Improving Augmented Human Intelligence To Distinguish Burkitt Lymphoma From Diffuse Large B-Cell Lymphoma Cases
AMERICAN JOURNAL OF CLINICAL PATHOLOGY(2019)
摘要
Objectives: To assess and improve the assistive role of a deep, densely connected convolutional neural network (CNN) to hematopathologists in differentiating histologic images of Burkitt lymphoma (BL) from diffuse large B-cell lymphoma (DLBCL). Methods: A total of 10,818 images from BL (n = 34) and DLBCL (n = 36) cases were used to either train or apply different CNNs. Networks differed by number of training images and pixels of images, absence of color, pixel and staining augmentation, and depth of the network, among other parameters. Results: Cases classified correctly were 17 of 18 (94%), nine with 100% of images correct by the best performing network showing a receiver operating characteristic curve analysis area under the curve 0.92 for both DLBCL and BL. The best performing CNN used all available training images, two random subcrops per image of 448 x 448 pixels, random H&E staining image augmentation, random horizontal flipping of images, random alteration of contrast, reduction on validation error plateau of 15 epochs, block size of six, batch size of 32, and depth of 22. Other networks and decreasing training images had poorer performance. Conclusions: CNNs are promising augmented human intelligence tools for differentiating a subset of BL and DLBCL cases.
更多查看译文
关键词
Burkitt lymphoma,Diffuse large B-cell lymphoma,Convolutional neural network,Artificial intelligence,Augmented human intelligence,Augmented intelligence,Hematopathology
AI 理解论文
溯源树
样例
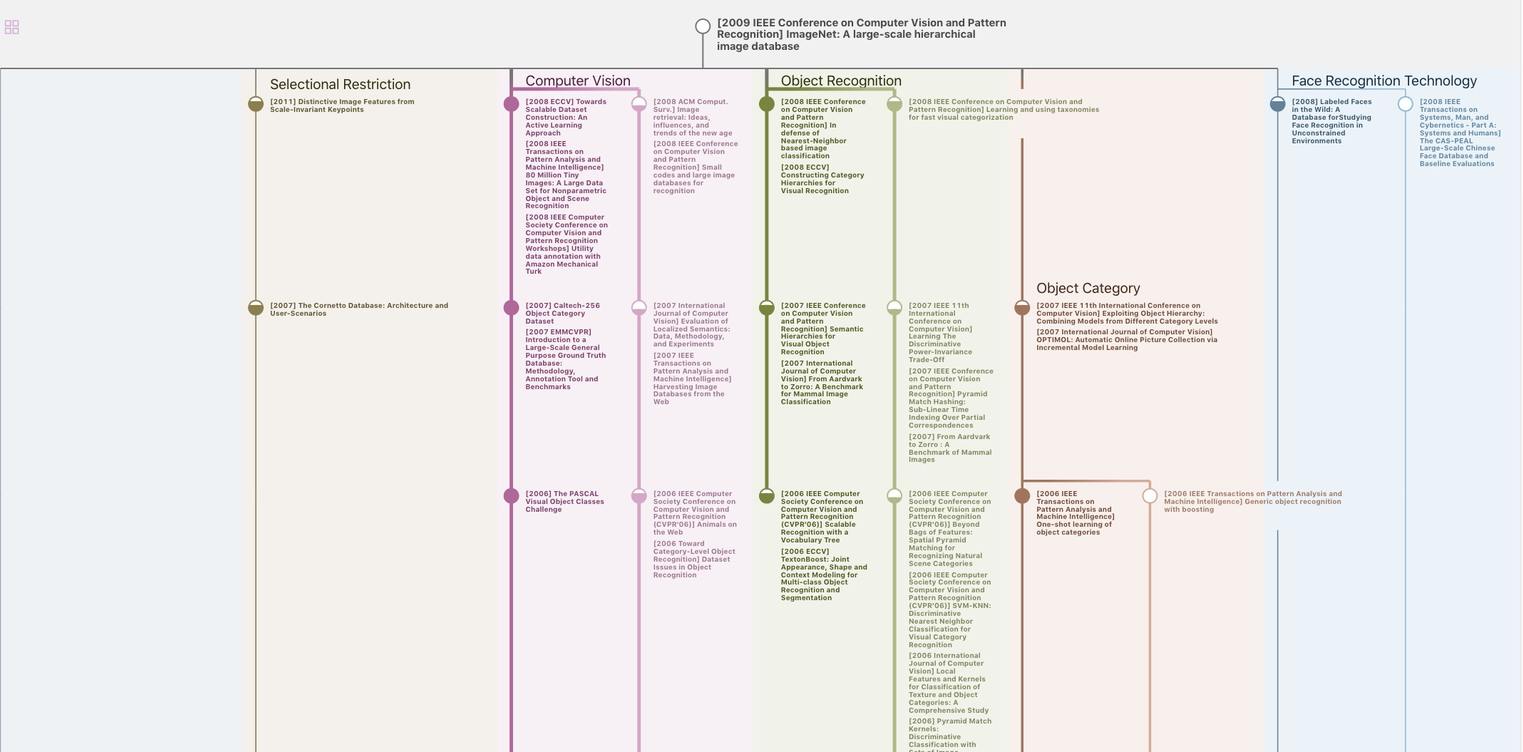
生成溯源树,研究论文发展脉络
Chat Paper
正在生成论文摘要