Forecasting College Football Game Outcomes Using Modern Modeling Techniques
JOURNAL OF SPORTS ANALYTICS(2020)
摘要
There are many reasons why data scientists and fans of college football would want to forecast the outcome of games - gambling, game preparation and academic research, for example. As advanced statistical methods become more readily accessible, so do the opportunities to develop robust forecasting models. Using data from the 2011 to 2014 seasons, we implemented a variety of advanced modeling techniques to determine which best forecasts the outcome of games. These methods included ridge regression, the lasso, the elastic net, neural networks, random forests, k-nearest neighbors, stochastic gradient boosting, and a Bayesian regression model. To evaluate the efficacy of the proposed models, we tested them on data from the 2015 season. The top performers - lasso regression, a Bayesian regression with team-specific variances, stochastic gradient boosting, and random forests - predicted the correct outcome over 70% of the time, and the lasso model proved most accurate at predicting win-loss outcomes in the 2015 test data set.
更多查看译文
关键词
NCAA football, prediction, machine learning, Bayesian, variable selection
AI 理解论文
溯源树
样例
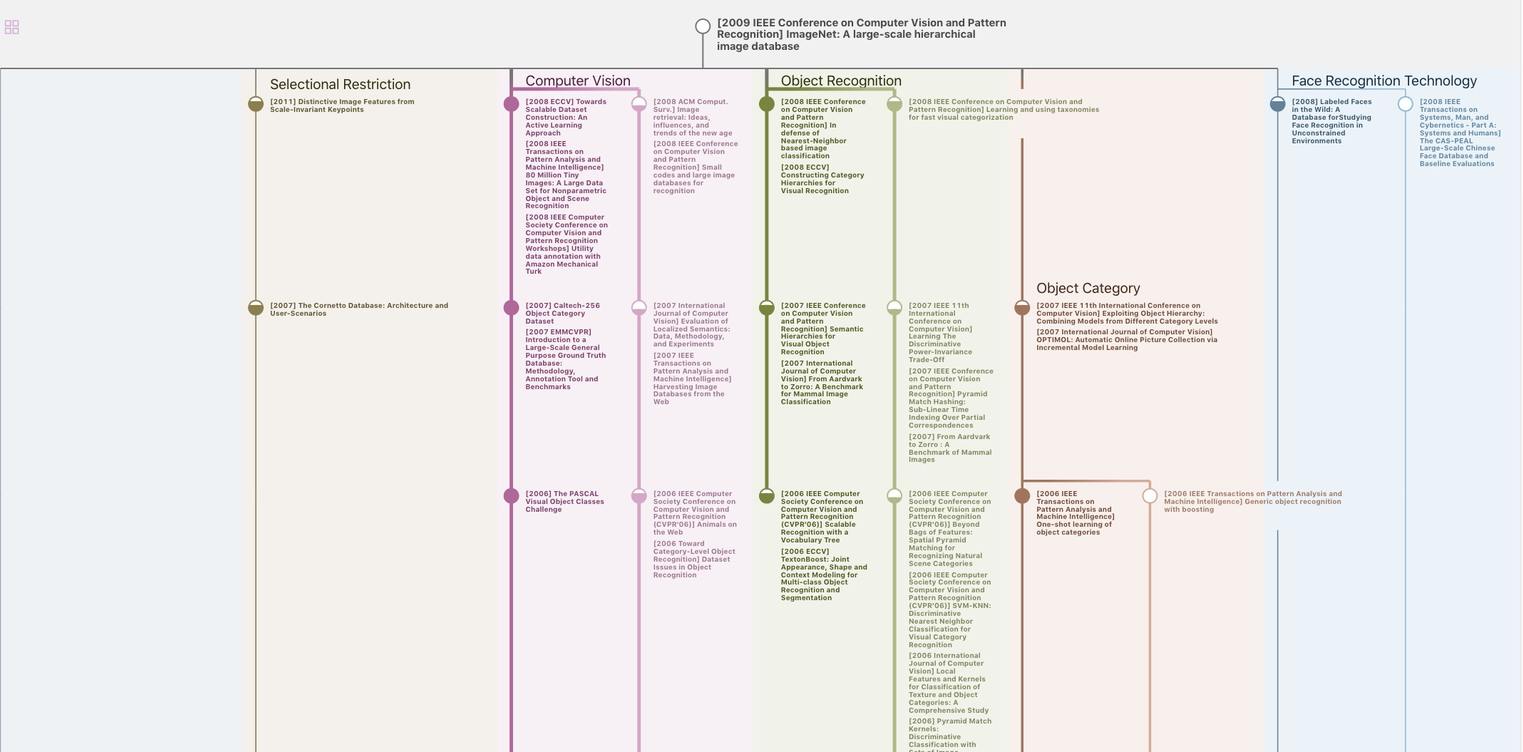
生成溯源树,研究论文发展脉络
Chat Paper
正在生成论文摘要