Driving-Condition Dependent and Monte Carlo Simulation-Based Optimization Method for a Bayesian Localization Filter for Parking
IEEE Transactions on Intelligent Vehicles(2020)
摘要
In this article, a method for the automated adaptive tuning of a Bayesian localization filter is investigated, since the tuning parameters were often only empirically determined and constantly set. The method is applied to a previously developed Bayesian localization filter called Odometry 2.0 estimator. Its architecture makes it feasible to individually combine different dead-reckoning models because the input is redundant. However, under different driving-conditions (DCs), in particular when parking, the models have individual advantages and disadvantages. Therefore, different cluster analysis methods are examined in order to automatically divide a parking maneuver into different useful segments and to enable dc-dependent optimization. In the next step these segments and statistical error and coefficient models serve as input for a Monte Carlo Simulation (MCS). The error model provides potential sensor errors to ensure robust filter tuning and the coefficient model provides randomly found filter settings in the large parameter space. Finally, the MCS results and ground truth data are used for the optimization to find the filter tuning with minor model errors. The application of the so found adaptive tuning setting shows a significant increase in the robustness of the localization filter.
更多查看译文
关键词
Tuning,Optimization,Bayes methods,Dead reckoning,Adaptation models,Mathematical model,Intelligent vehicles
AI 理解论文
溯源树
样例
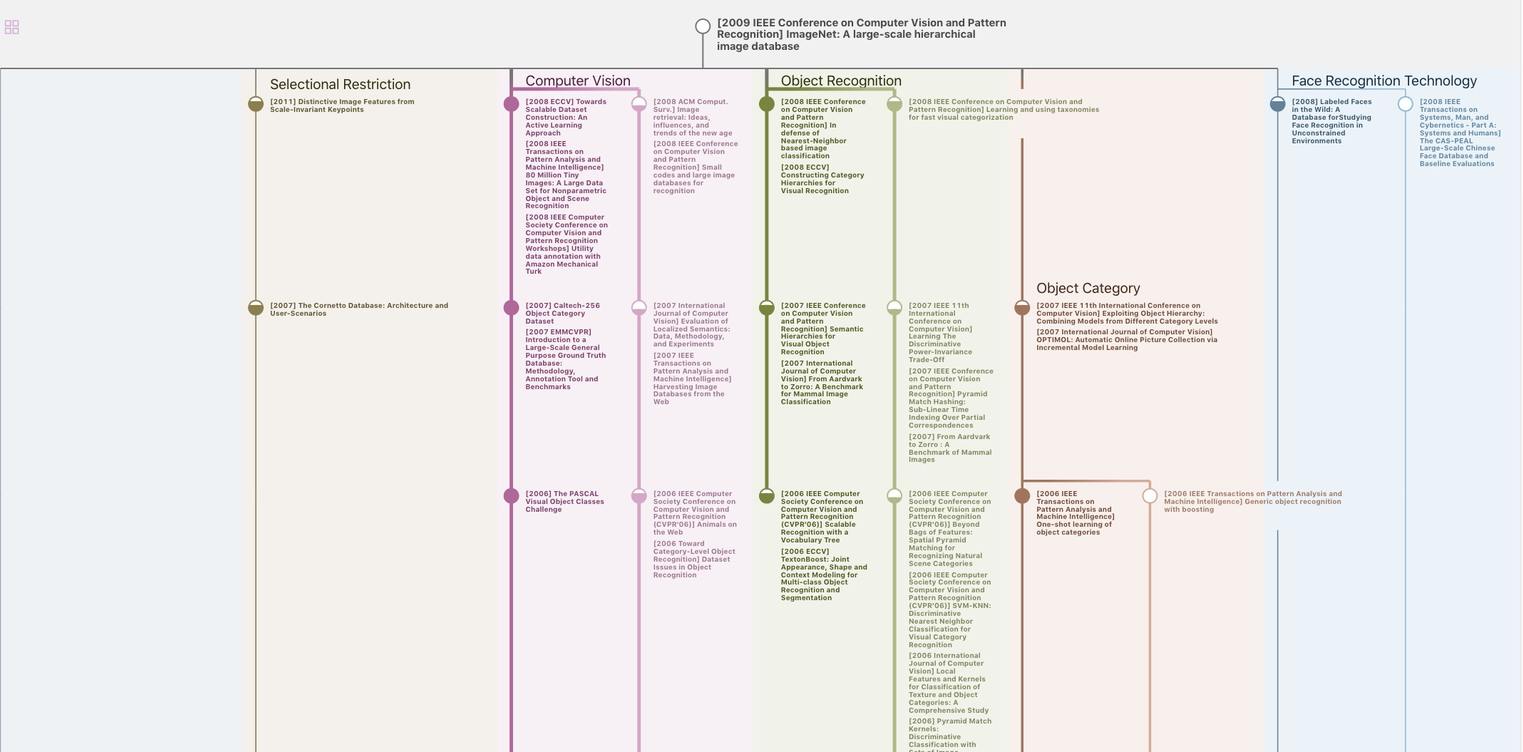
生成溯源树,研究论文发展脉络
Chat Paper
正在生成论文摘要