TIES: Temporal Interaction Embeddings For Enhancing Social Media Integrity At Facebook
KDD '20: The 26th ACM SIGKDD Conference on Knowledge Discovery and Data Mining Virtual Event CA USA July, 2020(2020)
摘要
Since its inception, Facebook has become an integral part of the online social community. People rely on Facebook to connect with others and build communities. As a result, it is paramount to protect the integrity of such a large network in a fast and scalable manner. In this paper, we present our efforts to protect various social media entities at Facebook from people who try to abuse our platform. We present a novel Temporal Interaction EmbeddingS (TIES) model that is designed to capture rogue social interactions and flag them for further suitable actions. TIES is a supervised, deep learning, production ready model at Facebook-scale networks. Prior works on integrity problems are mostly focused on capturing either only static or certain dynamic features of social entities. In contrast, TIES can capture both these variant behaviors in a unified model owing to the recent strides made in the domains of graph embedding and deep sequential pattern learning. To show the real-world impact of TIES, we present a few applications especially for preventing spread of misinformation, fake account detection, and reducing ads payment risks in order to enhance Facebook platform's integrity.
更多查看译文
关键词
temporal embeddings, sequence modeling, social media integrity
AI 理解论文
溯源树
样例
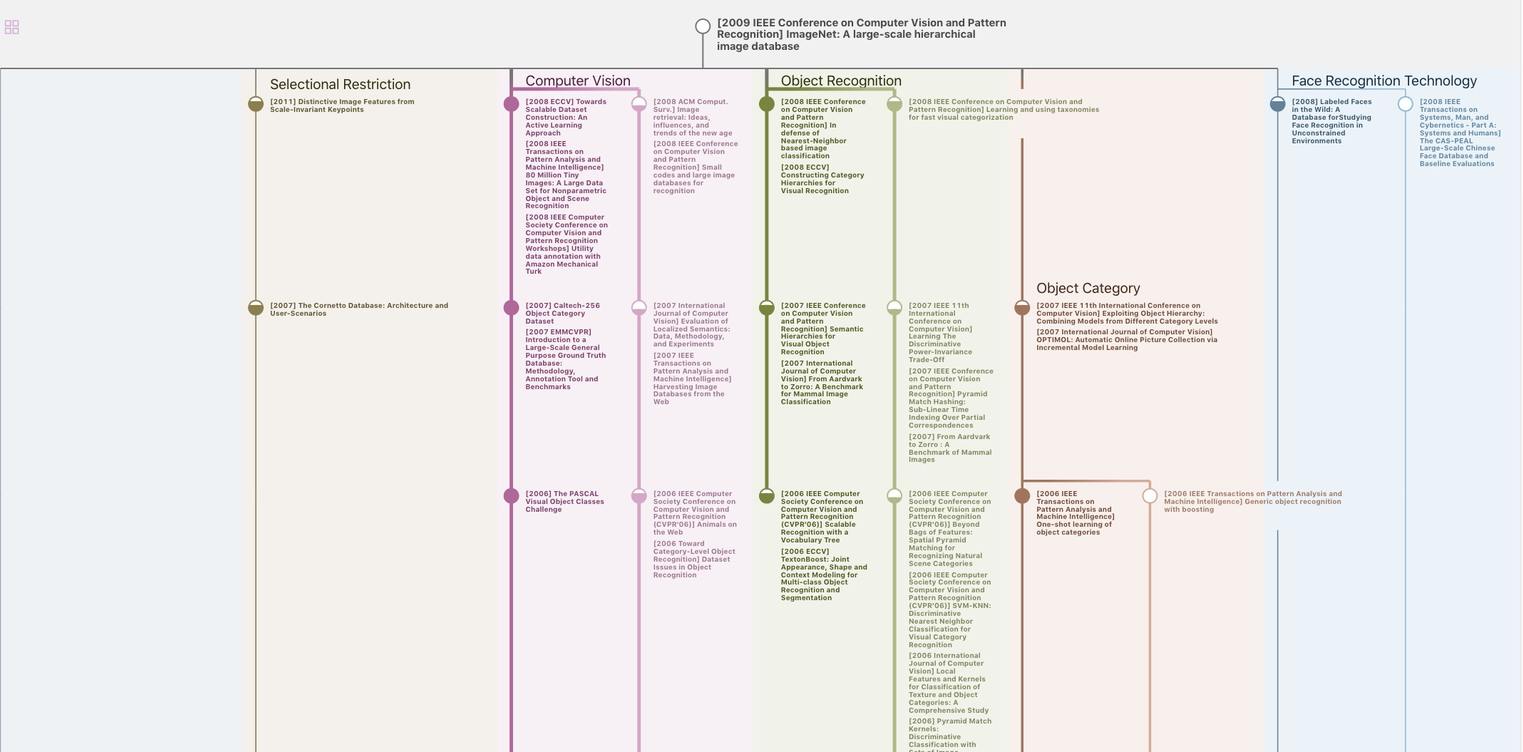
生成溯源树,研究论文发展脉络
Chat Paper
正在生成论文摘要