Independent Component Analysis Based on Mutual Dependence Measures.
ICMLA(2019)
摘要
We apply both distance-based and kernel-based mutual dependence measures to independent component analysis (ICA), and generalize dCovICA to MDMICA, minimizing empirical dependence measures as an objective function in both deflation and parallel manners. Solving this minimization problem, we introduce Latin hypercube sampling (LHS), and a global optimization method, Bayesian optimization (BO) to improve the initialization of the Newton-type local optimization method. The performance of MDMICA is evaluated in various simulation studies and an image data example. When the ICA model is correct, MDMICA achieves competitive results compared to existing approaches. When the ICA model is misspecified, the estimated independent components are less mutually dependent than the observed components using MDMICA, while the estimated independent components are prone to be even more mutually dependent than the observed components using other approaches.
更多查看译文
关键词
independent component analysis,mutual dependence measures,multivariate analysis,random sampling,global optimization
AI 理解论文
溯源树
样例
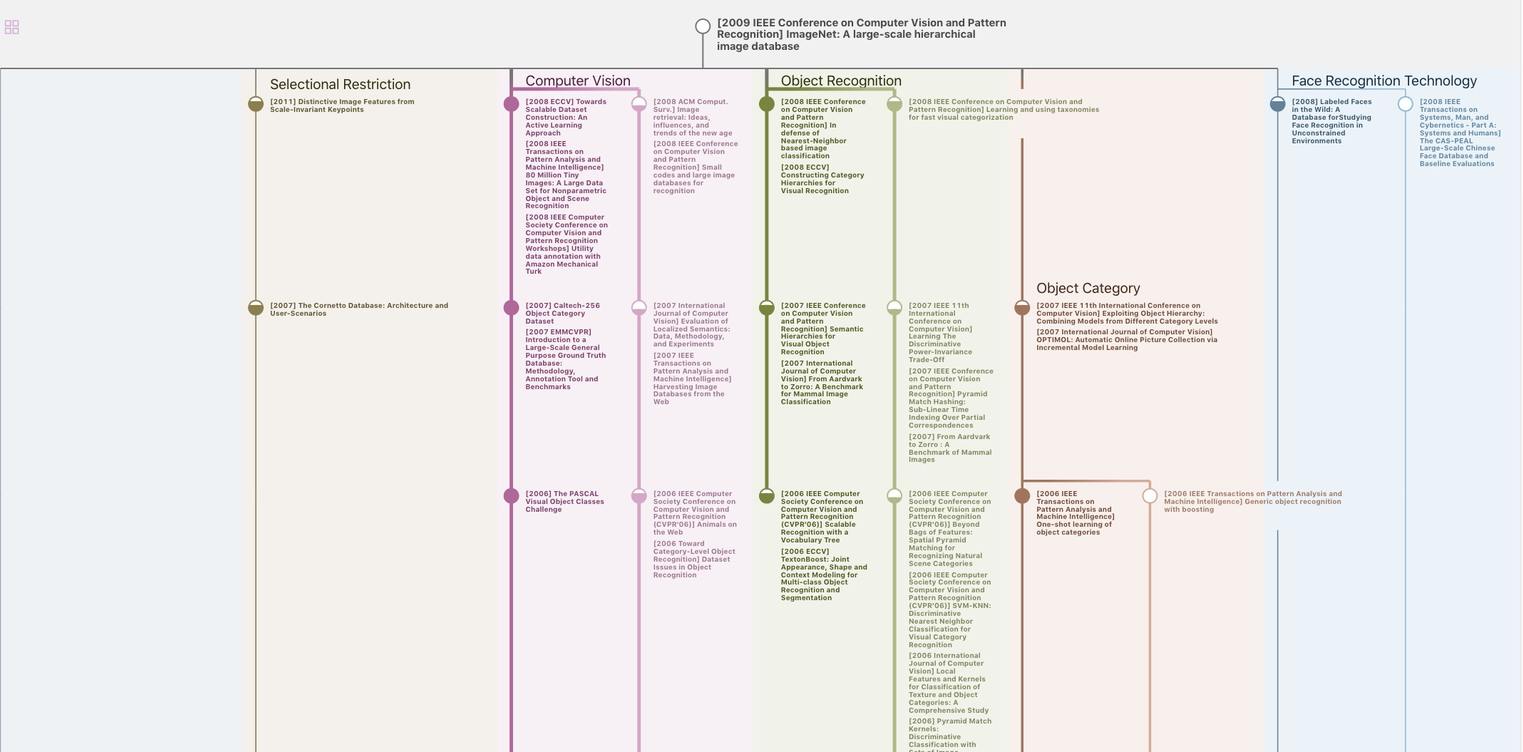
生成溯源树,研究论文发展脉络
Chat Paper
正在生成论文摘要