Cross-layer Feature Pyramid Network for Salient Object Detection
arxiv(2020)
摘要
Feature pyramid network (FPN) based models, which fuse the semantics and salient details in a progressive manner, have been proven highly effective in salient object detection. However, it is observed that these models often generate saliency maps with incomplete object structures or unclear object boundaries, due to the \emph{indirect} information propagation among distant layers that makes such fusion structure less effective. In this work, we propose a novel Cross-layer Feature Pyramid Network (CFPN), in which direct cross-layer communication is enabled to improve the progressive fusion in salient object detection. Specifically, the proposed network first aggregates multi-scale features from different layers into feature maps that have access to both the high- and low-level information. Then, it distributes the aggregated features to all the involved layers to gain access to richer context. In this way, the distributed features per layer own both semantics and salient details from all other layers simultaneously, and suffer reduced loss of important information. Extensive experimental results over six widely used salient object detection benchmarks and with three popular backbones clearly demonstrate that CFPN can accurately locate fairly complete salient regions and effectively segment the object boundaries.
更多查看译文
关键词
Feature extraction, Object detection, Semantics, Saliency detection, Fuses, Visualization, Computer architecture, Salient object detection, cross-layer communication, progressive feature fusion
AI 理解论文
溯源树
样例
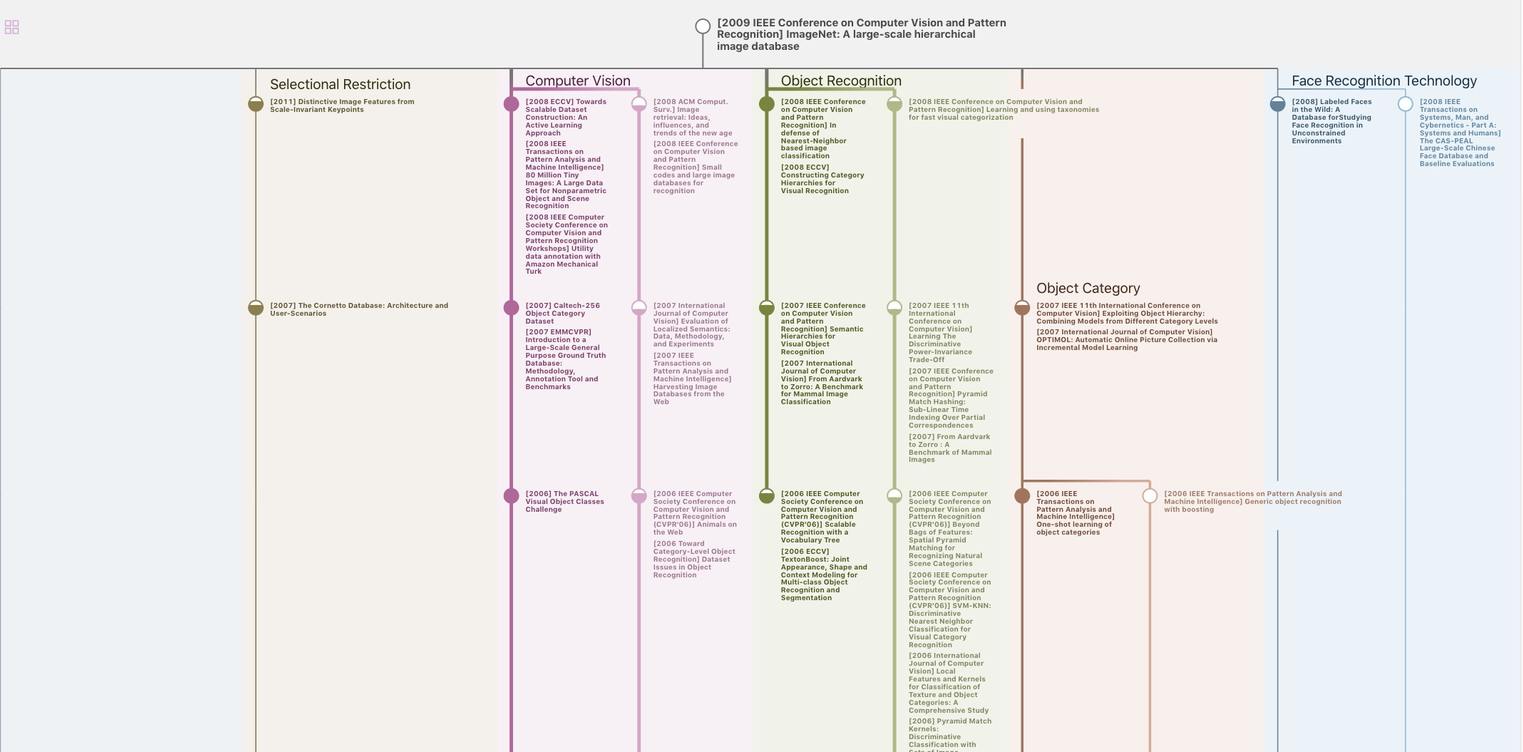
生成溯源树,研究论文发展脉络
Chat Paper
正在生成论文摘要