Hyper-parameter Analysis of the Improved Deep Embedding Clustering Model
2018 International Conference on Security, Pattern Analysis, and Cybernetics (SPAC)(2018)
摘要
Clustering has gained large successful in many areas in machine learning especially the computer vision and knowledge discovery. However, the clustering tasks on the high-dimension data is still the bottleneck of the clustering. And this problem mainly due to the lack of representative and important features of the data. Recently, the efficient deep clustering models including the deep embedded clustering model (DEC) and the improved deep embedded clustering model (IDEC) are proposed to solve this problem. They validated their models on the real-world datasets and improved the clustering performance compared with the traditional clustering algorithm, which have demonstrated that the superior of DEC and IDEC. Both the clustering method used in DEC and IDEC are student-t distribution, and we choose the IDEC model for further studying the robustness of these two models. Therefore, we discuss how sensitivity of hyper-parameter influence in the IDEC model, and comparative experiments are given to show the sensitivities of the hyper-meters in IDEC in term of the clustering performances.
更多查看译文
关键词
Deep Learning,Auto-encoder,student-t distribution,high-dimension real-world clustering
AI 理解论文
溯源树
样例
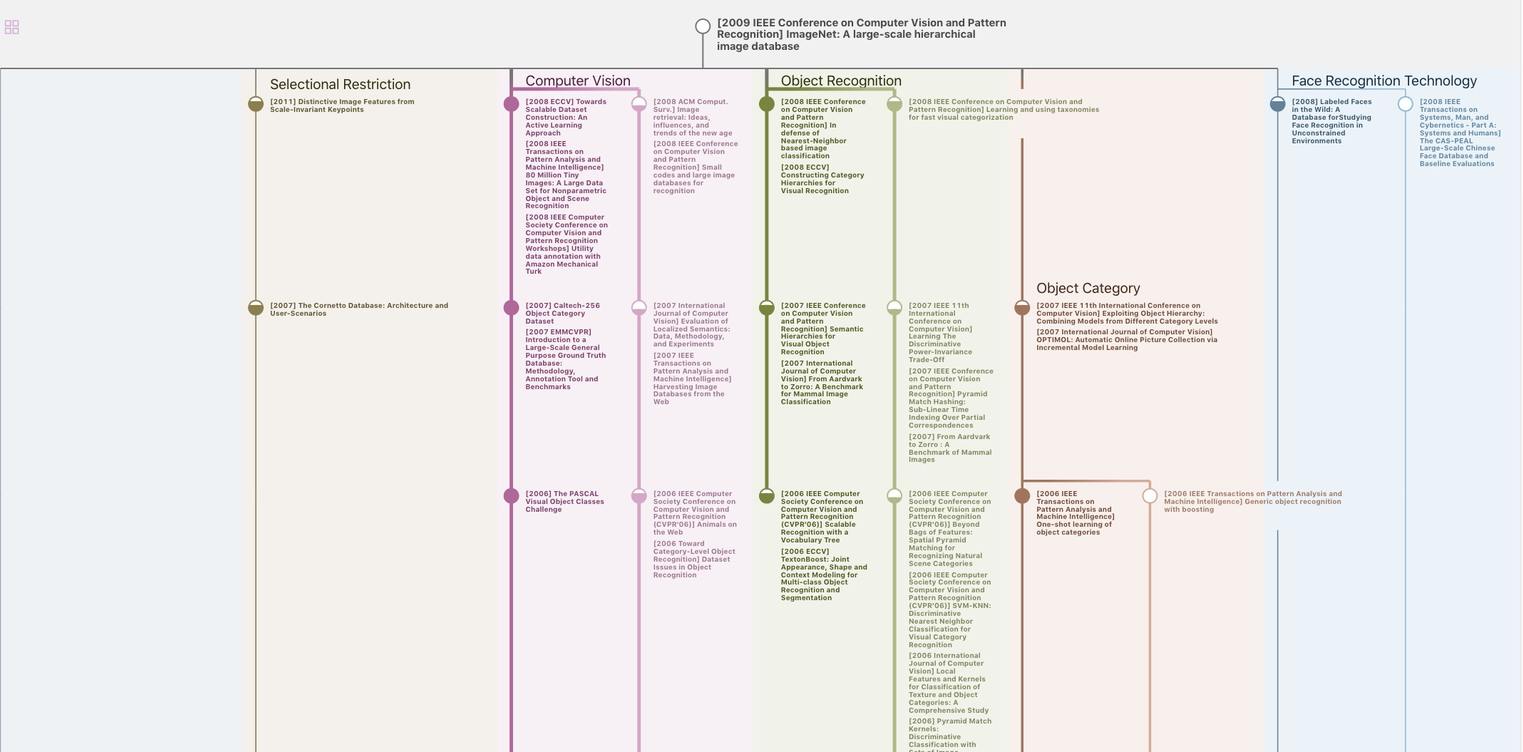
生成溯源树,研究论文发展脉络
Chat Paper
正在生成论文摘要