Non-Parametric Mixed-Manifold Products Using Multiscale Kernel Densities
2019 IEEE/RSJ INTERNATIONAL CONFERENCE ON INTELLIGENT ROBOTS AND SYSTEMS (IROS)(2019)
摘要
We extend the core operation of non-parametric belief propagation (NBP), also known as multi-scale sequential Gibbs sampling, to approximate products of kernel density estimated beliefs that reside on some manifold. The original algorithm, though multidimensional, implicitly assumes the beliefs to reside on the Euclidean R-d. space only. The proposed extension generalizes to any mixture of Riemannian manifolds, provided the primary operations-addition and subtraction-are defined. Our motivation is primarily focused on state-estimation using non-Gaussian factor graphs for multimodal simultaneous localization and mapping in robotics. The paper presents the method as well as simulation and experimental results for validation. Our implementation is publicly available and allows for expansion with user-defined manifold mixtures.
更多查看译文
关键词
nonparametric mixed-manifold products,nonparametric belief propagation,multiscale sequential Gibbs sampling,kernel density estimated beliefs,Euclidean,Riemannian manifolds,state-estimation,nonGaussian factor graphs,user-defined manifold mixtures,multiscale kernel densities,NBP,multimodal simultaneous localization and mapping
AI 理解论文
溯源树
样例
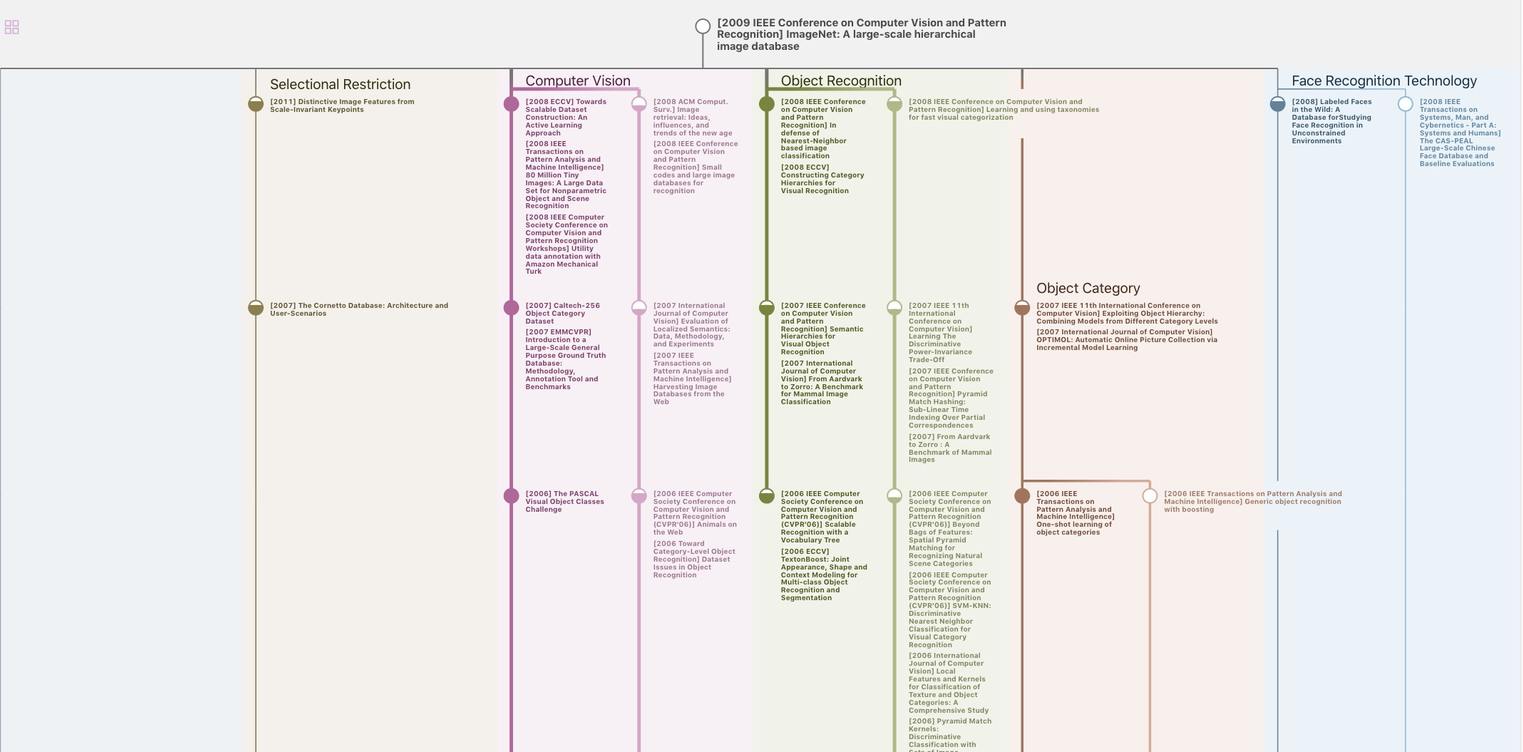
生成溯源树,研究论文发展脉络
Chat Paper
正在生成论文摘要