Automatic Building Extraction From High-Resolution Aerial Imagery Via Fully Convolutional Encoder-Decoder Network With Non-Local Block
IEEE ACCESS(2020)
摘要
Extracting buildings automatically from high-resolution aerial images is a significant and fundamental task for various practical applications, such as land-use statistics and urban planning. Recently, various methods based on deep learning, especially the fully convolution networks, achieve impressive scores in this challenging semantic segmentation task. However, the lack of global contextual information and the careless upsampling method limit the further improvement of the performance for building extraction task. To simultaneously address these problems, we propose a novel network named Efficient Non-local Residual U-shape Network(ENRU-Net), which is composed of a well designed U-shape encoder-decoder structure and an improved non-local block named asymmetric pyramid non-local block (APNB). The encoder-decoder structure is adopted to extract and restore the feature maps carefully, and APNB could capture global contextual information by utilizing self-attention mechanism. We evaluate the proposed ENRU-Net and compare it with other state-of-the-art models on two widely-used public aerial building imagery datasets: the Massachusetts Buildings Dataset and the WHU Aerial Imagery Dataset. The experiments show that the accuracy of ENRU-Net on these datasets has remarkable improvement against previous state-of-the-art semantic segmentation models, including FCN-8s, U-Net, SegNet and Deeplab v3. The subsequent analysis also indicates that our ENRU-Net has advantages in efficiency for building extraction from high-resolution aerial images.
更多查看译文
关键词
Deep learning, semantic segmentation, fully convolution network, building extraction, non-local method
AI 理解论文
溯源树
样例
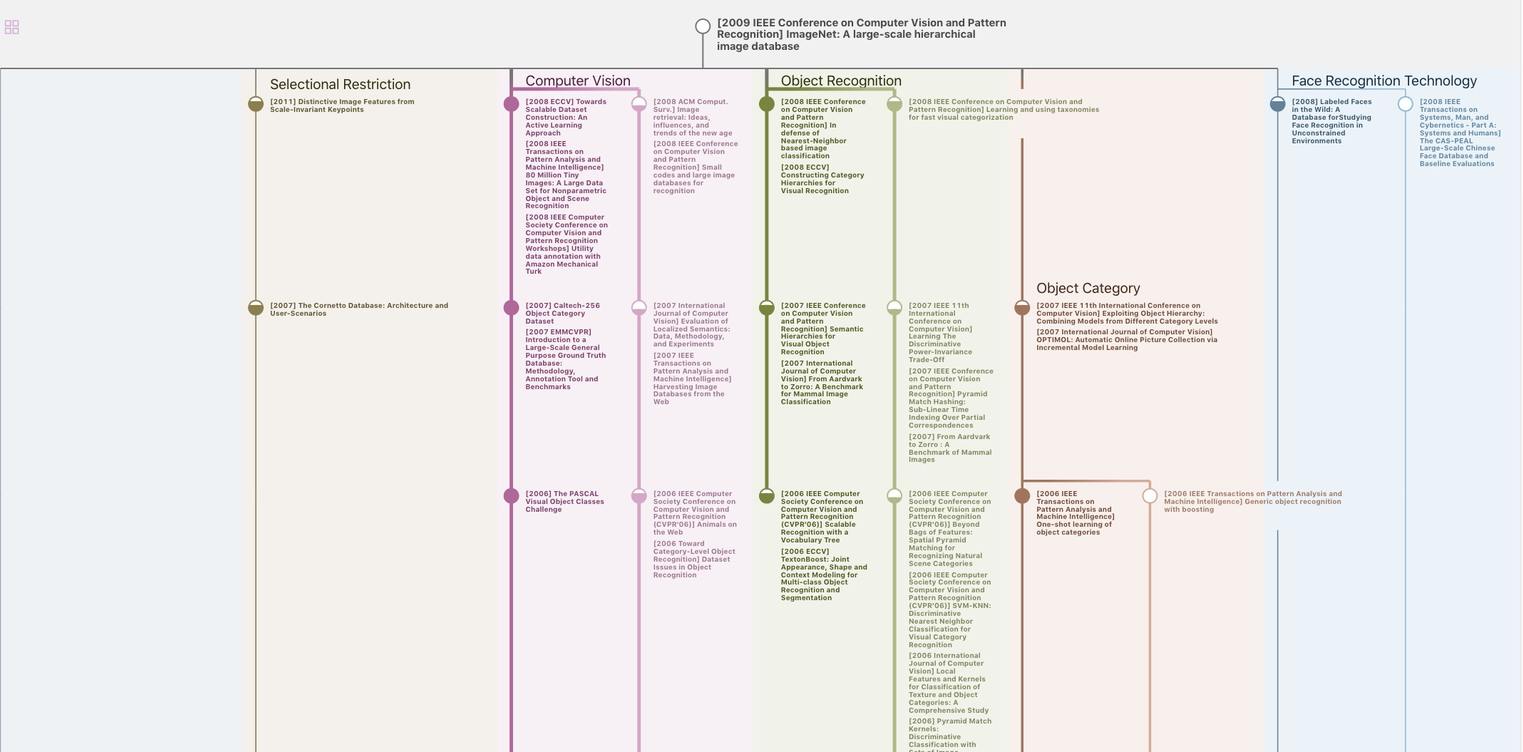
生成溯源树,研究论文发展脉络
Chat Paper
正在生成论文摘要