Q-Value Prediction for Reinforcement Learning Assisted Garbage Collection to Reduce Long Tail Latency in SSD
IEEE Transactions on Computer-Aided Design of Integrated Circuits and Systems(2020)
摘要
Garbage collection (GC), an essential operation for flash storage systems, causes long tail latency which is one of the key problems in real-time and quality-critical systems. In this article, we take advantage of reinforcement learning (RL) to reduce long tail latency. Especially, we propose two novel techniques which are: 1)
$Q$
-table cache (QTC) and 2)
$Q$
-value prediction. The QTC allows us to utilize appropriate and frequently recurring key states at a small memory cost. We propose a neural network called
$Q$
-value prediction network (QP Net) that predicts the initial
$Q$
-value of a new state in the QTC. The integrated solution of QTC and QP Net enables us to benefit from both short-term (by QTC) and long-term (by QP Net) history of system behavior to reduce the long tail latency. The experimental results demonstrate that the proposed scheme offers significant (by 25%–37%) reductions in the long tail latency of storage-intensive workloads compared with the state-of-the-art solution that adopts an RL-assisted GC scheduler.
更多查看译文
关键词
Reinforcement learning,Memory management,Random access memory,Flash memories,Real-time systems,Neural networks,Three-dimensional displays
AI 理解论文
溯源树
样例
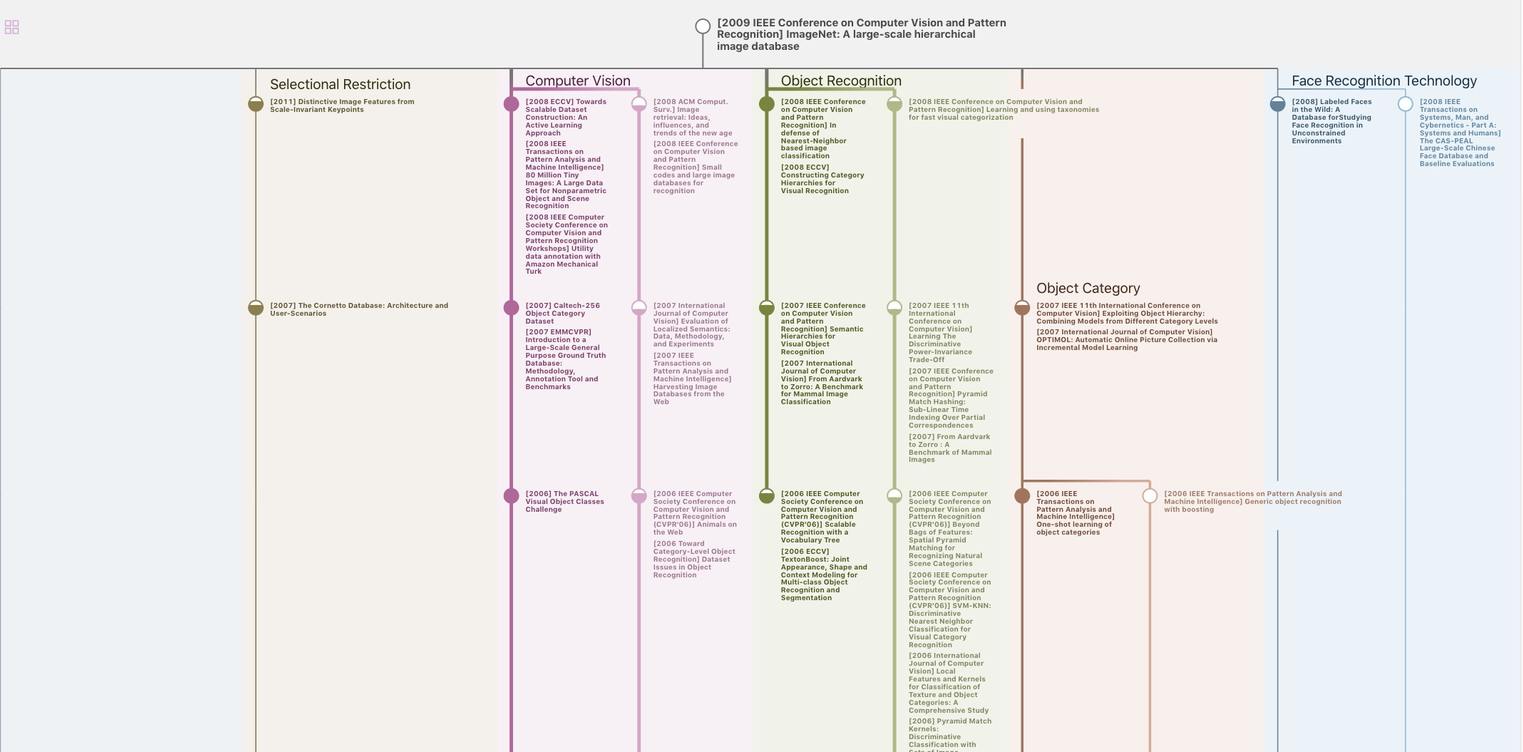
生成溯源树,研究论文发展脉络
Chat Paper
正在生成论文摘要