The Mechanism of a Multi-Branch Structure for EEG-Based Motor Imagery Classification
ROBIO(2019)
摘要
In the field of motor imagery (MI) classification, it is a great challenge to preserve temporal features and spatial ones of the electroencephalogram (EEG) data simultaneously for effective processing. In our former study, a novel framework for MI classification has been proposed, which represents the EEG data into a 3D form and handles it with a multi-branch 3D convolutional neural network (3D CNN). The 3D CNN is constructed with three different branches and already proved to be better than one branch network. In this paper, to study the mechanism of the multi-branch structure, we modify the framework of the multi-branch network with three same networks as the branch and compare different multi-branch networks with the corresponding one branch network to find whether multi-branch structure is better than one branch structure in MI classification tasks. Experiment results revealed that the modified multi-branch 3D CNN can also reach state-of-the-art classification kappa value level and performs better than other methods in terms of standard deviation including the original network with three different branches. The good performance of the modified network may reflect multi-branch structure is better than one branch structure and shows its application value in EEG classification.
更多查看译文
关键词
motor imagery (MI),electroencephalogram (EEG),3D convolutional neural network (3D CNN),multi-branch structure,one branch structure
AI 理解论文
溯源树
样例
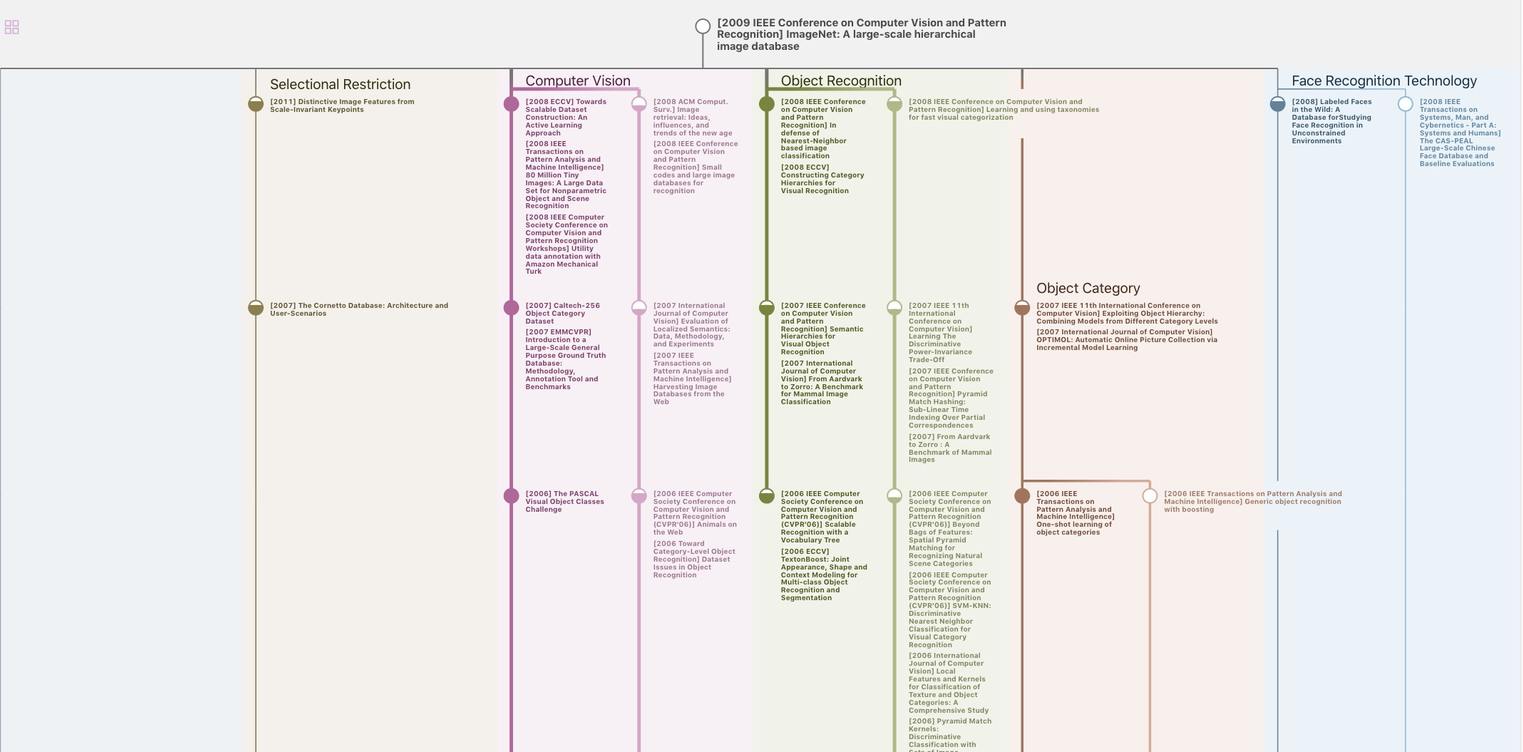
生成溯源树,研究论文发展脉络
Chat Paper
正在生成论文摘要