Learning Robust and Multilingual Speech Representations
EMNLP(2020)
摘要
Unsupervised speech representation learning has shown remarkable success at finding representations that correlate with phonetic structures and improve downstream speech recognition performance. However, most research has been focused on evaluating the representations in terms of their ability to improve the performance of speech recognition systems on read English (e.g. Wall Street Journal and LibriSpeech). This evaluation methodology overlooks two important desiderata that speech representations should have: robustness to domain shifts and transferability to other languages. In this paper we learn representations from up to 8000 hours of diverse and noisy speech data and evaluate the representations by looking at their robustness to domain shifts and their ability to improve recognition performance in many languages. We find that our representations confer significant robustness advantages to the resulting recognition systems: we see significant improvements in out-of-domain transfer relative to baseline feature sets and the features likewise provide improvements in 25 phonetically diverse languages including tonal languages and low-resource languages.
更多查看译文
关键词
robust,speech,learning
AI 理解论文
溯源树
样例
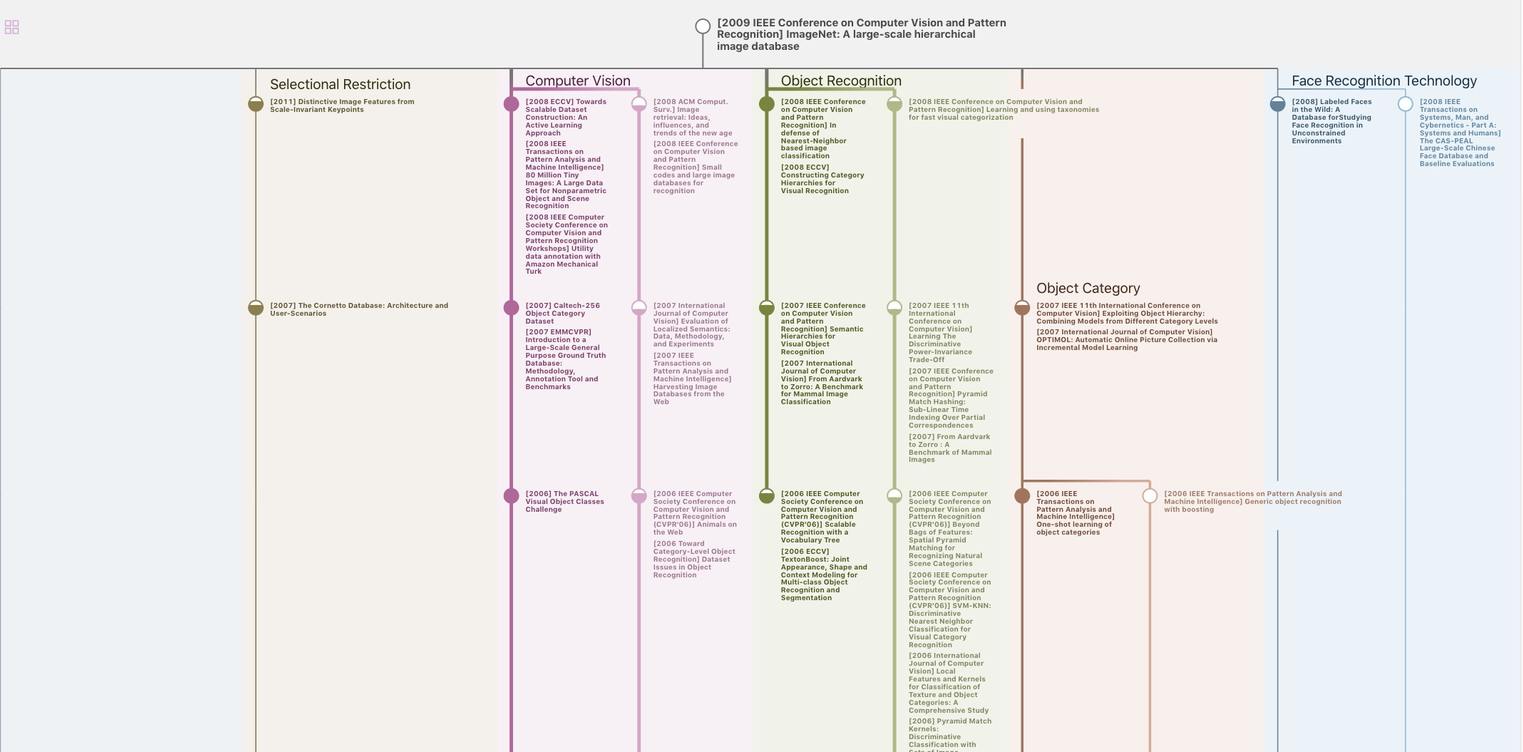
生成溯源树,研究论文发展脉络
Chat Paper
正在生成论文摘要