JRMOT: A Real-Time 3D Multi-Object Tracker and a New Large-Scale Dataset
IROS(2020)
摘要
An autonomous navigating agent needs to perceive and track the motion of objects and other agents in its surroundings to achieve robust and safe motion planning and execution. While autonomous navigation requires a multi-object tracking (MOT) system to provide 3D information, most research has been done in 2D MOT from RGB videos. In this work we present JRMOT, a novel 3D MOT system that integrates information from 2D RGB images and 3D point clouds into a real-time performing framework. Our system leverages advancements in neural-network based re-identification as well as 2D and 3D detection and descriptors. We incorporate this into a joint probabilistic data-association framework within a multi-modal recursive Kalman architecture to achieve online, real-time 3D MOT. As part of our work, we release the JRDB dataset, a novel large scale 2D+3D dataset and benchmark annotated with over 2 million boxes and 3500 time consistent 2D+3D trajectories across 54 indoor and outdoor scenes. The dataset contains over 60 minutes of data including 360 degree cylindrical RGB video and 3D pointclouds. The presented 3D MOT system demonstrates state-of-the-art performance against competing methods on the popular 2D tracking KITTI benchmark and serves as a competitive 3D tracking baseline for our dataset and benchmark.
更多查看译文
关键词
joint probabilistic data-association framework,multimodal recursive Kalman architecture,JRDB dataset,scale 2D+3D dataset,cylindrical RGB video,presented 3D MOT system,popular 2D tracking KITTI benchmark,3D tracking solution,social robot JackRabbot,multiobject tracker,large-scale dataset,robots navigating,robust trajectories,safe trajectories,3D Cartesian space,recent multiobject tracking research,tracking people,moving objects,2D RGB video sequences,novel 3D MOT system,RGB images,3D point clouds,state-of-the-art tracking performance,recent neural networks,track description,time 60.0 min
AI 理解论文
溯源树
样例
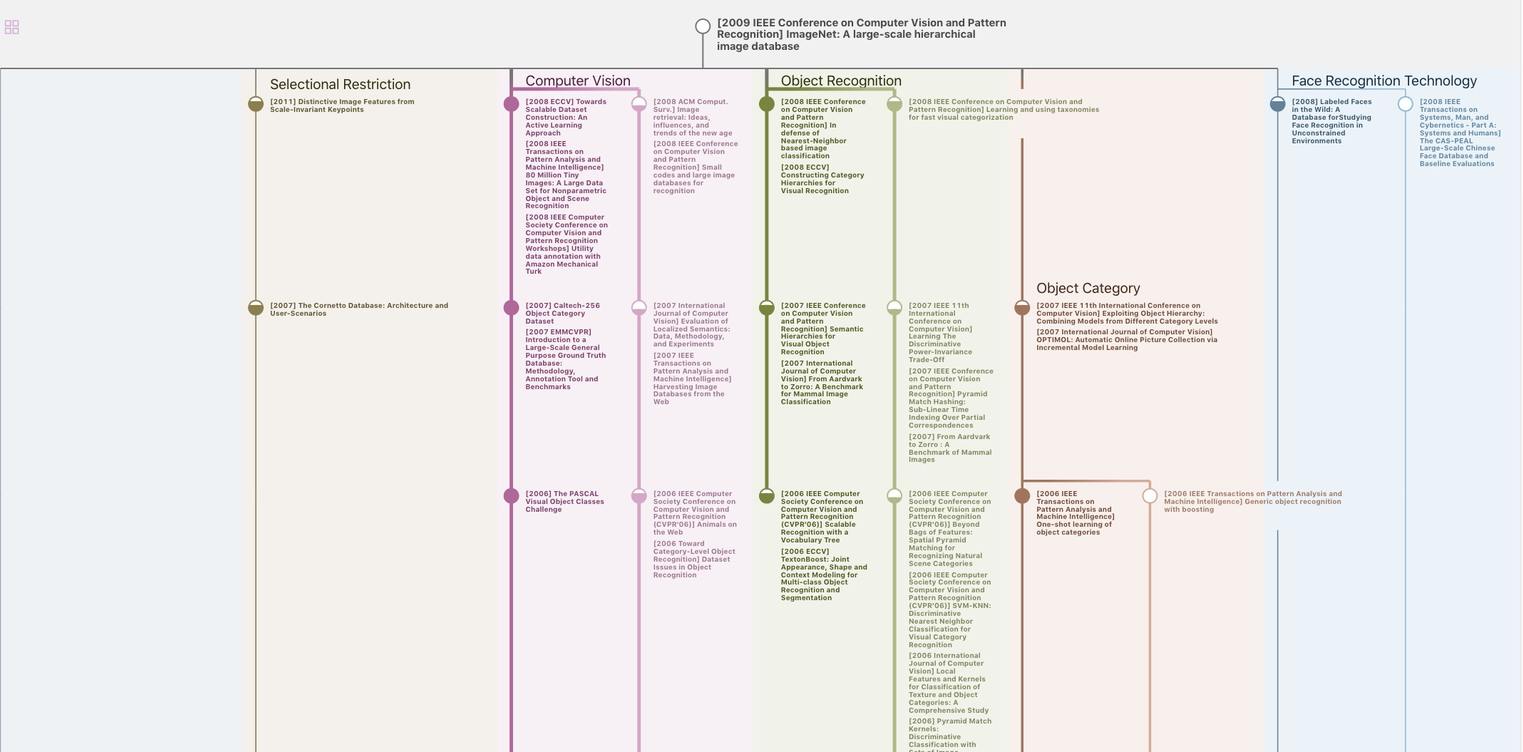
生成溯源树,研究论文发展脉络
Chat Paper
正在生成论文摘要