Adversarial Training Methods For Boltzmann Machines
IEEE ACCESS(2020)
摘要
A Restricted Boltzmann Machines (RBM) is a generative Neural Net that is typically trained to minimize KL divergence between data distribution P-data and its model distribution P-RBM. However, minimizing this KL divergence does not sufficiently penalize an RBM that place a high probability in regions where the data distribution has a low density, and therefore, RBMs always generate blurry images. In order to solve this problem, this paper extends the loss function of RBMs from KL divergence to adversarial loss and proposes an Adversarial Restricted Boltzmann Machine (ARBM) and an Adversarial Deep Boltzmann Machine (ADBM). Different from the other RBMs, an ARBM minimizes its adversarial loss between the data distribution and its model distribution without explicit gradients. Different from traditional DBMs, an ADBM minimizes its adversarial loss without a layer-by-layer pre-training. In order to generate high-quality color images, this paper proposes an Adversarial Hybrid Deep Generative Net (AHDGN) based on an ADBM. The experiments verify that the adversarial loss can be minimized in our proposed models, and the generated images are comparable with the current state-of-the-art results.
更多查看译文
关键词
Restricted Boltzmann machine, generative model, adversarial generative net, Gibbs sampling, neural net
AI 理解论文
溯源树
样例
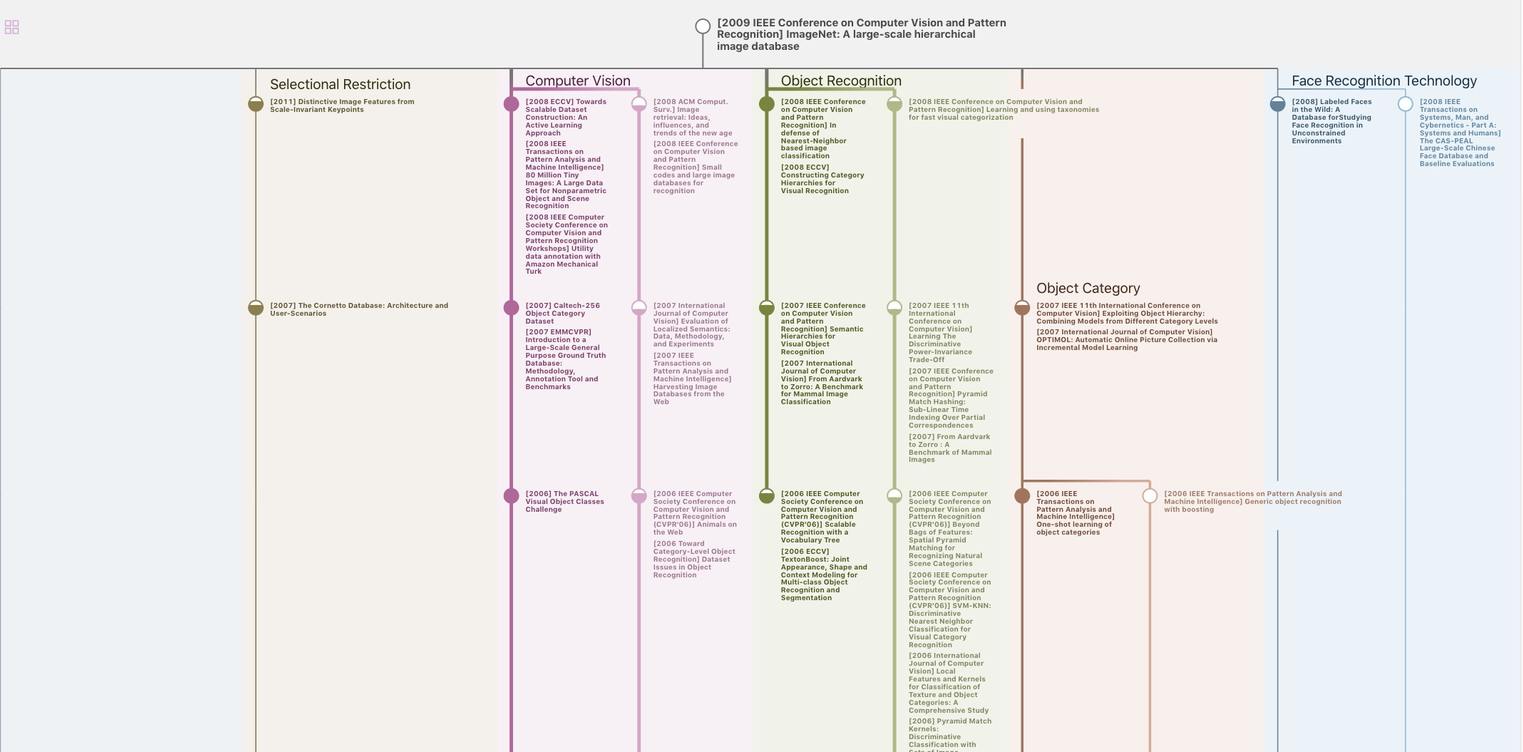
生成溯源树,研究论文发展脉络
Chat Paper
正在生成论文摘要