Towards Fast and Unified Transfer Learning Architectures for Sequence Labeling.
ICMLA(2019)
摘要
Sequence labeling systems have advanced continuously using neural architectures over the past several years. However, these tasks require large sets of annotated data to achieve such performance. In particular, we focus on the Named Entity Recognition (NER) task on clinical notes, which is one of the most fundamental and critical problems for medical text analysis. Our work centers on effectively adapting these neural architectures towards low-resource settings using parameter transfer methods. We complement a standard hierarchical NER model with a general transfer learning framework, the Tunable Transfer Network (TTN) consisting of parameter sharing between the source and target tasks, and showcase scores significantly above the baseline architecture. Our best TTN model achieves 2-5% improvement over pre-trained language model BERT as well as its multi task extension MT-DNN in low resource settings. However, our proposed sharing scheme requires an exponential search over tied parameter sets to generate an optimal configuration. To mitigate the problem of exhaustively searching for model optimization, we propose the Dynamic Transfer Networks (DTN), a gated architecture which learns the appropriate parameter sharing scheme between source and target datasets. DTN achieves the improvements of the optimized transfer learning framework with just a single training setting, effectively removing the need for an exponential search.
更多查看译文
关键词
transfer learning,named entity recognition,comprehend medical
AI 理解论文
溯源树
样例
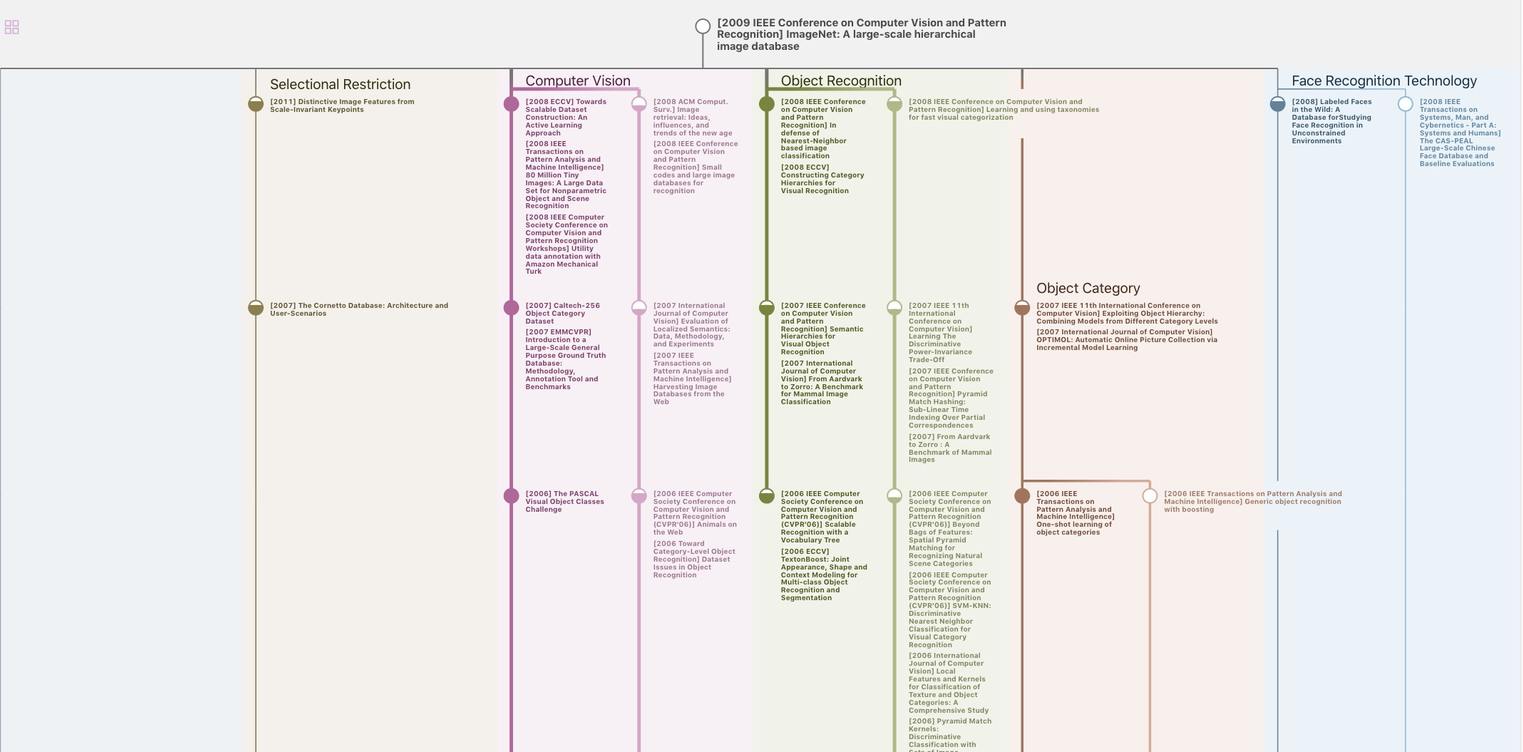
生成溯源树,研究论文发展脉络
Chat Paper
正在生成论文摘要