS (INFOCOM WKSHPS)(2020)
摘要
Due to the flexibility and low operational cost, dispatching unmanned aerial vehicles (UAVs) to collect information from distributed sensors is expected to be a promising solution in Internet of Things (IoT), especially for time-critical applications. How to maintain the information freshness is a challenging issue. In this paper, we investigate the fresh data collection problem in UAV-assisted IoT networks. Particularly, the UAV flies towards the sensors to collect status update packets within a given duration while maintaining a non-negative residual energy. We formulate a Markov Decision Process (MDP) to find the optimal flight trajectory of the UAV and transmission scheduling of the sensors that minimizes the weighted sum of the age of information (AoI). A UAV-assisted data collection algorithm based on deep reinforcement learning (DRL) is further proposed to overcome the curse of dimensionality. Extensive simulation results demonstrate that the proposed DRL-based algorithm can significantly reduce the weighted sum of the AoI compared to other baseline algorithms.
更多查看译文
关键词
deep reinforcement learning,UAV-assisted IoT networks,unmanned aerial vehicles,distributed sensors,time-critical applications,information freshness,fresh data collection problem,UAV-assisted data collection algorithm,Markov decision process,MDP,optimal flight trajectory,transmission scheduling,age of information,AoI,DRL
AI 理解论文
溯源树
样例
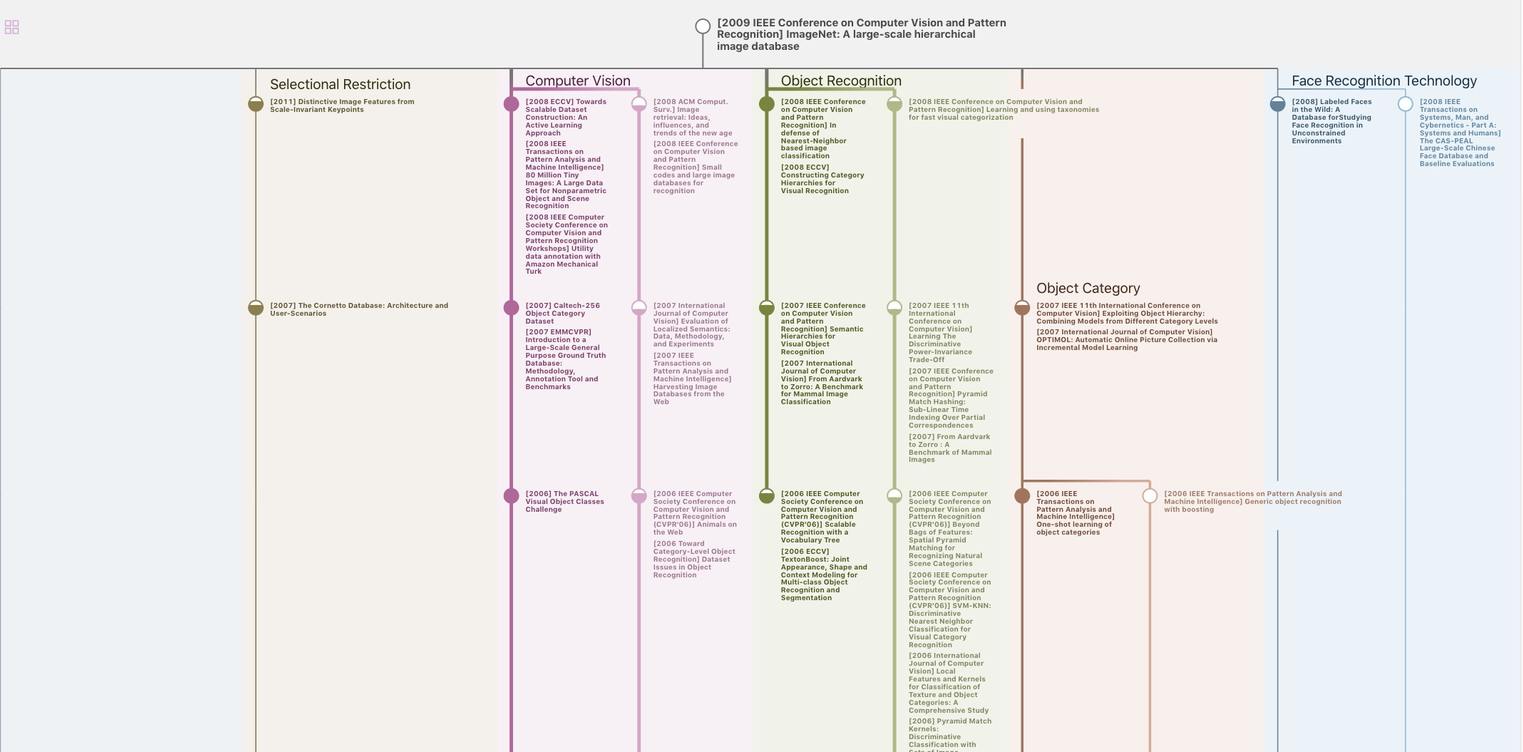
生成溯源树,研究论文发展脉络
Chat Paper
正在生成论文摘要