A Machine Learning Approach to Maximizing Broadband Capacity via Dynamic DOCSIS 3.1 Profile Management
2019 18th IEEE International Conference On Machine Learning And Applications (ICMLA)(2019)
摘要
A majority of American households with broadband today subscribe to a cable broadband service. The technology underpinning this cable broadband service is DOCSIS (Data-Over-Cable Service Interface Specifications). The latest version of this technology, DOCSIS 3.1, enables higher modem speeds compared to the previous DOCSIS 3.0 technology. DOCSIS 3.1 provides faster speeds by increasing the modulation order, and by using Orthogonal Frequency-Division Multiplexing (OFDM) to enable the use of such higher modulation orders. DOCSIS 3.1 allows different modems to use different modulations on different parts of the spectrum. Due to the complexity of selecting and configuring these varying modulation levels across the spectrum, most operators use the same modulation level across the entire spectrum. They configure multiple such profiles on a channel, so that different modems can use the most appropriate profile for them. This enable modems on a cleaner part of the plant to run higher modulations. However, the problem with such an approach is that noise in one part of the spectrum might reduce the entire channel's modulation to limit bit errors. A more sophisticated approach is to use profiles that dynamically vary the modulation on different sub-carriers to best match the noise conditions experienced in the plant. We leverage a Machine Learning based dynamic profile management approach and tested it on data from a top 10 US Cable Company and we observe more than 4% broadband bandwidth gains on average, with some consumers in noisy plant conditions seeing over 30% increase in their bandwidth speeds. And the gains are far more dramatic when compared with using lower modulation profiles (similar to DOCSIS 3.0), with average gain itself being over 37%.
更多查看译文
关键词
DOCSIS 3.1,OFDM,clustering,K-Means,Agglomerative clustering
AI 理解论文
溯源树
样例
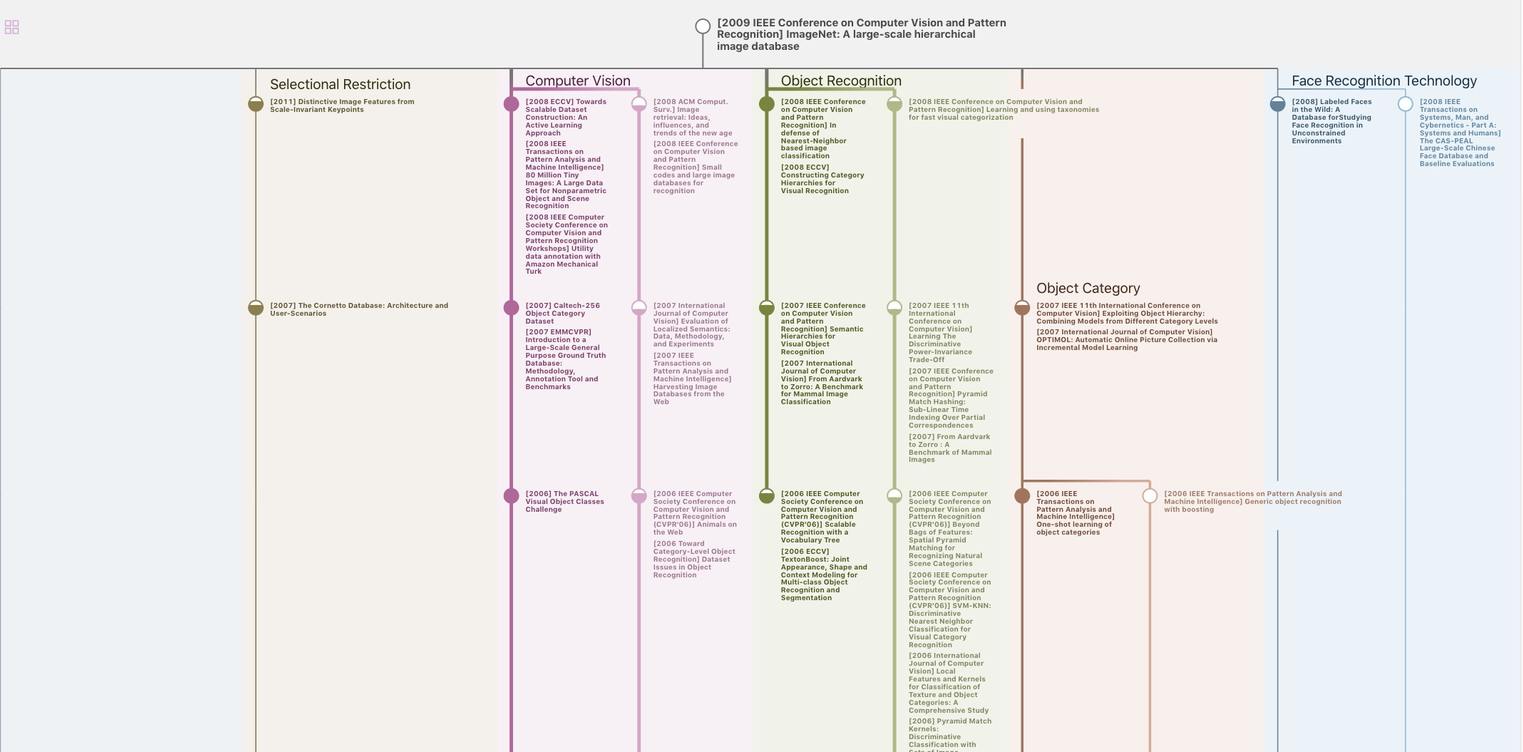
生成溯源树,研究论文发展脉络
Chat Paper
正在生成论文摘要