CNN-based approach for glaucoma diagnosis using transfer learning and LBP-based data augmentation
arxiv(2020)
摘要
Glaucoma causes an irreversible damage to retinal nerve fibers which results in vision loss, if undetected in early stage. Therefore, diagnosis of glaucoma in its early stage may prevent further vision loss. In this paper, we propose a convolutional neural network (CNN) based approach for automated glaucoma diagnosis by employing retinal fundus images. This approach employs transfer learning technique and local binary pattern (LBP) based data augmentation. In the proposed approach, we employ Alexnet as a pre-trained CNN model which is used for transfer learning. Initially, the proposed approach divides the fundus image dataset into training and testing data. Further, the color fundus images in training and testing data are separated into red (R), green (G), and blue (B) channels. Additionally, the LBP-based data augmentation is performed on training data. Specifically, we compute LPBs for each of the channel. Finally, the augmented training data is used to train the CNN model via transfer learning. In testing stage, the R, G, and B channels of test image are fed to the trained CNN model which generates 3 decisions. We employ a decision level fusion technique to combine the decisions obtained from the trained CNN model. The experimental evaluation of the proposed approach on the public RIM-ONE fundus image database, achieves state-of-the-art performance for glaucoma diagnosis.
更多查看译文
关键词
glaucoma diagnosis,data augmentation,transfer learning,cnn-based,lbp-based
AI 理解论文
溯源树
样例
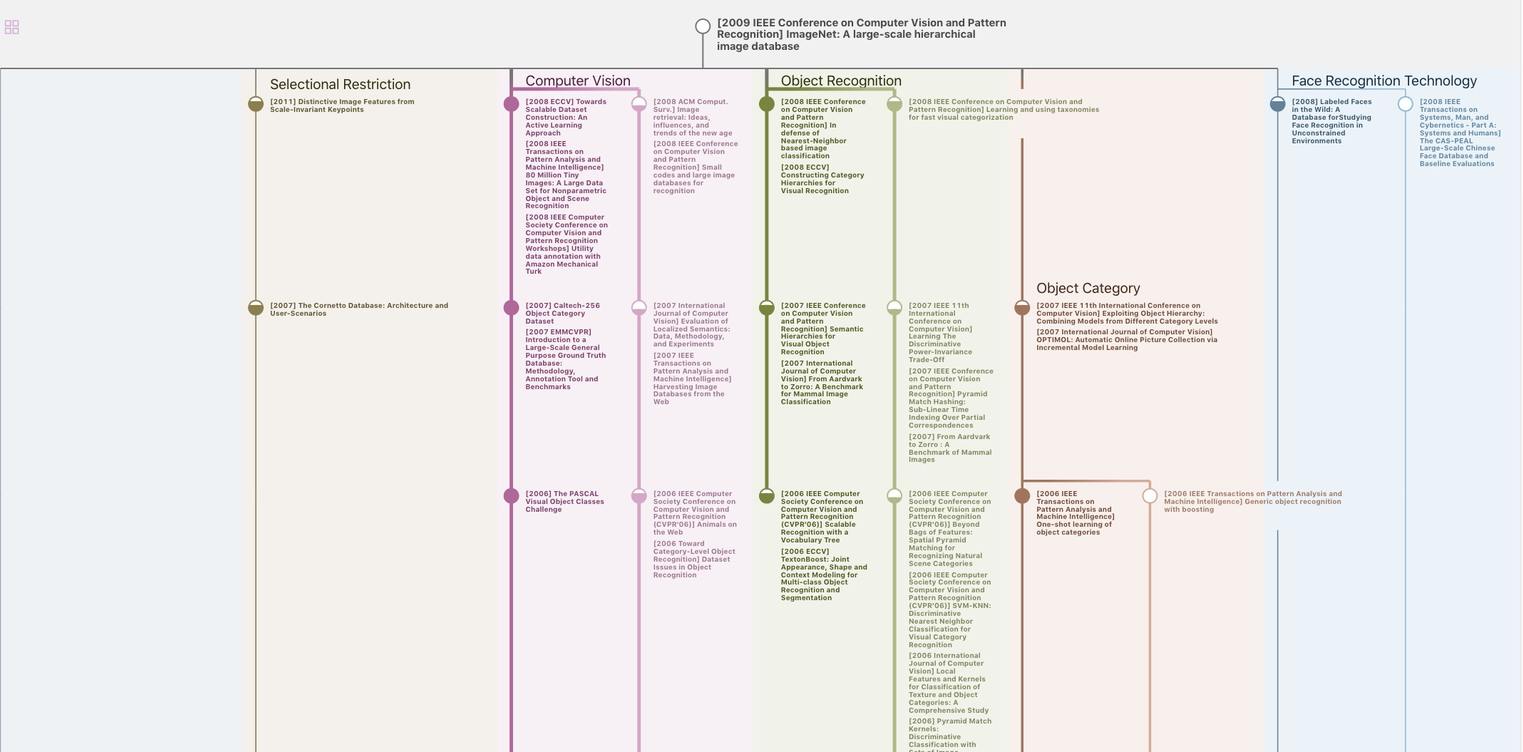
生成溯源树,研究论文发展脉络
Chat Paper
正在生成论文摘要