Spatial-Temporal Gaussian Scale Mixture Modeling For Foreground Estimation
AAAI(2020)
摘要
Subtracting the backgrounds from the video frames is an important step for many video analysis applications. Assuming that the backgrounds are low-rank and the foregrounds are sparse, the robust principle component analysis (RPCA)-based methods have shown promising results. However, the RPCA-based methods suffered from the scale issue, i.e., the l(1)-sparsity regularizer fails to model the varying sparsity of the moving objects. While several efforts have been made to address this issue with advanced sparse models, previous methods cannot fully exploit the spatial-temporal correlations among the foregrounds. In this paper, we proposed a novel spatial-temporal Gaussian scale mixture (STGSM) model for foreground estimation. In the proposed STGSM model, a temporal consistent constraint is imposed over the estimated foregrounds through nonzero-means Gaussian models. Specifically, the estimates of the foregrounds obtained in the previous frame are used as the prior for these of the current frame, and nonzero means Gaussian scale mixture models (GSM) are developed. To better characterize the temporal correlations, the optical flow has been used to model the correspondences between foreground pixels in adjacent frames. The spatial correlations have also been exploited by considering that local correlated pixels should be characterized by the same STGSM model, leading to further performance improvements. Experimental results on real video datasets show that the proposed method performs comparably or even better than current state-of-the-art background subtraction methods.
更多查看译文
AI 理解论文
溯源树
样例
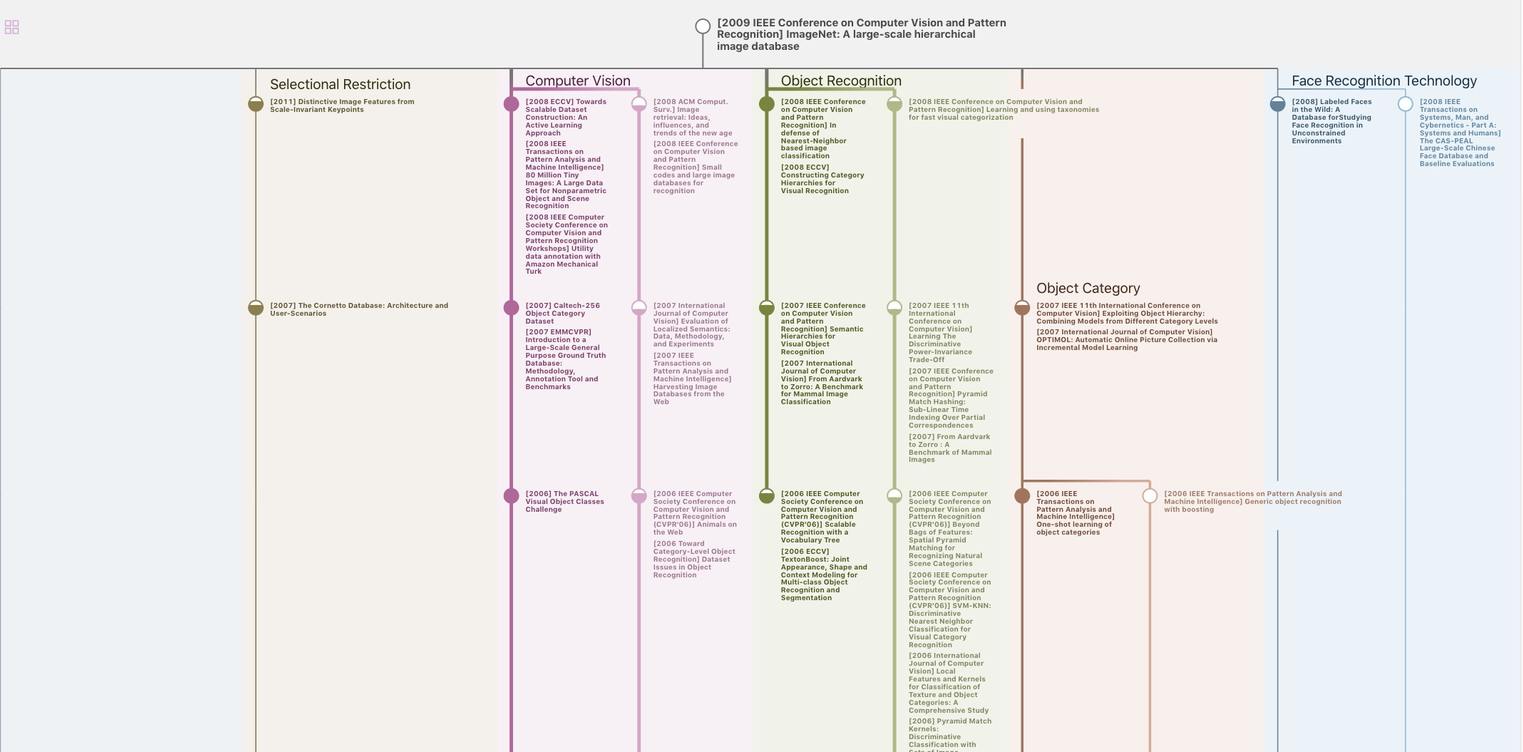
生成溯源树,研究论文发展脉络
Chat Paper
正在生成论文摘要