LARA - Attribute-to-feature Adversarial Learning for New-item Recommendation.
WSDM '20: The Thirteenth ACM International Conference on Web Search and Data Mining Houston TX USA February, 2020(2020)
摘要
Recommending new items in real-world e-commerce portals is a challenging problem as the cold start phenomenon, i.e., lacks of user-item interactions. To address this problem, we propose a novel recommendation model, i.e., adversarial neural network with multiple generators, to generate users from multiple perspectives of items' attributes. Namely, the generated users are represented by attribute-level features. As both users and items are attribute-level representations, we can implicitly obtain user-item attribute-level interaction information. In light of this, the new item can be recommended to users based on attribute-level similarity. Extensive experimental results on two item cold-start scenarios, movie and goods recommendation, verify the effectiveness of our proposed model as compared to state-of-the-art baselines.
更多查看译文
关键词
Recommender System, Cold-start, Generative Adversarial Networks
AI 理解论文
溯源树
样例
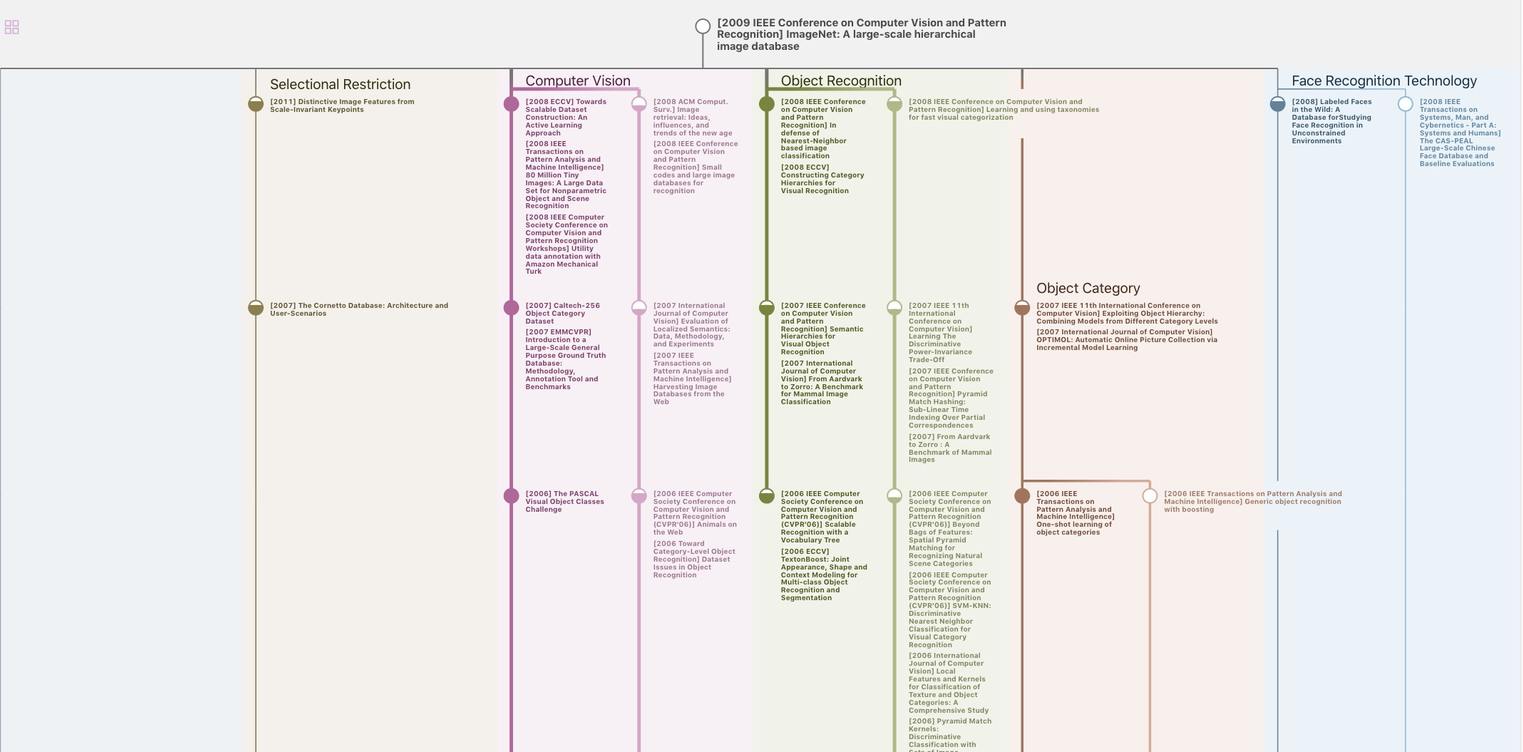
生成溯源树,研究论文发展脉络
Chat Paper
正在生成论文摘要