ScleraSegNet: An Attention Assisted U-Net Model for Accurate Sclera Segmentation
IEEE Transactions on Biometrics, Behavior, and Identity Science(2020)
摘要
This paper proposes a novel sclera segmentation approach based on an attention assisted U-Net model, named ScleraSegNet. Several off-the-shelf or improved attention modules are incorporated into the central bottleneck part or skip connection part of the original U-Net, helping the new model implicitly learn to suppress irrelevant regions in an input image while highlighting salient features useful for a specific task. This enables us to eliminate the necessity of using an external ROI localization model of cascade frameworks. The proposed approach is evaluated on several public, challenging eye datasets and experimental results show that introduced attention modules consistently improve the segmentation performance over the original U-Net across different datasets, and the best performing ScleraSegNet (CBAM) model achieves state-of-the-art segmentation performance. By utilizing the high stage’s semantic information to guide the selection of features from the low stage in channel-wise and spatial-wise, the further improved ScleraSegNet (SSBC) model ranked first in the Sclera Segmentation Benchmarking Competition 2019 (SSBC 2019), part of ICB 2019, with a Precision value of 92.88% and a Recall value of 90.34% on the MASD.v1 dataset, and a Precision value of 83.19% and a Recall value of 80.01% on the MSD dataset, respectively.
更多查看译文
关键词
Sclera segmentation,sclera recognition,U-net,attention mechanism,SSBC
AI 理解论文
溯源树
样例
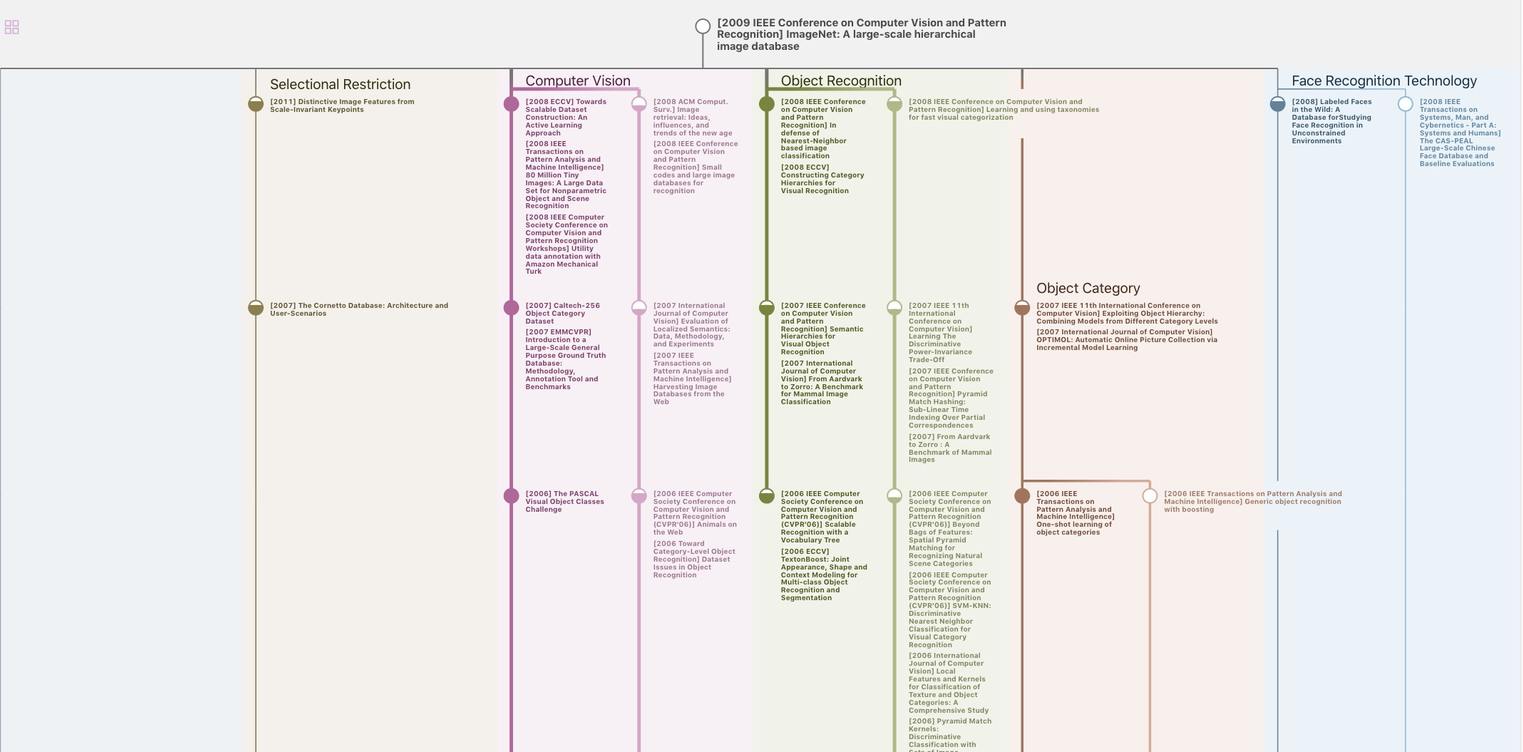
生成溯源树,研究论文发展脉络
Chat Paper
正在生成论文摘要