Hierarchical Symbol-Based Health-Status Analysis Using Time-Series Data in a Core Router System
IEEE Transactions on Computer-Aided Design of Integrated Circuits and Systems(2020)
摘要
To ensure high reliability and rapid error recovery in commercial core router systems, a health-status analyzer is essential to monitor the different features of core routers. However, traditional health analyzers need to store a large amount of historical data in order to identify health status. The storage requirement becomes prohibitively high when we attempt to carry out long-term health-status analysis for a large number of core routers. We describe the design of a symbol-based health status analyzer that first encodes, as a symbol sequence, the long-term complex time series collected from a number of core routers, and then utilizes the symbol sequence to do health analysis. The symbolic aggregation approximation (SAX), 1d-SAX, moving-average-based trend approximation, and nonparametric symbolic approximation representation methods are implemented to encode complex time series in a hierarchical way. Hierarchical agglomerative clustering and sequitur rule discovery are implemented to learn important global and local patterns. Three classification methods including a vector-space-model-based approach are then utilized to identify the health status of core routers. Data collected from a set of commercial core router systems are used to validate the proposed health-status analyzer. The experimental results show that our symbol-based health status analyzer requires much lower storage than traditional methods, but can still maintain comparable diagnosis accuracy.
更多查看译文
关键词
Time series analysis,Feature extraction,Monitoring,Fault tolerance,Fault tolerant systems,Detectors,Hardware
AI 理解论文
溯源树
样例
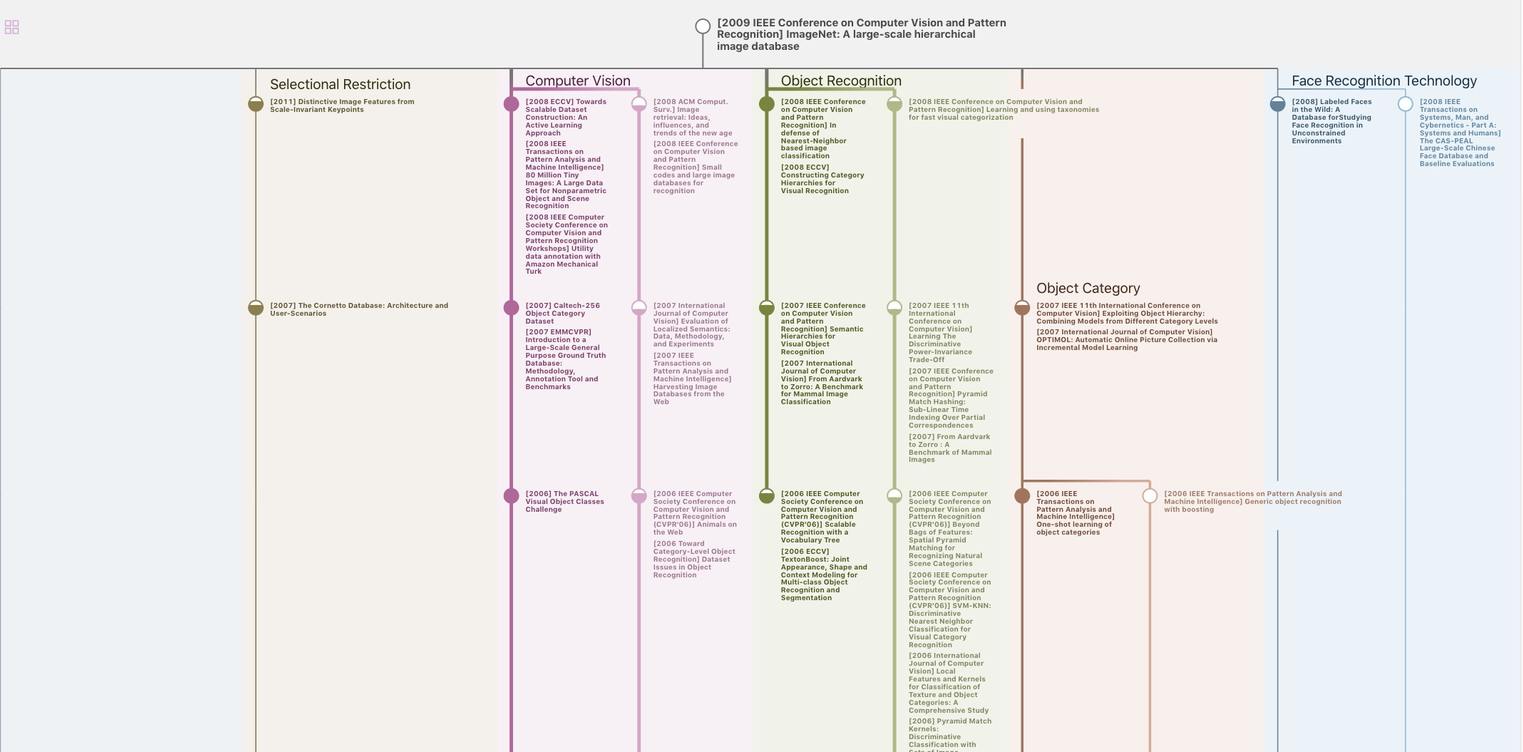
生成溯源树,研究论文发展脉络
Chat Paper
正在生成论文摘要