Multi-Level Correlation Adversarial Hashing for Cross-Modal Retrieval
IEEE Transactions on Multimedia(2020)
摘要
Cross-modal hashing (CMH) has been widely used for similarity search in multimedia retrieval applications, thanks to low storage cost and fast query speed. However, preserving the content similarities in finite-length hash codes between different data modalities is still challenging due to the existing heterogeneity gap. To further address the crucial bottleneck, we propose a Multi-Level Correlation Adversarial Hashing (MLCAH) algorithm to integrate the multi-level correlation information into hash codes. The proposed MLCAH model enjoys several merits. First, to the best of our knowledge, it is the early attempt of leveraging the multi-level correlation information for cross-modal hashing retrieval. Second, we propose global and local semantic alignment mechanisms, which can effectively encode multi-level correlation information, including global information, local information, and label information into hash codes. Third, a label-consistency attention mechanism with adversarial training is designed for exploiting the local cross-modality similarity from multi-modality data. Extensive evaluations on four benchmarks demonstrate that the proposed model brings significant improvements over several state-of-the-art cross-modal hashing methods.
更多查看译文
关键词
Cross-modal retrieval,adversarial hashing,multi-level correlation
AI 理解论文
溯源树
样例
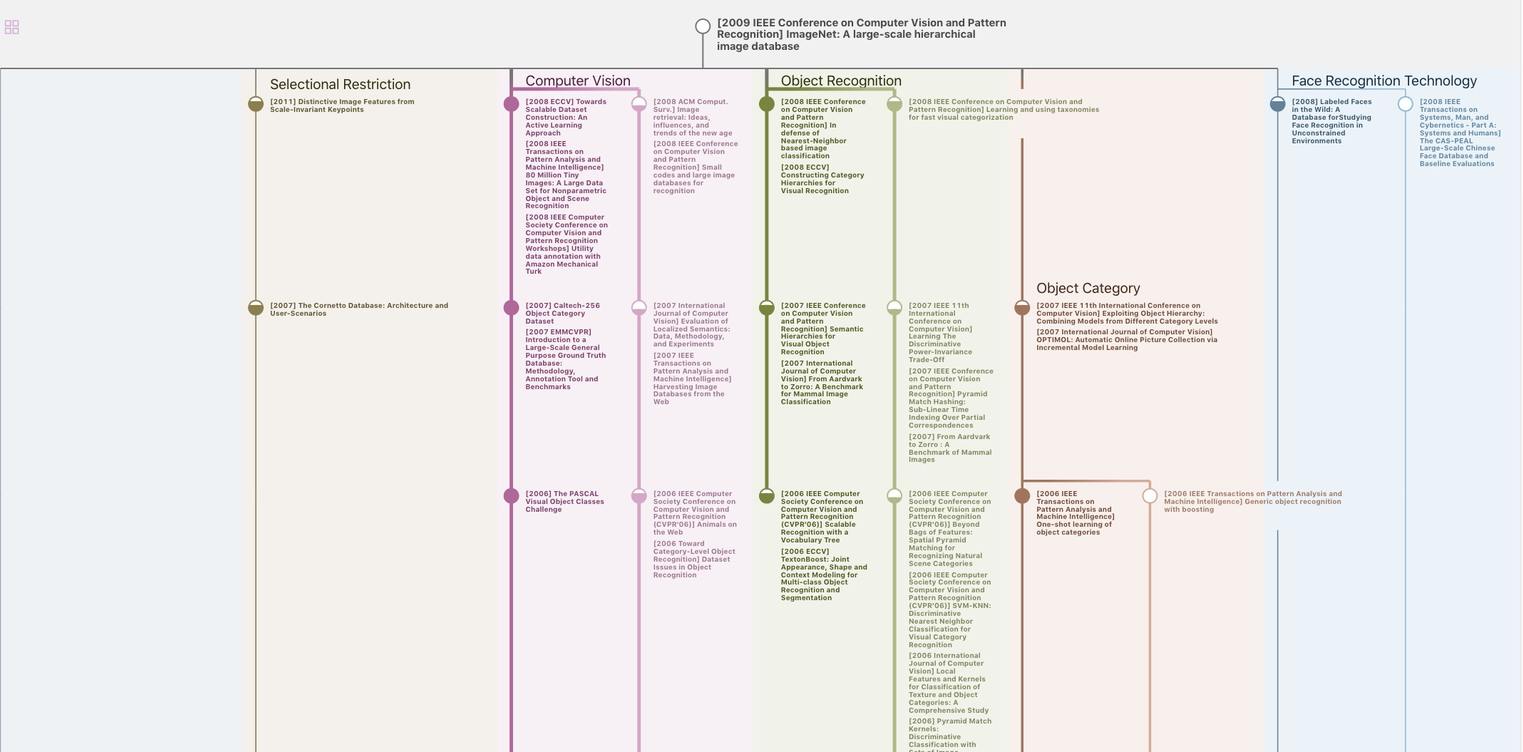
生成溯源树,研究论文发展脉络
Chat Paper
正在生成论文摘要