Online Binary Space Partitioning Forests
INTERNATIONAL CONFERENCE ON ARTIFICIAL INTELLIGENCE AND STATISTICS, VOL 108(2020)
摘要
The Binary Space Partitioning-Tree~(BSP-Tree) process was recently proposed as an efficient strategy for space partitioning tasks. Because it uses more than one dimension to partition the space, the BSP-Tree Process is more efficient and flexible than conventional axis-aligned cutting strategies. However, due to its batch learning setting, it is not well suited to large-scale classification and regression problems. In this paper, we develop an online BSP-Forest framework to address this limitation. With the arrival of new data, the resulting online algorithm can simultaneously expand the space coverage and refine the partition structure, with guaranteed universal consistency for both classification and regression problems. The effectiveness and competitive performance of the online BSP-Forest is verified via simulations on real-world datasets.
更多查看译文
关键词
binary,online
AI 理解论文
溯源树
样例
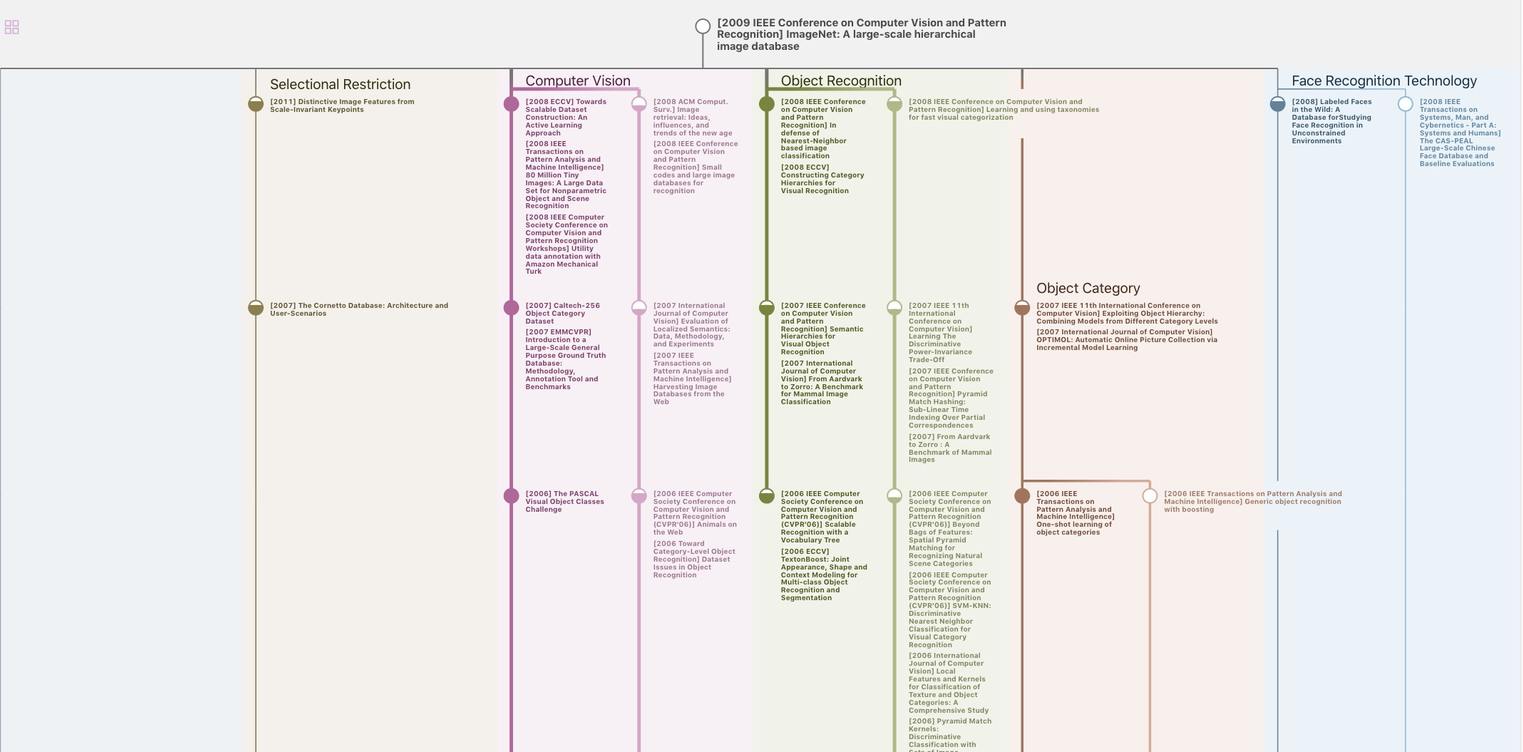
生成溯源树,研究论文发展脉络
Chat Paper
正在生成论文摘要