Robust Belief State Space Representation For Statistical Dialogue Managers Using Deep Autoencoders
2019 IEEE AUTOMATIC SPEECH RECOGNITION AND UNDERSTANDING WORKSHOP (ASRU 2019)(2019)
摘要
Statistical Dialogue Systems (SDS) have proved their humongous potential over the past few years. However, the lack of efficient and robust representations of the belief state (BS) space refrains them from revealing their full potential. There is a great need for automatic BS representations, which will replace the old hand-crafted, variable-length ones. To tackle those problems, we introduce a novel use of Autoencoders (AEs). Our goal is to obtain a low-dimensional, fixed-length, and compact, yet robust representation of the BS space. We investigate the use of dense AE, Denoising AE (DAE) and Variational Denoising AE (VDAE), which we combine with GP-SARSA to learn dialogue policies in the PyDial toolkit. In this framework, the BS is normally represented in a relatively compact, but still redundant summary space which is obtained through a heuristic mapping of the original master space. We show that all the proposed AE-based representations consistently outperform the summary BS representation. Especially, as the Semantic Error Rate (SER) increases, the DAE/VDAE-based representations obtain state-of-the-art and sample efficient performance.
更多查看译文
关键词
denoising autoencoder, variational autoencoder, statistical dialogue system, dialogue manager, belief state representation
AI 理解论文
溯源树
样例
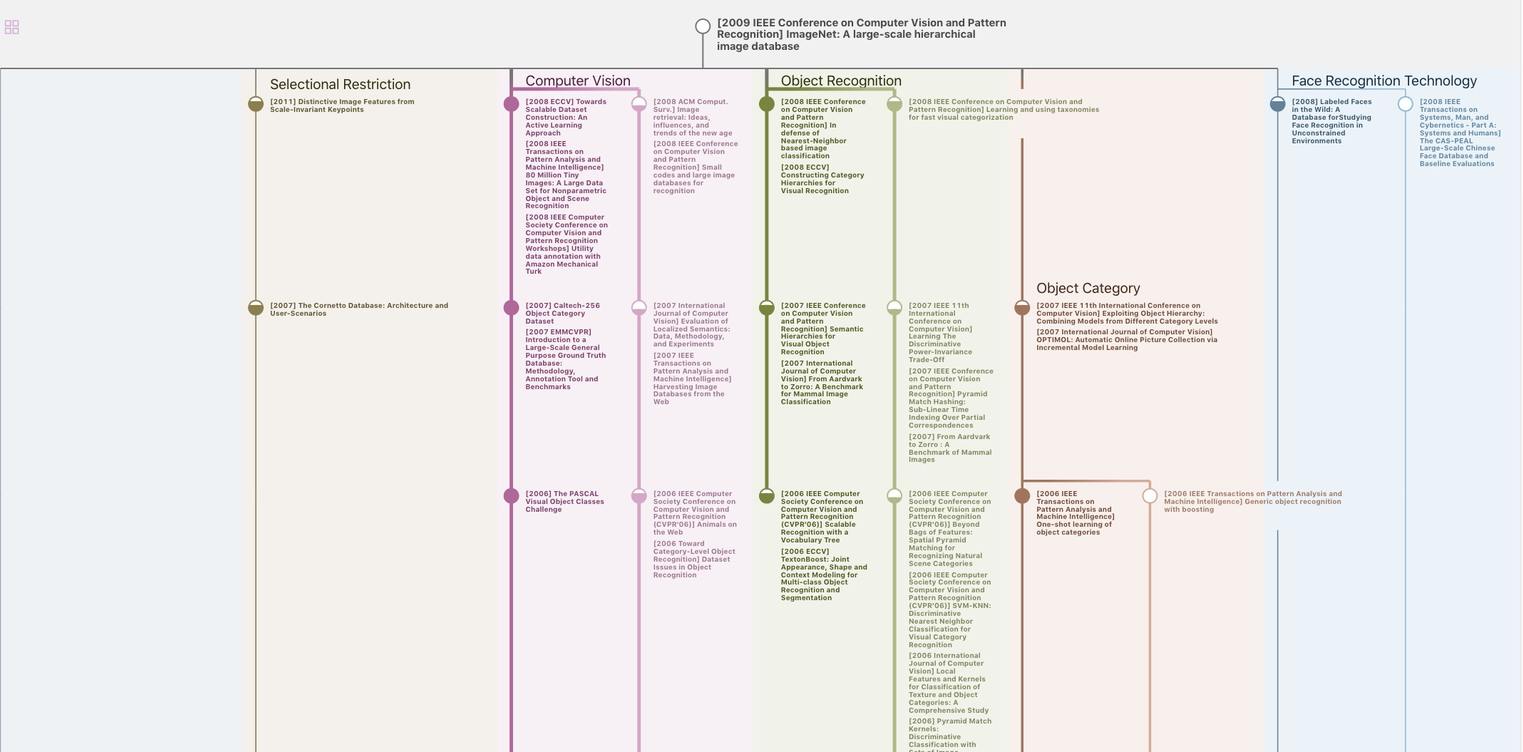
生成溯源树,研究论文发展脉络
Chat Paper
正在生成论文摘要