Lagrangian Fluid Simulation with Continuous Convolutions
ICLR(2020)
摘要
We present an approach to Lagrangian fluid simulation with a new type of convolutional network. Our networks process sets of moving particles, which describe fluids in space and time. Unlike previous approaches, we do not build an explicit graph structure to connect the particles but use spatial convolutions as the main differentiable operation that relates particles to their neighbors. To this end we present a simple, novel, and effective extension of N-D convolutions to the continuous domain. We show that our network architecture can simulate different materials, generalizes to arbitrary collision geometries, and can be used for inverse problems. In addition, we demonstrate that our continuous convolutions outperform prior formulations in terms of accuracy and speed.
更多查看译文
关键词
particle-based physics, fluid mechanics, continuous convolutions, material estimation
AI 理解论文
溯源树
样例
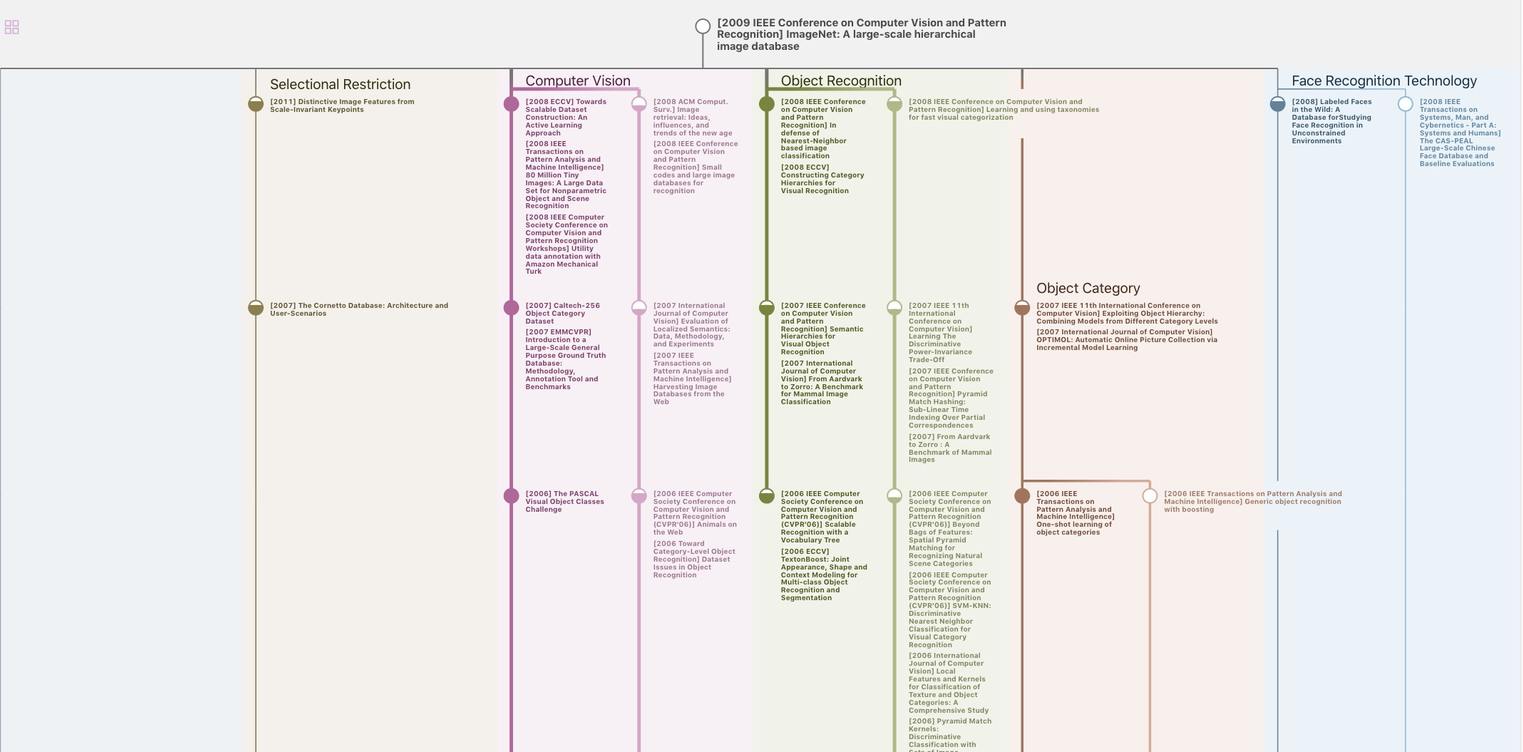
生成溯源树,研究论文发展脉络
Chat Paper
正在生成论文摘要