Improved Network Traffic Classification Using Ensemble Learning
2019 IEEE SYMPOSIUM ON COMPUTERS AND COMMUNICATIONS (ISCC)(2019)
摘要
Despite the large number of research efforts that applied specific machine learning algorithms for network traffic classification, recent work has highlighted limitations and particularities of individual algorithms that make them more suitable to specific types of traffic and scenarios. As such, an important topic in this area is how to combine individual algorithms using meta-learning techniques in order to obtain more robust traffic classification metrics. This paper presents a comparative analysis among meta-learning approaches and individual classifiers to classify network traffic. We investigate and evaluate a range of meta-learning techniques, including Voting, Stacking, Bagging and Boosting. We then propose a new experimental analysis of different meta-learning techniques - also known as ensemble learners- and compare them with their own base classifiers when used individually. Finally, considering the emerging popularity of Neural Networks, we analyze this scenario using the Multi-layer Perceptron classifier. The experiments were performed with data provided by the UCI Machine Learning Repository. The best performance was obtained by an ensemble technique (Bagging), which obtained accuracy of 99.972% and false positive rate of 0.00018%.
更多查看译文
关键词
ensemble learning,specific machine learning algorithms,robust traffic classification metrics,meta-learning techniques,base classifiers,Neural Networks,multilayer perceptron classifier,UCI Machine Learning Repository,ensemble technique,improved network traffic classification,voting technique,stacking techniques,bagging techniques,boosting techniques
AI 理解论文
溯源树
样例
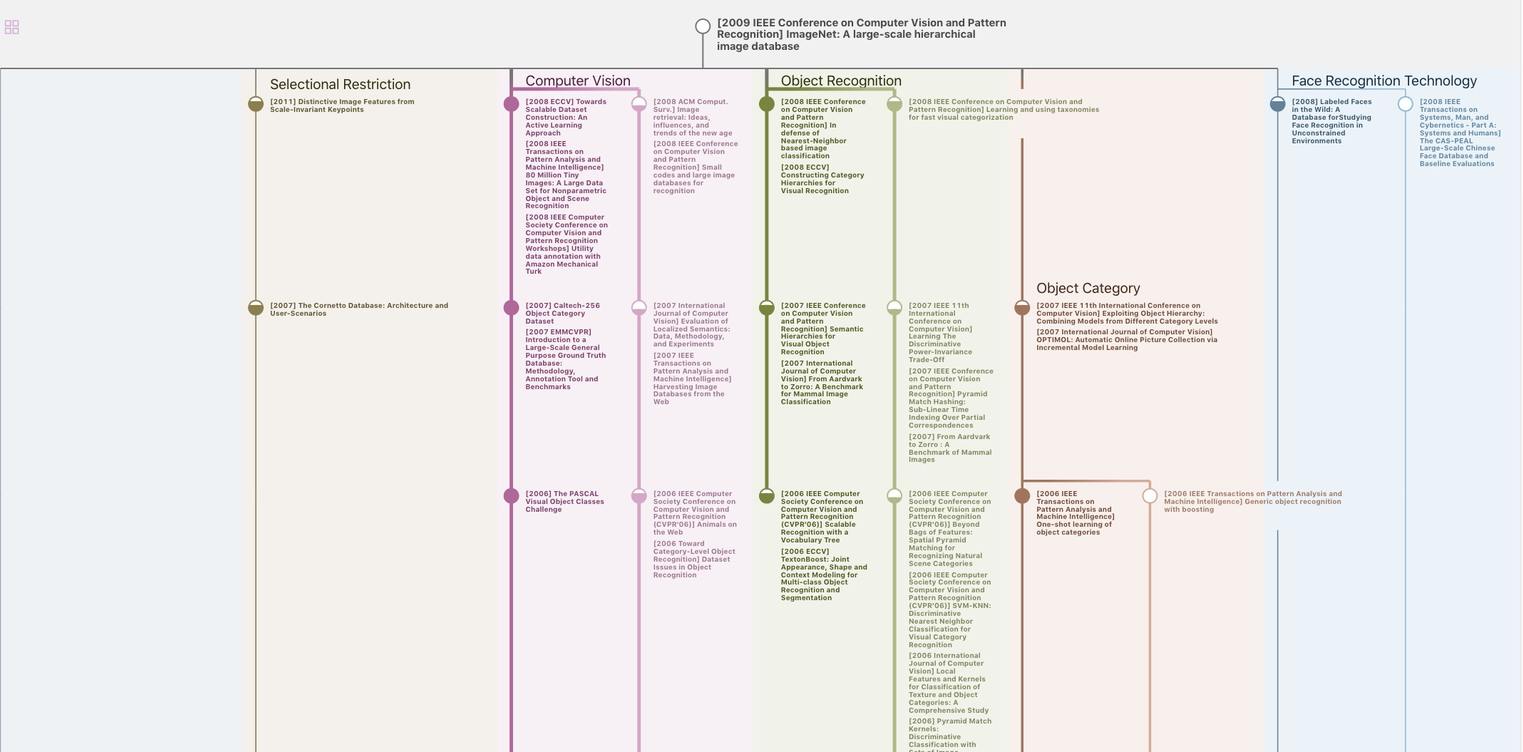
生成溯源树,研究论文发展脉络
Chat Paper
正在生成论文摘要