Multiseries Featural LSTM for Partial Periodic Time-Series Prediction: A Case Study for Steel Industry
IEEE Transactions on Instrumentation and Measurement(2020)
摘要
Partial periodic time series are found in many areas. However, many partial periodic time-series prediction methods are unable to capture the feature dependence within the fluctuation of data. In this article, a multiseries featural long short-term memory (LSTM) is proposed. A novel template-matching method is used to extract specific periodic characteristics adaptively and restack the 1-D time series into multiseries featural structure data. The extended series is fed into a multivariable LSTM network to exploit the feature-temporal patterns for predictions. To enhance the long-term prediction performance, a period correction method is used to reduce the iteration errors caused by multistep prediction. To demonstrate the effectiveness of the proposed method, two classical partial periodic data sets and two byproduct gas data sets are studied here. Our results demonstrate that the proposed prediction method has advantages on prediction accuracy, especially for the critical structural features, that satisfies the requirements of the practically viable prediction.
更多查看译文
关键词
Energy system,long short-term memory (LSTM),partial periodic,steel industry,time-series prediction
AI 理解论文
溯源树
样例
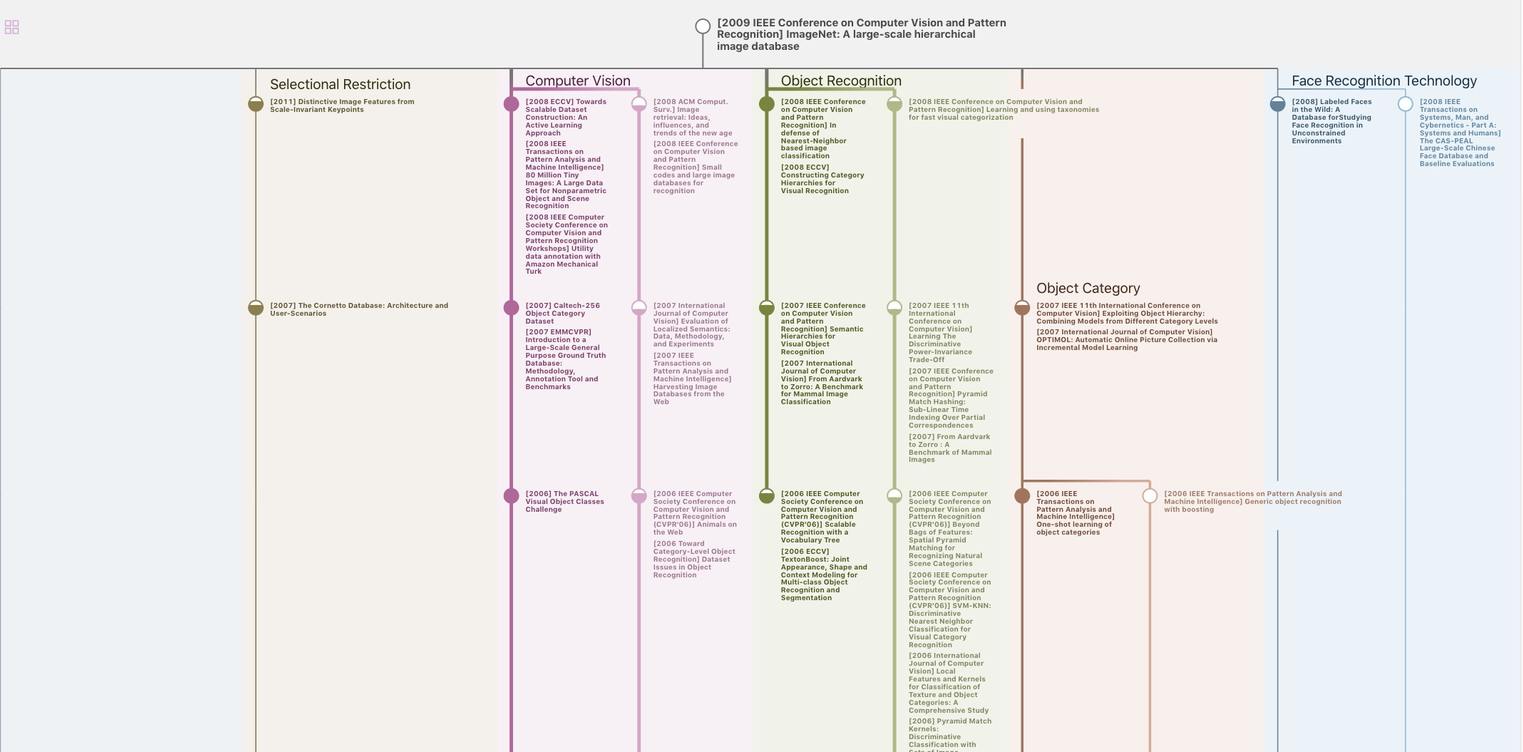
生成溯源树,研究论文发展脉络
Chat Paper
正在生成论文摘要