A Multiscale Deep Learning Approach for High-Resolution Hyperspectral Image Classification
IEEE Geoscience and Remote Sensing Letters(2021)
摘要
Hyperspectral imagery (HSI) has emerged as a highly successful sensing modality for a variety of applications ranging from urban mapping to environmental monitoring and precision agriculture. Despite the efforts made by the scientific community, developing reliable algorithms of HSI classification remains a challenging problem, especially for high-resolution HSI data where there is often larger intraclass variability combined with a scarcity of the ground-truth data and class imbalance. In recent years, deep neural networks have emerged as a promising strategy for problems of HSI classification where they have shown remarkable potential for learning joint spectral-spatial features efficiently via backpropagation. In this letter, we propose a deep learning strategy for HSI classification that combines different convolutional neural networks, especially designed to efficiently learn joint spatial-spectral features over multiple scales. Our method achieves an overall classification accuracy of 66.73% on the 2018 IEEE GRSS hyperspectral data set-a high-resolution data set that includes 20 urban land-cover and land-use classes.
更多查看译文
关键词
Convolutional neural networks (CNNs),deep learning,hyperspectral data,multiscale analysis
AI 理解论文
溯源树
样例
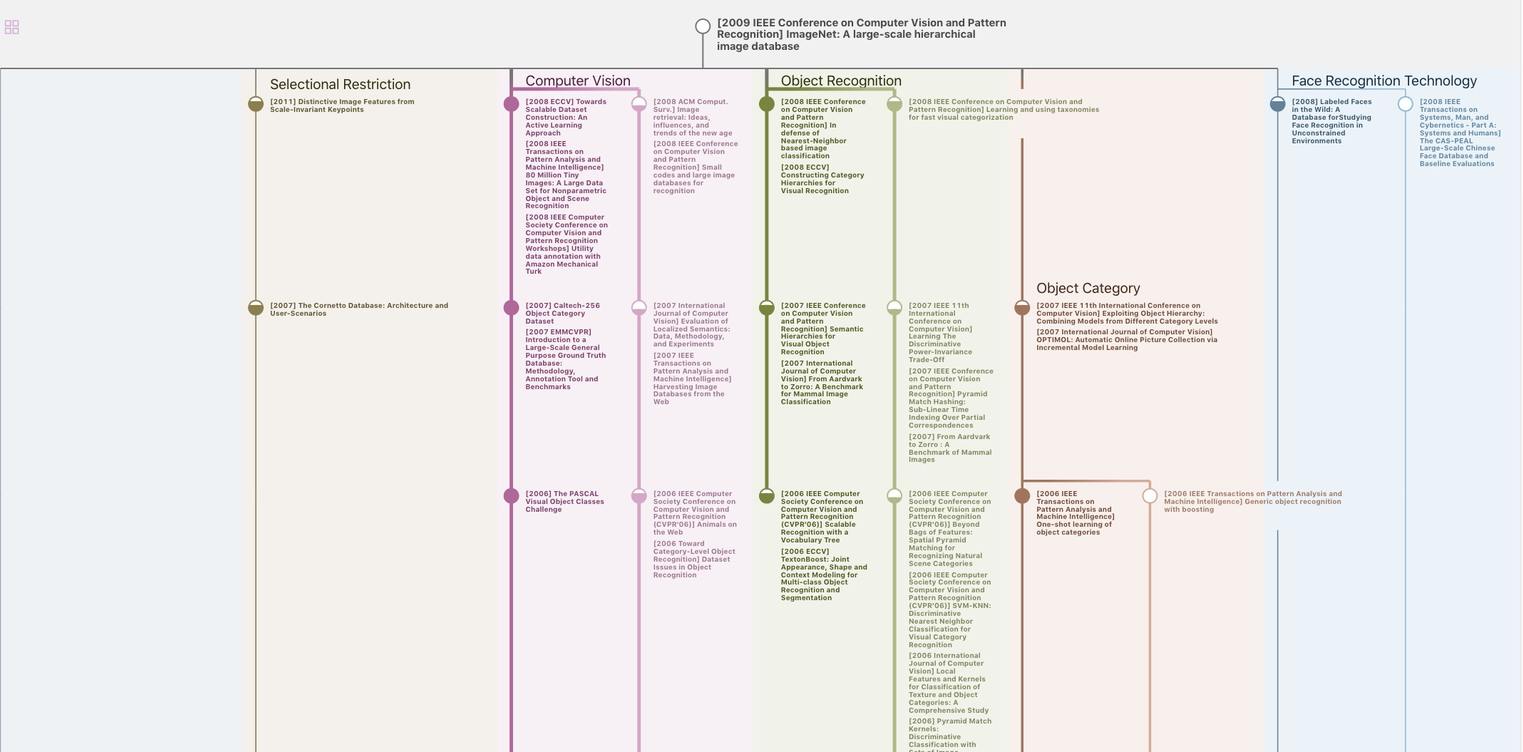
生成溯源树,研究论文发展脉络
Chat Paper
正在生成论文摘要